Research on neural processes with multiple latent variables
IET IMAGE PROCESSING(2023)
摘要
Neural Process (NP) fully combines the advantages of neural network and Gaussian Process (GP) to provide an efficient method for solving regression problems. Nonetheless, limited by the dimensionality of the latent variable, NP has difficulty fitting the observed data completely and predicting the targets perfectly. To remedy these drawbacks, the authors propose a concise and effective improvement of the latent path of NP, which the authors term Multi-Latent Variables Neural Process (MLNP). MLNP samples multiple latent variables and integrates the representations corresponding to the latent variables in the decoder with adaptive weights. MLNP inherits the desirable property of linear computation scales of NP and learns the approximate distribution over objective functions from contexts more flexibly and accurately. By applying MLNP to 1-D regression, real-world image completion, which can be seen as a 2-D regression task, the authors demonstrate its significant improvement in the accuracy of prediction and contexts fitting capability compared with NP. Through ablation experiments, the authors also verify that the number of latent variables has a great impact on the prediction accuracy and fitting capability of MLNP. Moreover, the authors also analyze the roles played by different latent variables in reconstructing images.
更多查看译文
关键词
encoder–decoder,multiple latent variables,neural process,regression
AI 理解论文
溯源树
样例
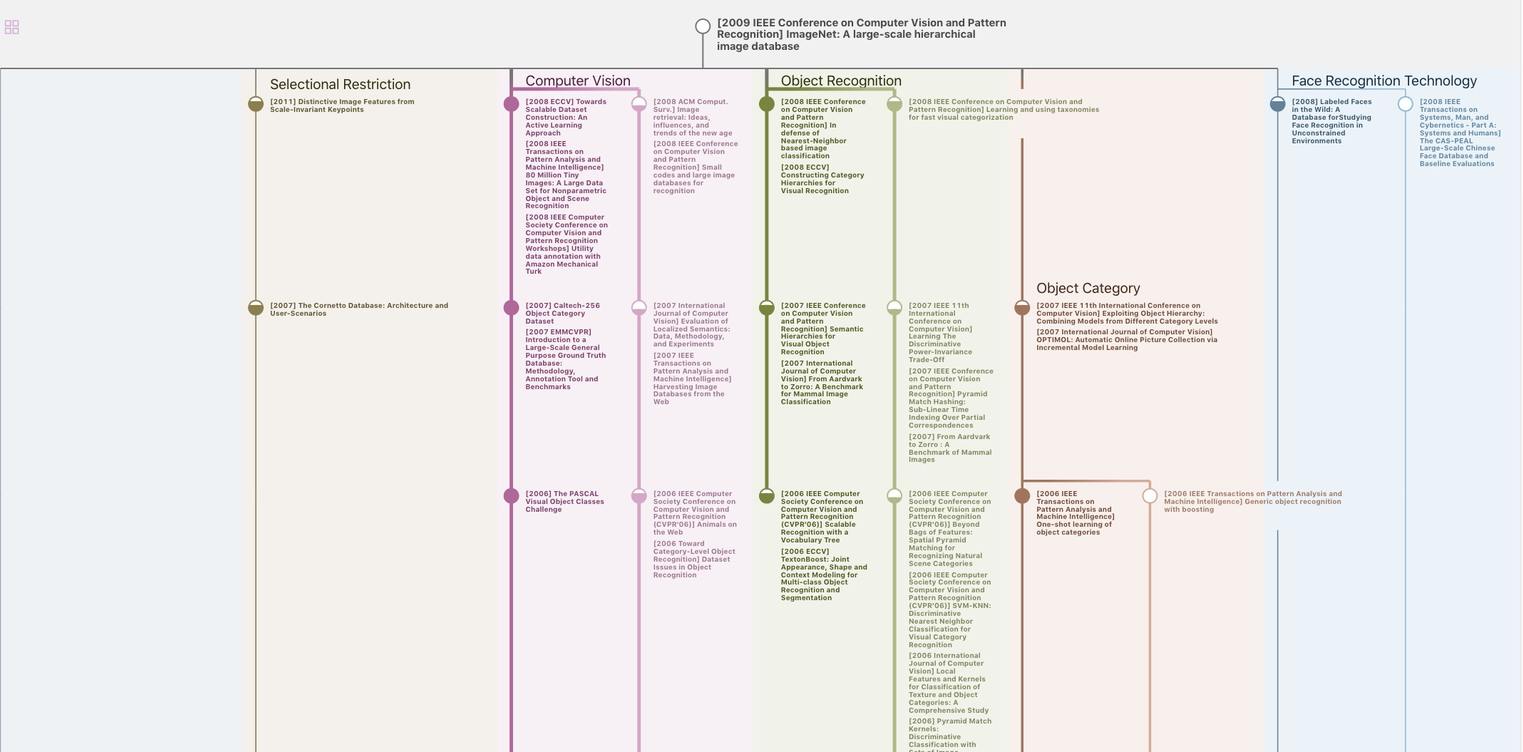
生成溯源树,研究论文发展脉络
Chat Paper
正在生成论文摘要