A comparison of machine learning and econometric models for pricing perpetual Bitcoin futures and their application to algorithmic trading
EXPERT SYSTEMS(2023)
摘要
Bitcoin (BTC) perpetual futures contracts are highly leveraged speculative trading instruments with daily market trading of $45 Billion. BTC perpetual futures are derivative contracts, which depend upon the underlying BTC SPOT (current) price. Pricing perpetual futures fairly is hard, using traditional arbitrage arguments, because of the volatile nature of the so called funding rate, which is used as the replacement of risk free rate in the Cryptocurrency market. This work presents a novel technique for pricing BTC futures contracts using conditional volatility and mean models. Intra-day high-frequency futures' return volatility and mean are modelled using different ML and econometric techniques. A comparison is made using statistical measures to find the model that best captures the intra-day conditional mean and volatility. Exponential generalized autoregressive conditional heteroskedasticity is shown to be an almost unbiased predictor of intra-day volatility, while a constant autoregressive moving average (0, 0) model best captures the conditional mean of the returns. A market directional high frequency trading algorithm is developed using the volatility and mean models. The algorithm first prices the futures contract at some future point of time using the volatility and mean regression models. Next, the slope between the current futures price and the expected price are used to predict the market direction. A long or short position is taken depending upon the expected market direction movement. Extensive back-testing results show absolute returns of 1500%-8000% depending upon the transaction fees and leverage used. On average, the market direction is predicted correctly 85% of the time by the best model. Finally, the trading technique is market neutral, in that it gives large positive returns, with low SD, in both bull and bear markets.
更多查看译文
关键词
perpetual bitcoin futures,econometric models,machine learning
AI 理解论文
溯源树
样例
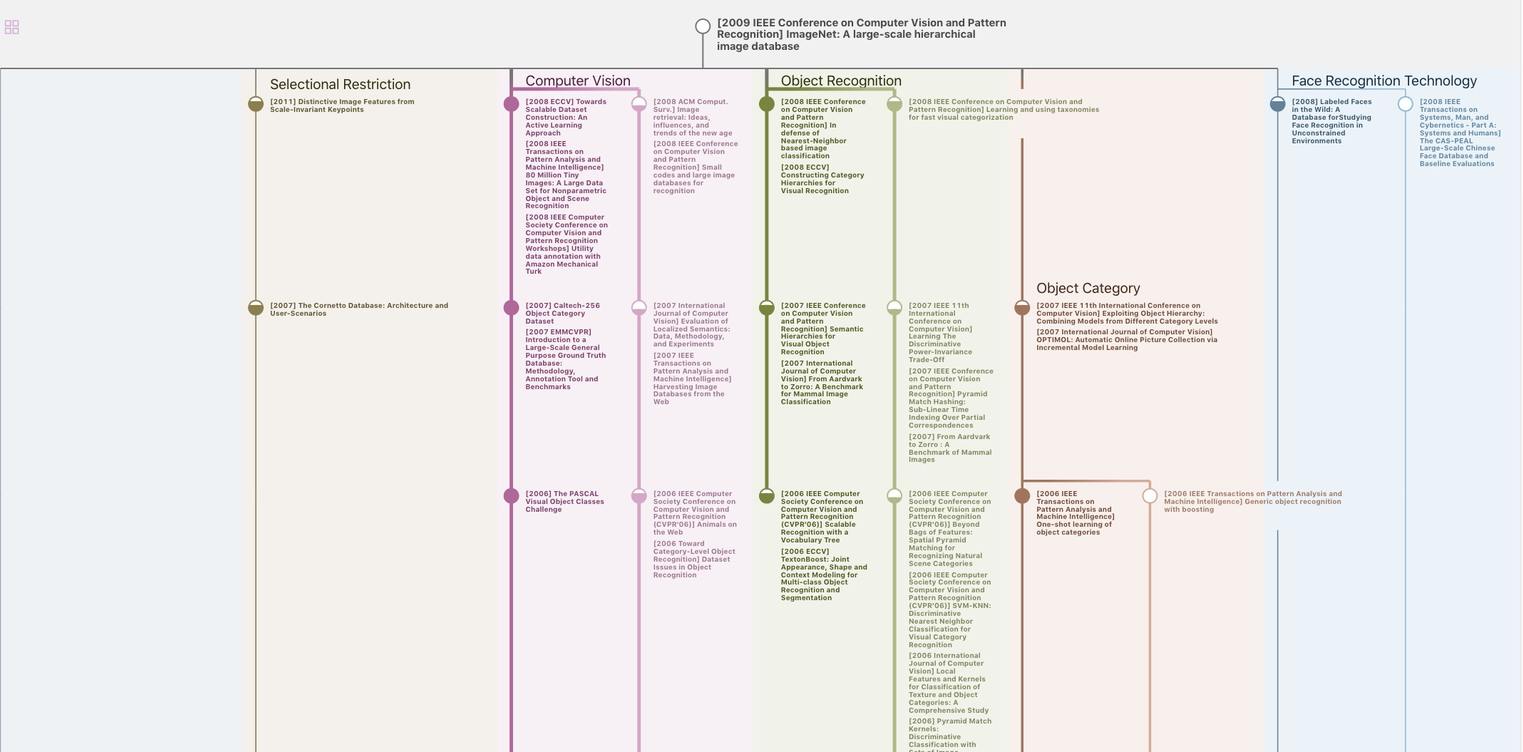
生成溯源树,研究论文发展脉络
Chat Paper
正在生成论文摘要