Global priors guided modulation network for joint super-resolution and SDRTV-to-HDRTV
Neurocomputing(2023)
摘要
Watching low resolution standard dynamic range (LR SDR) video on a 4K high dynamic range (HDR) TV is not the best viewing experience. Joint super-resolution (SR) and SDRTV-to-HDRTV aims to enhance the visual quality of LR SDR videos that have quality deficiencies in resolution and dynamic range. Previous methods that rely on learning local information typically cannot do well in preserving color conformity and long-range structural similarity, resulting in unnatural color transition and texture artifacts. In order to tackle these challenges, we propose a global priors guided modulation network (GPGMNet). In particular, we design a global priors extraction module (GPEM) to extract color conformity prior and structural similarity prior that are beneficial for SDRTV-to-HDRTV and SR tasks, respectively. To further exploit the global priors and preserve spatial information, we devise multiple global priors-guided spatial-wise modulation blocks (GSMBs) with a few parameters for intermediate feature modulation. In these GSMBs, the modulation parameters are generated by the shared global priors and the spatial features map from the spatial pyramid convolution block (SPCB). With these elaborate designs, the GPGMNet can achieve higher visual quality with lower computational complexity. Extensive experiments demonstrate that our proposed GPGMNet is superior to the state-of-the-art methods. Specifically, our proposed model exceeds the state-of-the-art by 0.64 dB in PSNR, with 69% fewer parameters and 3.1× speedup.
更多查看译文
关键词
Convolutional neural network,Super-resolution,SDRTV-to-HDRTV,High dynamic range
AI 理解论文
溯源树
样例
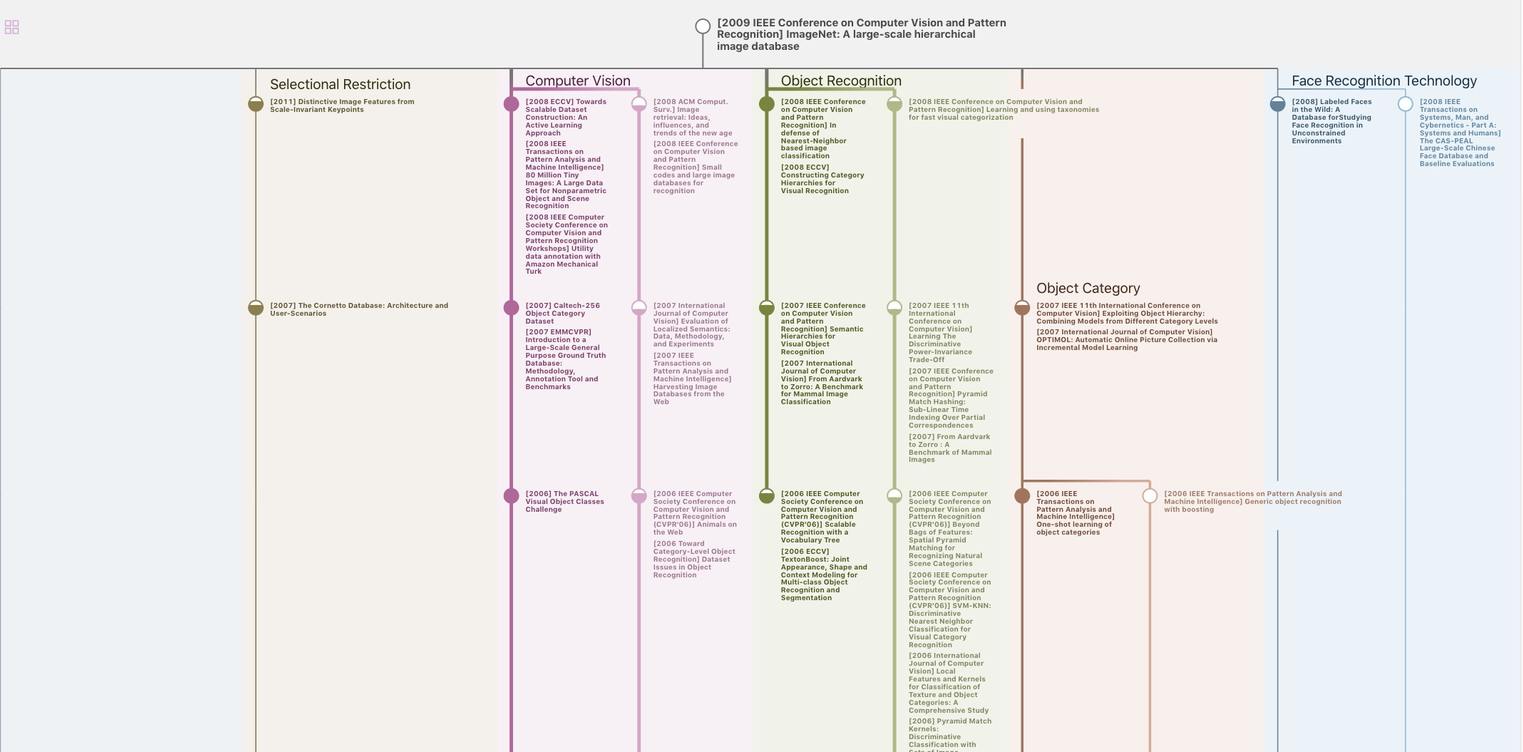
生成溯源树,研究论文发展脉络
Chat Paper
正在生成论文摘要