Segmentation of high-speed flow fields using physics-informed clustering
APPLICATIONS IN ENERGY AND COMBUSTION SCIENCE(2023)
摘要
The advent of data-based modeling has provided new methods and algorithms for analyzing the complex flow fields in high-speed combustion applications. These techniques can be used to study the fluid dynamics and reaction progress in different regions of the flow, which can provide insight into the underlying physics of the system while helping one identify avenues for the development and application of new models. The present work implements two such algorithms - the standard K-means clustering algorithm and a modified Jacobian-scaled K-means clustering algorithm - to analyze a high-speed reacting flow field. The results show that the Jacobian-scaled K-means algorithm allocates more clusters in the regions of the thermochemical state space where chemical source term is highest. In physical space, this concentrates the flow partitions in regions where heat release rate is higher and chemical timescales are lower. This is observed for different cluster numbers and data sets. When moving between data sets that occupy different portions of the state space, the cluster centroid locations are found to be less sensitive to the data set when the Jacobian-scaled K-means algorithm is used. Altogether, the modified K-means algorithm provides a new tool for identifying thermodynamic and thermochemical similarities in and across compressible reactive flow fields.
更多查看译文
关键词
Clustering, K-means, Flow segmentation, High-speed combustion, Physics-informed machine learning
AI 理解论文
溯源树
样例
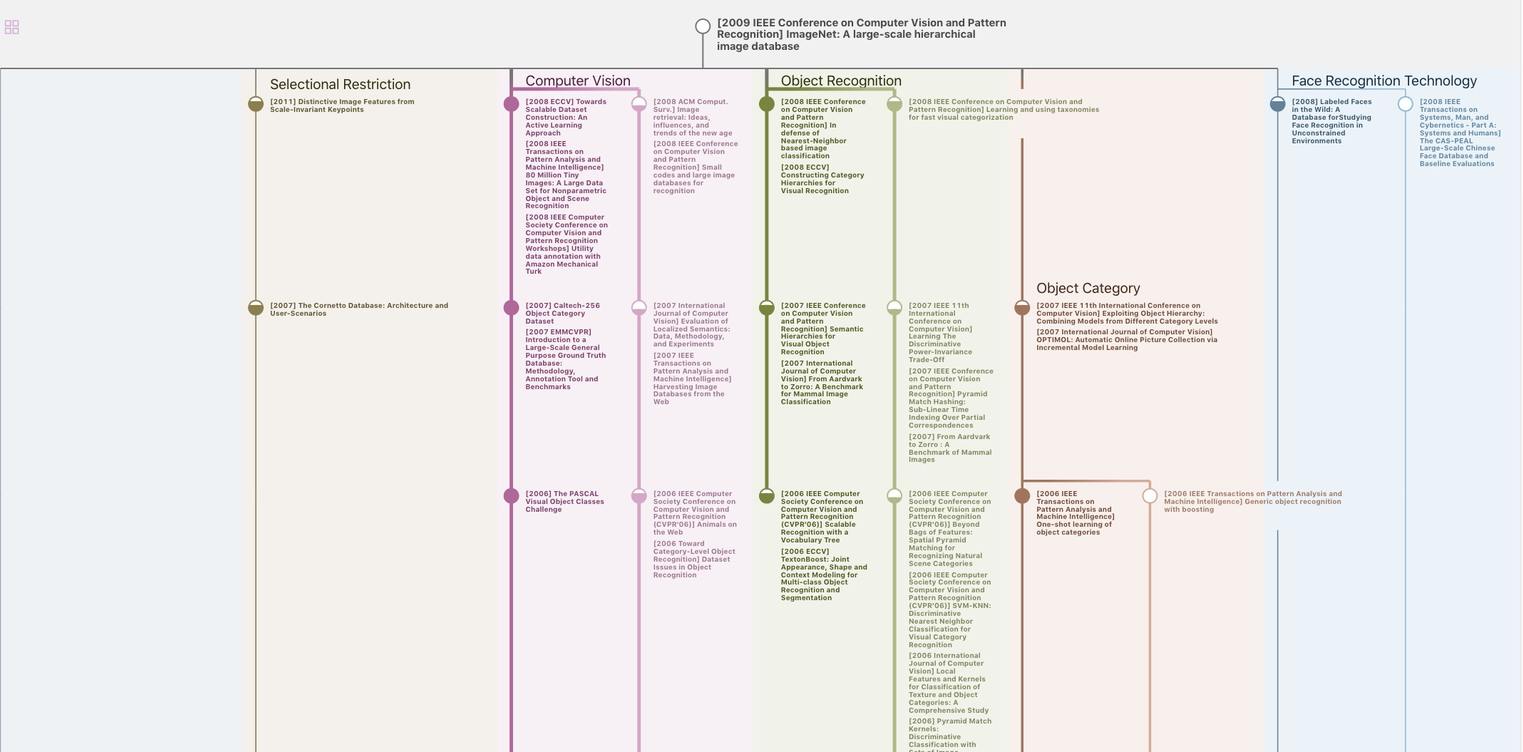
生成溯源树,研究论文发展脉络
Chat Paper
正在生成论文摘要