Multi factors-PredRNN based significant wave height prediction in the Bohai, Yellow, and East China Seas
FRONTIERS IN MARINE SCIENCE(2023)
摘要
IntroductionCurrently, deep-learning-based prediction of Significant Wave Height (SWH) is mostly performed for a single location in the ocean or simply relies on a single factor (SF). Such approaches have the disadvantage of lacking spatial correlations or dynamic complexity, leading to an inevitable growth of the prediction error with time. MethodsHere, attempting a solution, we develop a Multi-Factor (MF) data-driven 2D SWH prediction model for the Bohai, Yellow, and East China Seas (BYECS). Our model is developed based on a multi-channel PredRNN algorithm that is an improved deep-learning calculation of the ConvLSTM. ResultsIn our model, the MF of historical SWH, 10 m surface winds, ocean surface currents, bathymetries, and open boundaries are used to predict 2D SWH in the next 1-72h. Our modeled SWHs show the correlation coefficients as 0.98, 0.90, and 0.87 for the next 6h, 24h, and 72h, respectively. DiscussionAccording to the ablation experiments, winds are the dominant factor in the MF model and the memory-decoupling module is the key improvement of the PredRNN compared to the ConvLSTM. Furthermore, when the historical SWH is excluded from the input, the correlation coefficients remain around 0.95 in the 1-72h prediction due to the elimination of the error accumulation. It was worse than the MF-PredRNN with the historical SWH before 10h but better than it after 10h. Overall, for the prediction of SWH in the BYECS, our MF-PredRNN-based 2D SWH prediction model significantly improves the accuracy and extends the effective prediction time length.
更多查看译文
关键词
multi factors-PredRNN, significant wave height, spatiotemporal forecast, long time prediction, memory decouple
AI 理解论文
溯源树
样例
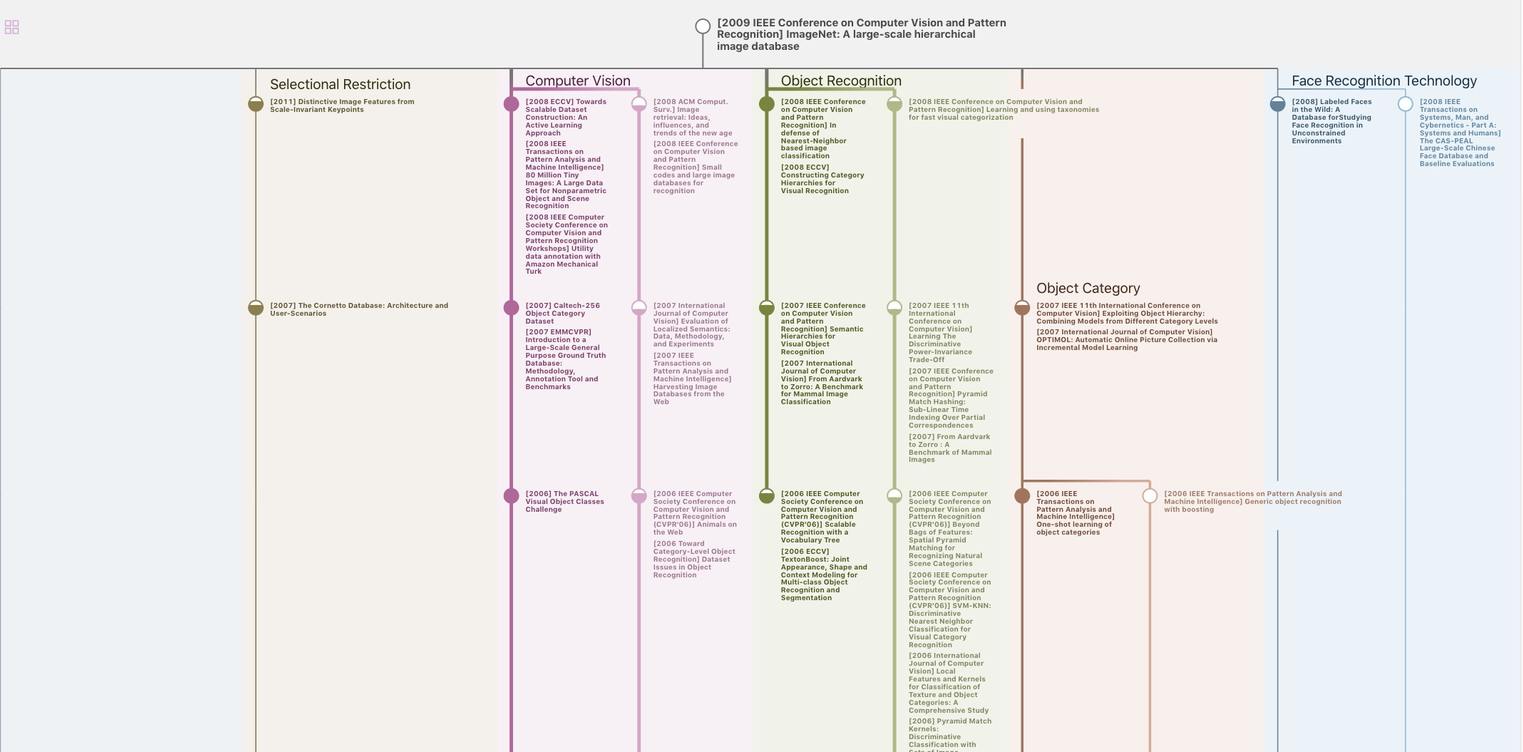
生成溯源树,研究论文发展脉络
Chat Paper
正在生成论文摘要