Sequence tagging with a rethinking structure for joint entity and relation extraction
INTERNATIONAL JOURNAL OF MACHINE LEARNING AND CYBERNETICS(2024)
摘要
Joint entity and relation extraction have become increasingly popular due to their knowledge graph construction advantages. Despite the promising results on this task, disregarding the multi-triple interaction poses a problem since multi-triples commonly appear in sentences and interact implicitly. This paper proposes a sequence tagging with a rethink structure method for joint extraction and builds upon a Seq2Seq framework. The model starts with a sentence encoder that vectorizes input text. Then the vectors are fed into a sequence tagging component to acquire pre-extracted triples. Afterward, a newly designed rethinking structure is utilized as a decoder, double-checking the pre-extracted triples. Specifically, the pre-extracted triples serve as the decoder's query vector. Thus, the decoder can simultaneously generate triples in a non-autoregressive manner. Since the decoder is aware of the entire set of per-extracted triples, it can effectively model the interactions. The model was comprehensively compared to state-of-the-art (SOTA) benchmarks on widely used datasets, NYT and WebNLG. The result shows that the extraction performance of our model is superior to that of SOTA, especially when the sample contains sufficient triple interactions.
更多查看译文
关键词
Relation extraction,Sequence tagging,Rethinking structure,Non-autoregressive decoding
AI 理解论文
溯源树
样例
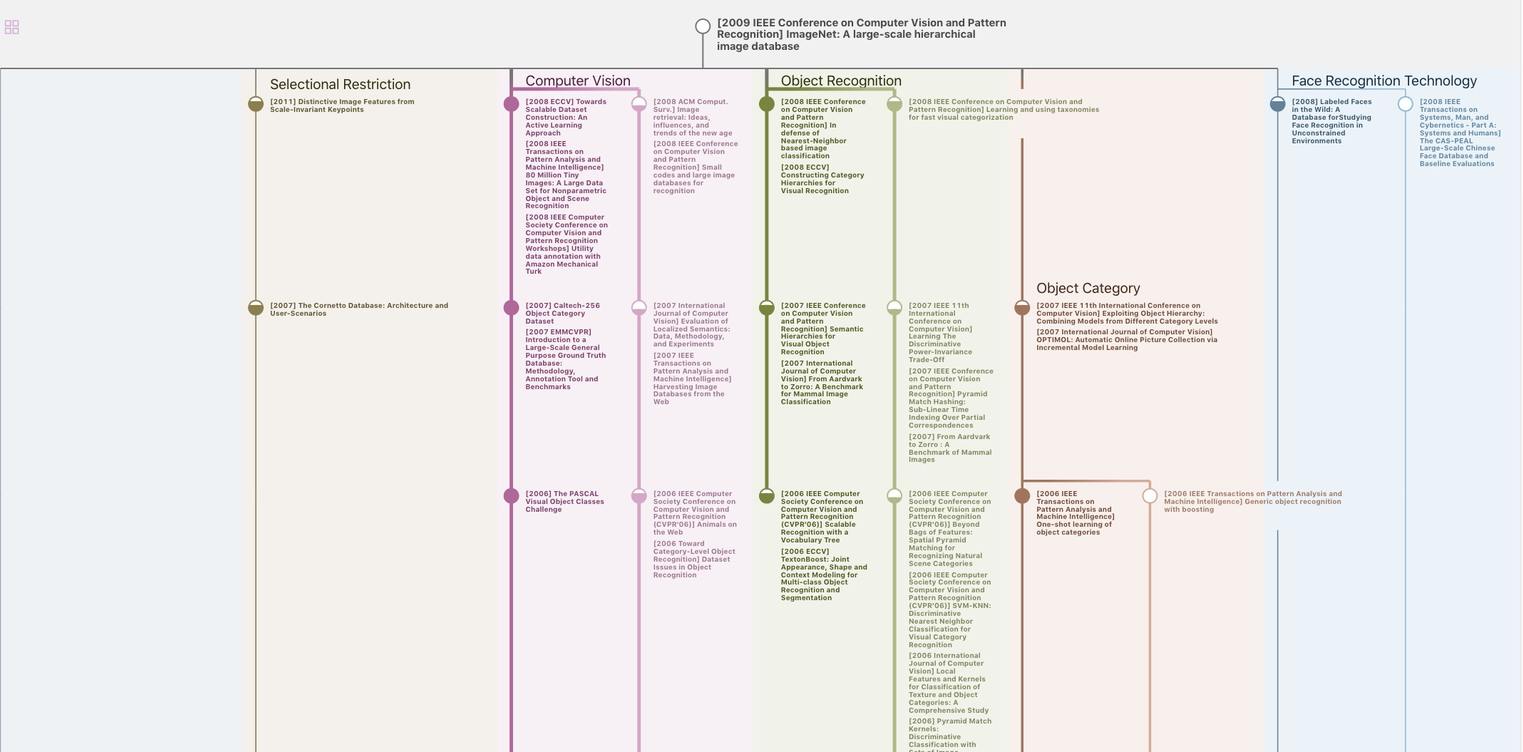
生成溯源树,研究论文发展脉络
Chat Paper
正在生成论文摘要