Kernelized multi-granulation fuzzy rough set over hybrid attribute decision system and application to stroke risk prediction
APPLIED INTELLIGENCE(2023)
摘要
Searching for quantitative scientific prediction models and methods under uncertain hybrid information environment is helpful to the scientific decision-making of practical management problems. In this paper, we discuss the uncertain decision making problem with hybrid attributes and fuzzy decision objects. Considering the characteristic of hybrid attribute information, we introduce kernel functions to abstract the similarities from different types of attributes. On this basis, the kernel-based upper and lower approximations of arbitrary fuzzy decision objects over hybrid attribute information system are given under a multi-granularity framework, i.e., kernelized multi-granulation fuzzy rough sets, called KMGFRS in this paper. Then, the attribute reduction on hybrid attribute decision system based on KMGFRS is discussed. In addition, we combine KMGFRS and fuzzy k -nearest neighbors (F k NN) to propose a new prediction method without prior information and apply it to stroke risk assessment and prediction in clinical decision-making. Finally, we use real clinical data and UCI datasets for experiment analysis, and the results verify the applicability and validity of the model.
更多查看译文
关键词
Rough set, Multi-granulation fuzzy rough set, Multi-kernel learning, Fuzzy kNN, Stroke risk prediction
AI 理解论文
溯源树
样例
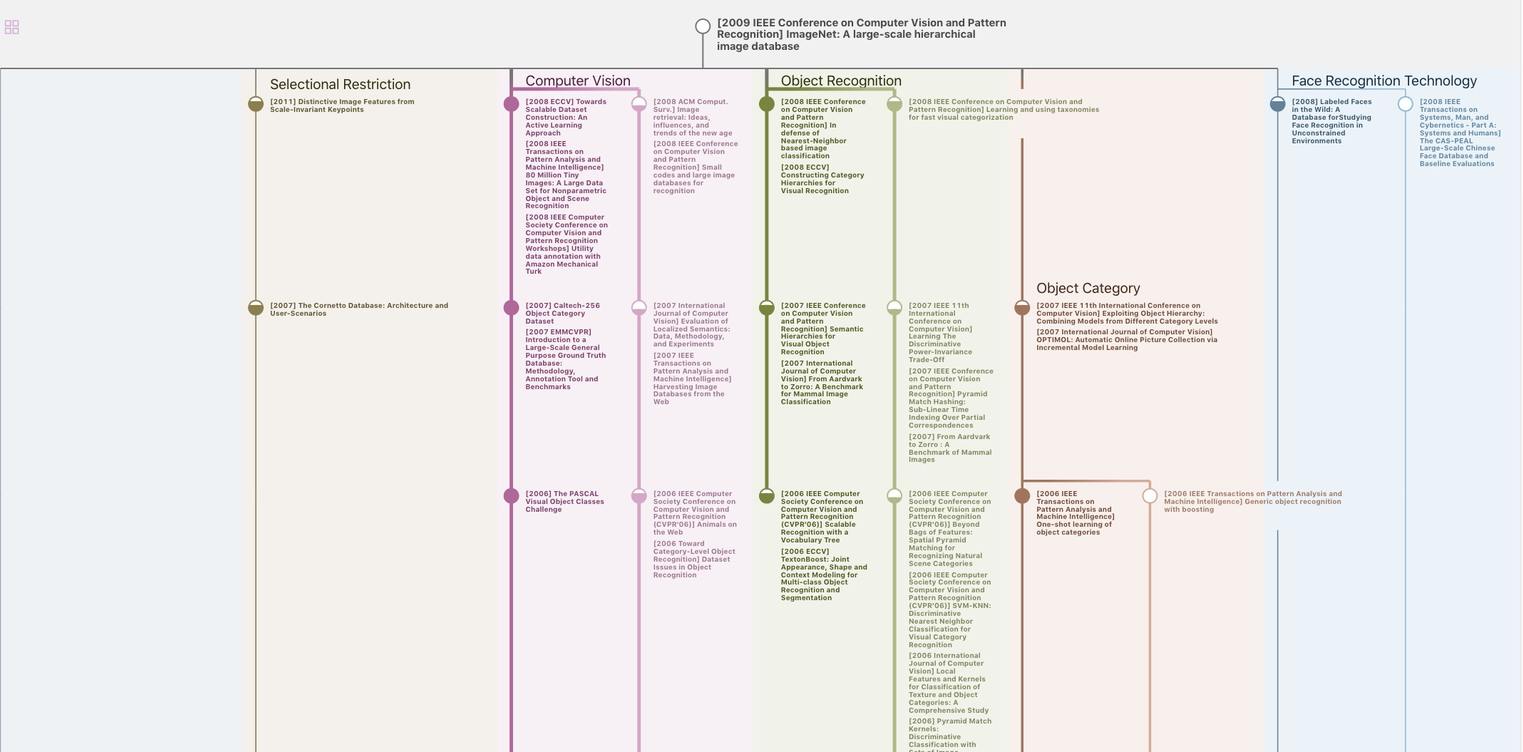
生成溯源树,研究论文发展脉络
Chat Paper
正在生成论文摘要