A Curriculum Learning Approach for Multi-Domain Text Classification Using Keyword Weight Ranking
ELECTRONICS(2023)
摘要
Text classification is a well-established task in NLP, but it has two major limitations. Firstly, text classification is heavily reliant on domain-specific knowledge, meaning that a classifier that is trained on a given corpus may not perform well when presented with text from another domain. Secondly, text classification models require substantial amounts of annotated data for training, and in certain domains, there may be an insufficient quantity of labeled data available. Consequently, it is essential to explore methods for efficiently utilizing text data from various domains to improve the performance of models across a range of domains. One approach for achieving this is through the use of multi-domain text classification models that leverage adversarial training to extract domain-shared features among all domains as well as the specific features of each domain. After observing the varying distinctness of domain-specific features, our paper introduces a curriculum learning approach using a ranking system based on keyword weight to enhance the effectiveness of multi-domain text classification models. The experimental data from Amazon reviews and FDU-MTL datasets show that our method significantly improves the efficacy of multi-domain text classification models adopting adversarial learning and reaching state-of-the-art outcomes on these two datasets.
更多查看译文
关键词
keyword weight ranking,curriculum learning approach,learning approach,classification,multi-domain
AI 理解论文
溯源树
样例
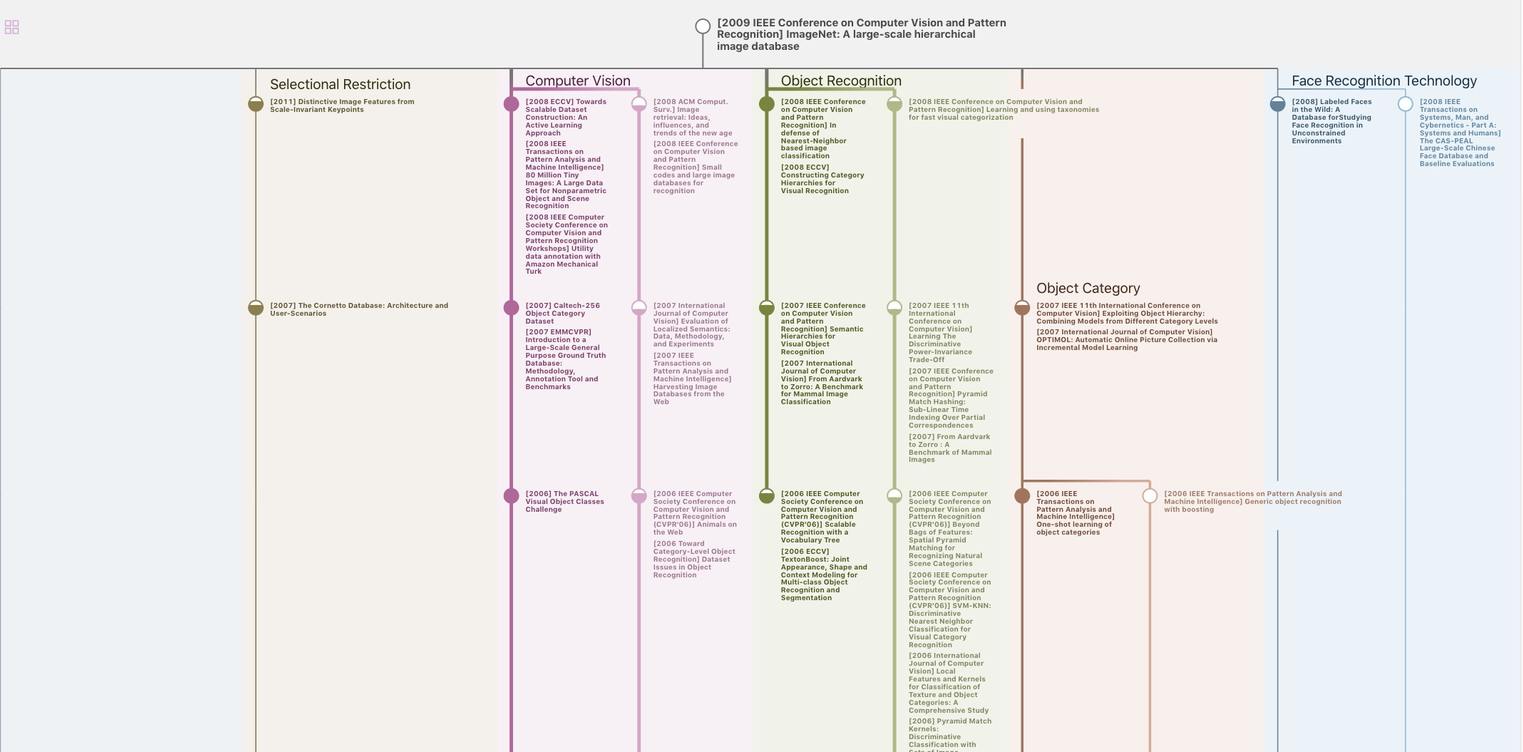
生成溯源树,研究论文发展脉络
Chat Paper
正在生成论文摘要