A Cross-Level Interaction Network Based on Scale-Aware Augmentation for Camouflaged Object Detection
IEEE TRANSACTIONS ON EMERGING TOPICS IN COMPUTATIONAL INTELLIGENCE(2024)
摘要
Camouflaged object detection (COD), with the task of separating the camouflaged object from its color/texture similar background, has been widely used in the fields of medical diagnosis and military reconnaissance. However, the COD task is still a challenging problem due to two main difficulties: large scale-variation for different camouflaged objects, and extreme similarity between the camouflaged object and its background. To address these problems, a cross-level interaction network based on scale-aware augmentation (CINet) for the COD task is proposed. Specifically, a scale-aware augmentation module (SAM) is firstly designed to perceive the scales information of the camouflaged object by calculating an optimal receptive field. Furthermore, a cross-level interaction module (CLIM) is proposed to facilitate the interaction of scale information at all levels, and the context of the feature maps is enriched accordingly. Finally, with the purpose of fully utilizing these features, we design a dual-branch feature decoder (DFD) to strengthen the connection between the predictions at each level. Extensive experiments performed on four CODdatasets, e.g., CHAMELEON, CAMO, COD10K, and NC4K, demonstrate the superiority of the proposed CINet compared with 21 existing state-of-the-art methods.
更多查看译文
关键词
Index Terms-Camouflaged object detection,large scale-variation,interactive learning
AI 理解论文
溯源树
样例
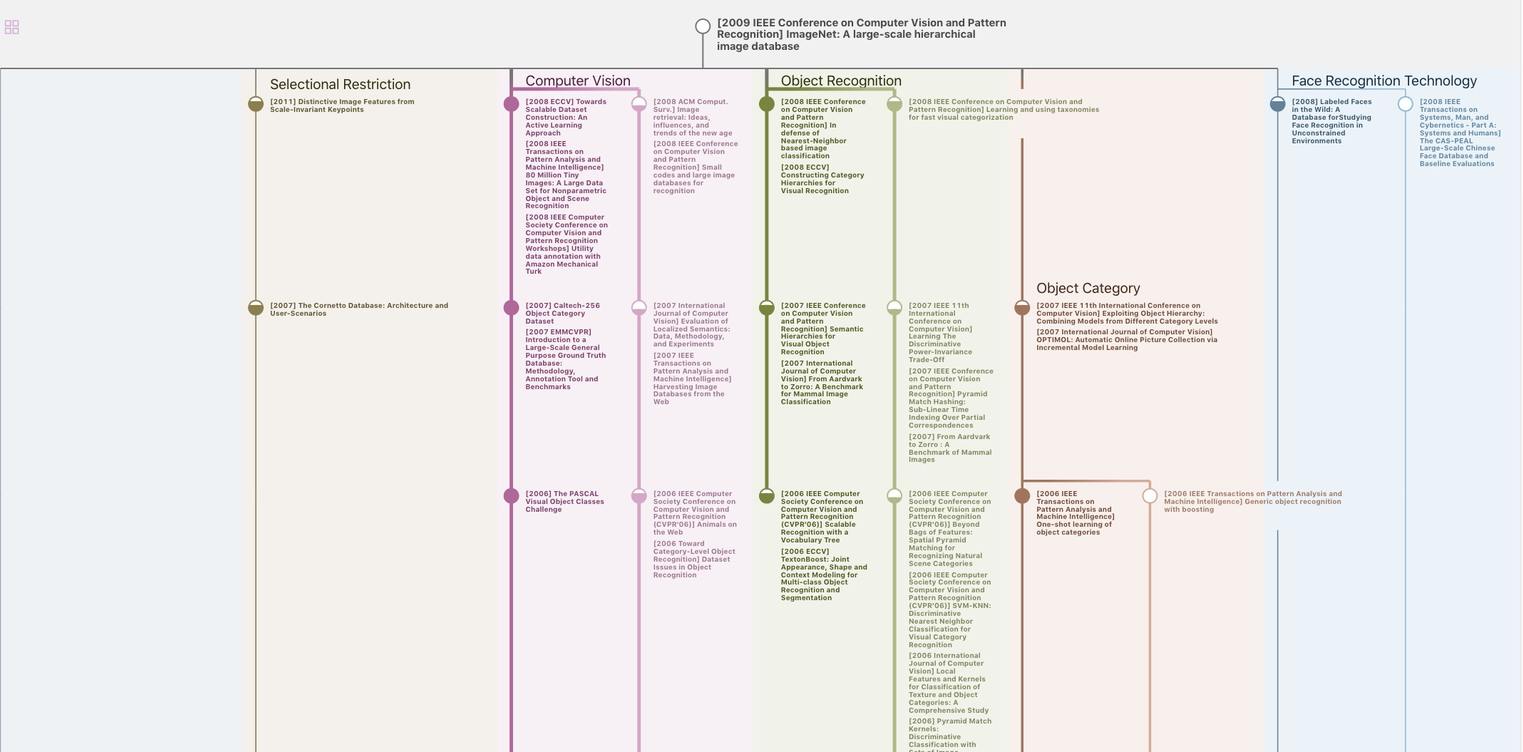
生成溯源树,研究论文发展脉络
Chat Paper
正在生成论文摘要