Approaching feature matrix: To solve two issues in link prediction
EXPERT SYSTEMS WITH APPLICATIONS(2023)
摘要
With the increase of graph-structure data, link prediction has become an active research topic. Recent progress in link prediction involves learning graph embeddings through graph neural networks. Graph autoencoders(GAE), variational graph autoencoders(VGAE), and their variants such as graph normalized autoencoders(GNAE) try to learn graph embeddings in an unsupervised setting and show promising results in link prediction. However, they suffer from degraded performance with two issues; they tend to assign close-to-zero embedding on nodes with zero degree (i.e., isolated node problem) and the issue caused by random negative sampling has not been addressed in link prediction experiments. As the two issues affect only links and not feature information, we propose a generic learning method based on the property of feature information that alleviates both issues and outperforms strong baselines across benchmark datasets.
更多查看译文
关键词
Link prediction,Graph embedding,Isolated node,Random negative sampling
AI 理解论文
溯源树
样例
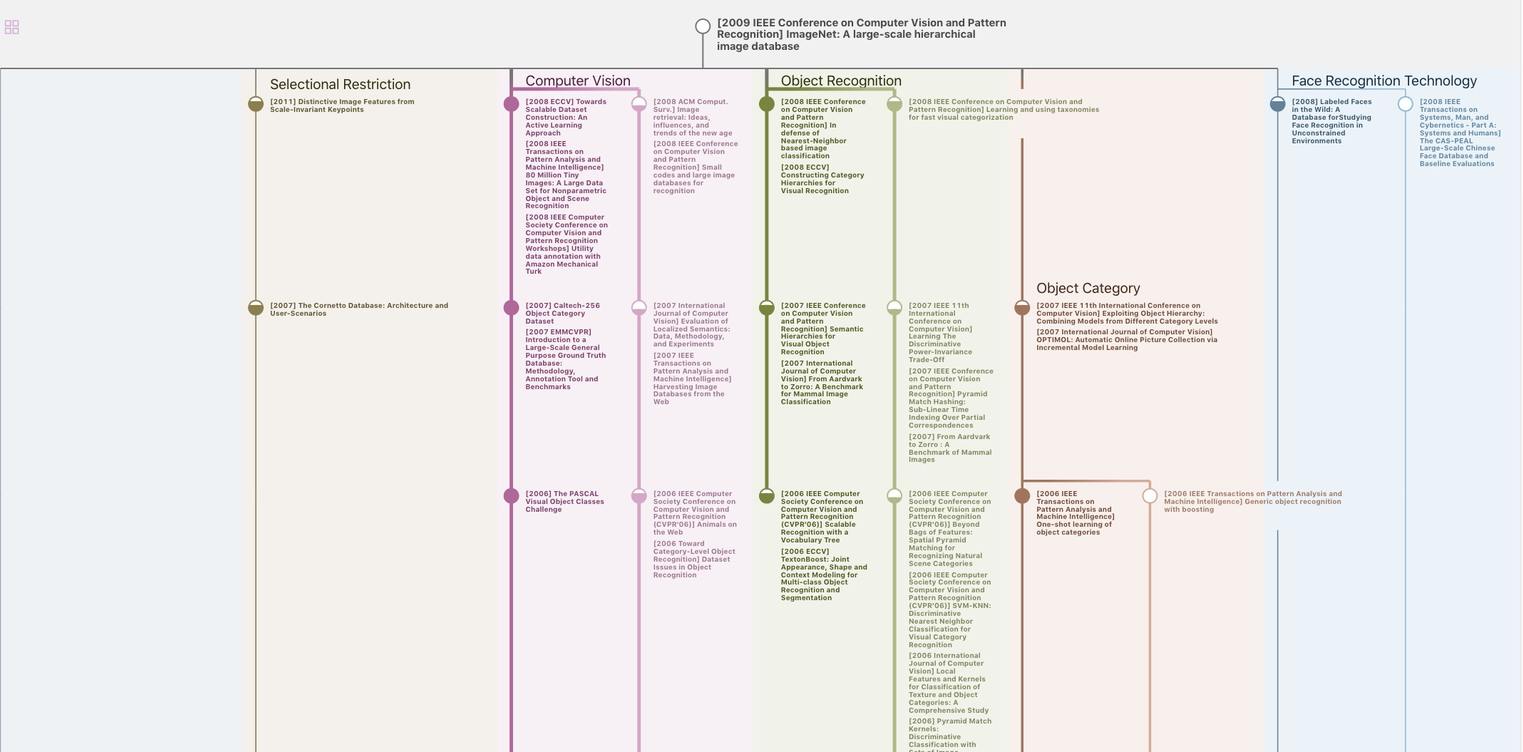
生成溯源树,研究论文发展脉络
Chat Paper
正在生成论文摘要