Fine-grained Image Recognition via Attention Interaction and Counterfactual Attention Network
ENGINEERING APPLICATIONS OF ARTIFICIAL INTELLIGENCE(2023)
摘要
Learning subtle and discriminative regions plays an important role in fine-grained image recognition, and attention mechanisms have shown great potential in such tasks. Recent research mainly focuses on employing the attention mechanism to locate key discriminative regions and learn salient features, whilst ignoring imperceptible complementary features and the causal relationship between prediction results and attention. To address the above issues, we propose an Attention Interaction and Counterfactual Attention Network (AICA-Net). Specifically, we propose an Attention Interaction Fusion Module (AIFM) to model the negative correlation between the attention map channels to locate the complementary features, and fuse the complementary features and key discriminative features to generate richer fine-grained features. Simultaneously, an Enhanced Counterfactual Attention Module (ECAM) is proposed to generate a counterfactual attention map. By comparing the impact of the learned attention map and the counterfactual attention map on the final prediction results, quantifying the quality of attention drives the network to learn more effective attention. Extensive experiments on CUB-200-2011, FGVC-Aircraft and Stanford Cars datasets have shown that our AICA-Net can get outstanding results. In particular, it achieves 90.83% and 95.87% accuracy on two open competitive benchmark datasets CUB-200-2011 and Stanford Cars, respectively. Experiments demonstrate that our method outperforms state-of-the-art solutions.
更多查看译文
关键词
Fine-grained image recognition, Counterfactual attention, Attention interaction, Attention mechanism
AI 理解论文
溯源树
样例
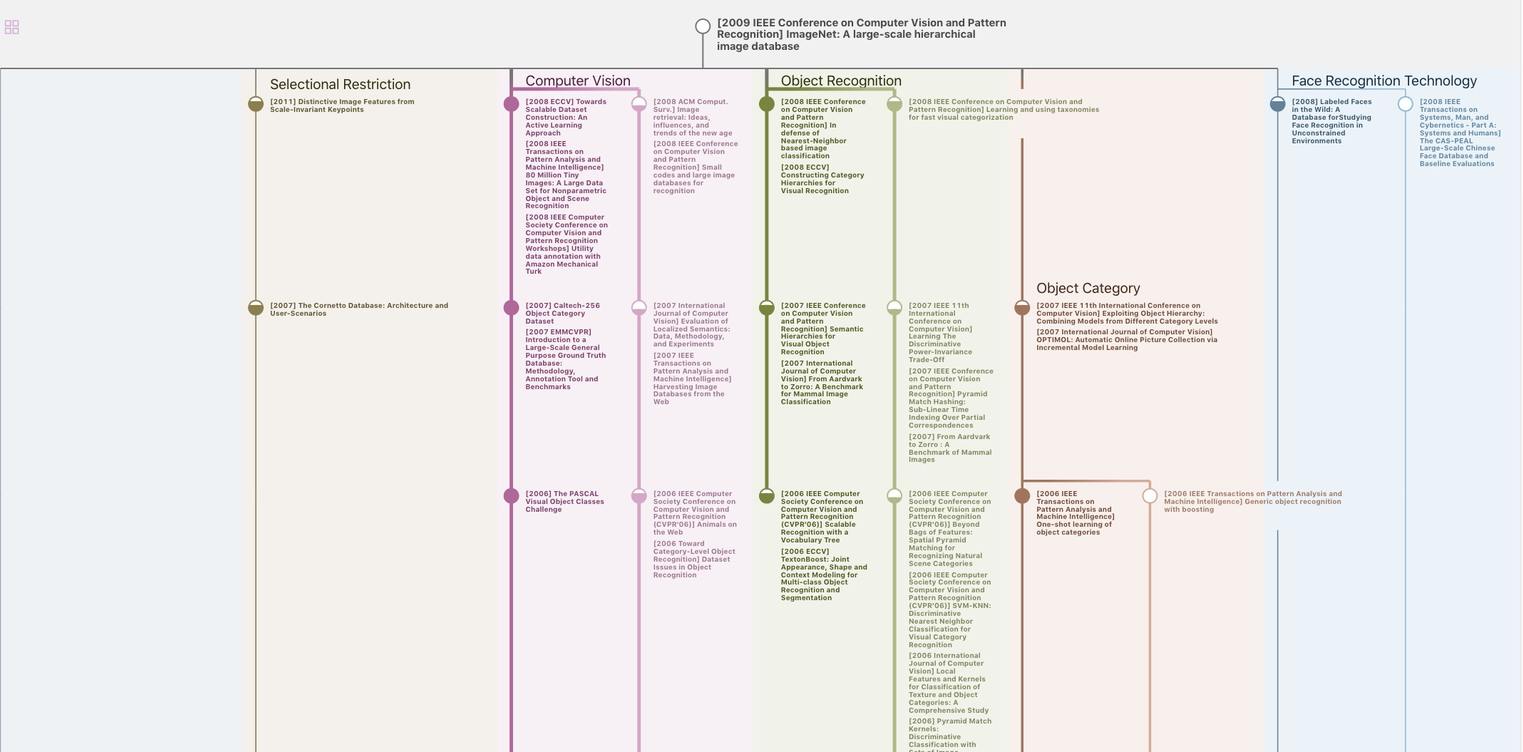
生成溯源树,研究论文发展脉络
Chat Paper
正在生成论文摘要