Density Matrix-Based Features as Descriptors for Oxygen Reduction and Evolution Catalysts
JOURNAL OF PHYSICAL CHEMISTRY C(2023)
摘要
Developing cost-effective and efficient catalysts containingnonpreciousmetals is critical for chemical-to-electrical conversion technologies.The onset potentials for the fundamentally important oxygen reductionreaction, oxygen evolution reaction, and hydrogen evolution reactioncan be determined from binding free energies. Herein, artificial neuralnetworks (ANNs) were trained on a dataset of approximately 1500 metal-nitrogen-dopedcarbon (MNC) complexes containing first-row transition metals to predictOOH, O, OH, and H binding free energies from transferable densitymatrix-based features. These ANNs use density matrices from gas-phaseHartree-Fock theory with a minimal basis set for a fixed geometryto predict binding free energies at the level of solution-phase densityfunctional theory (DFT) with a much larger basis set for optimizedgeometries. The ANNs were able to predict binding free energies witha mean absolute error of around 0.1 eV. Several feature selectiontools such as recursive feature elimination were used to decreasethe number of density matrix-based features and increase accuracy.The off-diagonal density matrix elements between the metal and ligatingnitrogens were found to be especially predictive of binding free energies.This machine learning strategy has the potential to facilitate thediscovery of efficient and abundant metal-based catalysts for electrochemicalenergy conversion.
更多查看译文
关键词
catalysts,oxygen reduction,density,matrix-based
AI 理解论文
溯源树
样例
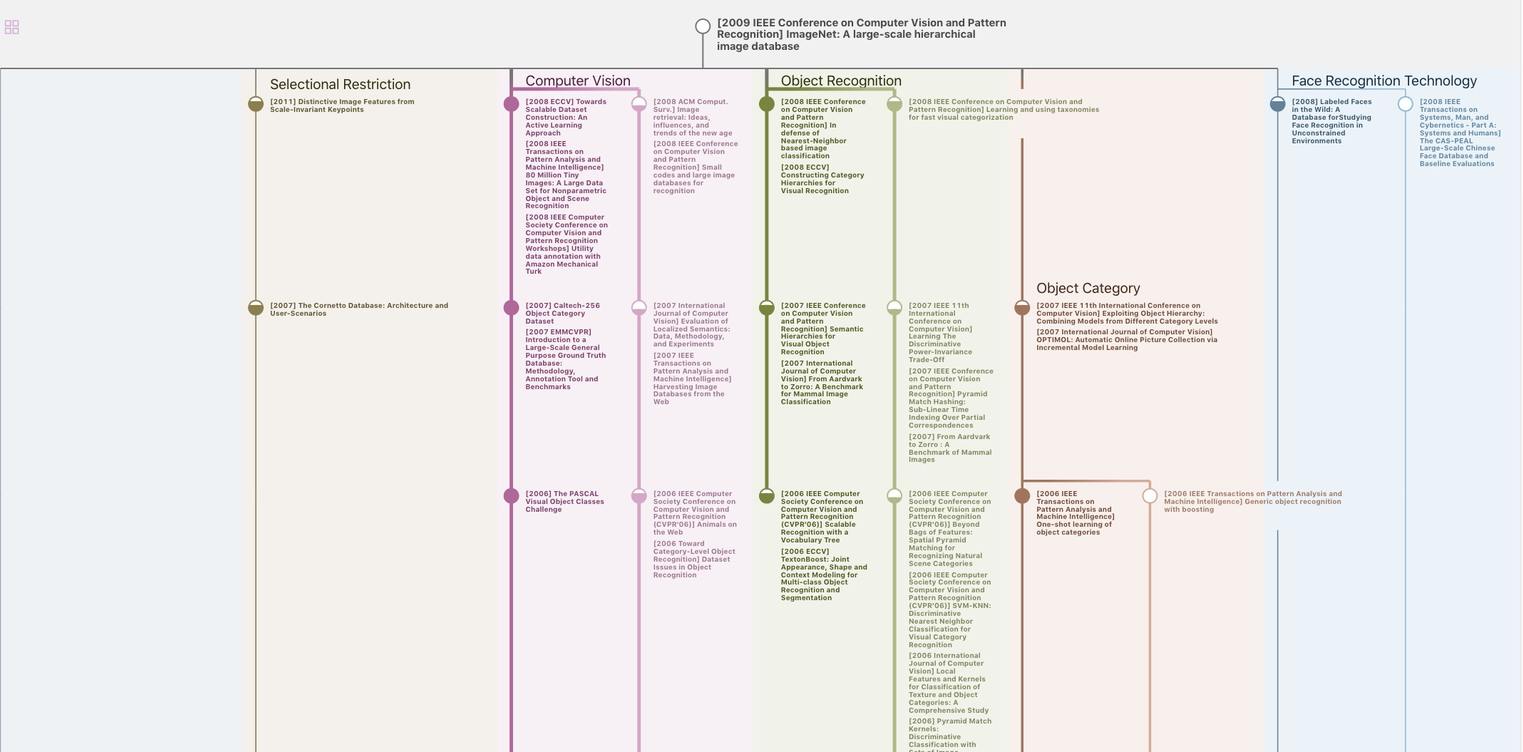
生成溯源树,研究论文发展脉络
Chat Paper
正在生成论文摘要