Data-Driven Models Informed by Spatiotemporal Mobility Patterns for Understanding Infectious Disease Dynamics
ISPRS INTERNATIONAL JOURNAL OF GEO-INFORMATION(2023)
摘要
Data-driven approaches predict infectious disease dynamics by considering various factors that influence severity and transmission rates. However, these factors may not fully capture the dynamic nature of disease transmission, limiting prediction accuracy and consistency. Our proposed data-driven approach integrates spatiotemporal human mobility patterns from detailed point-of-interest clustering and population flow data. These patterns inform the creation of mobility-informed risk indices, which serve as auxiliary factors in data-driven models for detecting outbreaks and predicting prevalence trends. We evaluated our approach using real-world COVID-19 outbreaks in Beijing and Guangzhou, China. Incorporating the risk indices, our models successfully identified 87% (95% Confidence Interval: 83-90%) of affected subdistricts in Beijing and Guangzhou. These findings highlight the effectiveness of our approach in identifying high-risk areas for targeted disease containment. Our approach was also tested with COVID-19 prevalence data in the United States, which showed that including the risk indices reduced the mean absolute error and improved the R-squared value for predicting weekly case increases at the county level. It demonstrates applicability for spatiotemporal forecasting of widespread diseases, contributing to routine transmission surveillance. By leveraging comprehensive mobility data, we provide valuable insights to optimize control strategies for emerging infectious diseases and facilitate proactive measures against long-standing diseases.
更多查看译文
关键词
infectious disease dynamics,spatiotemporal mobility patterns,infectious disease,data-driven
AI 理解论文
溯源树
样例
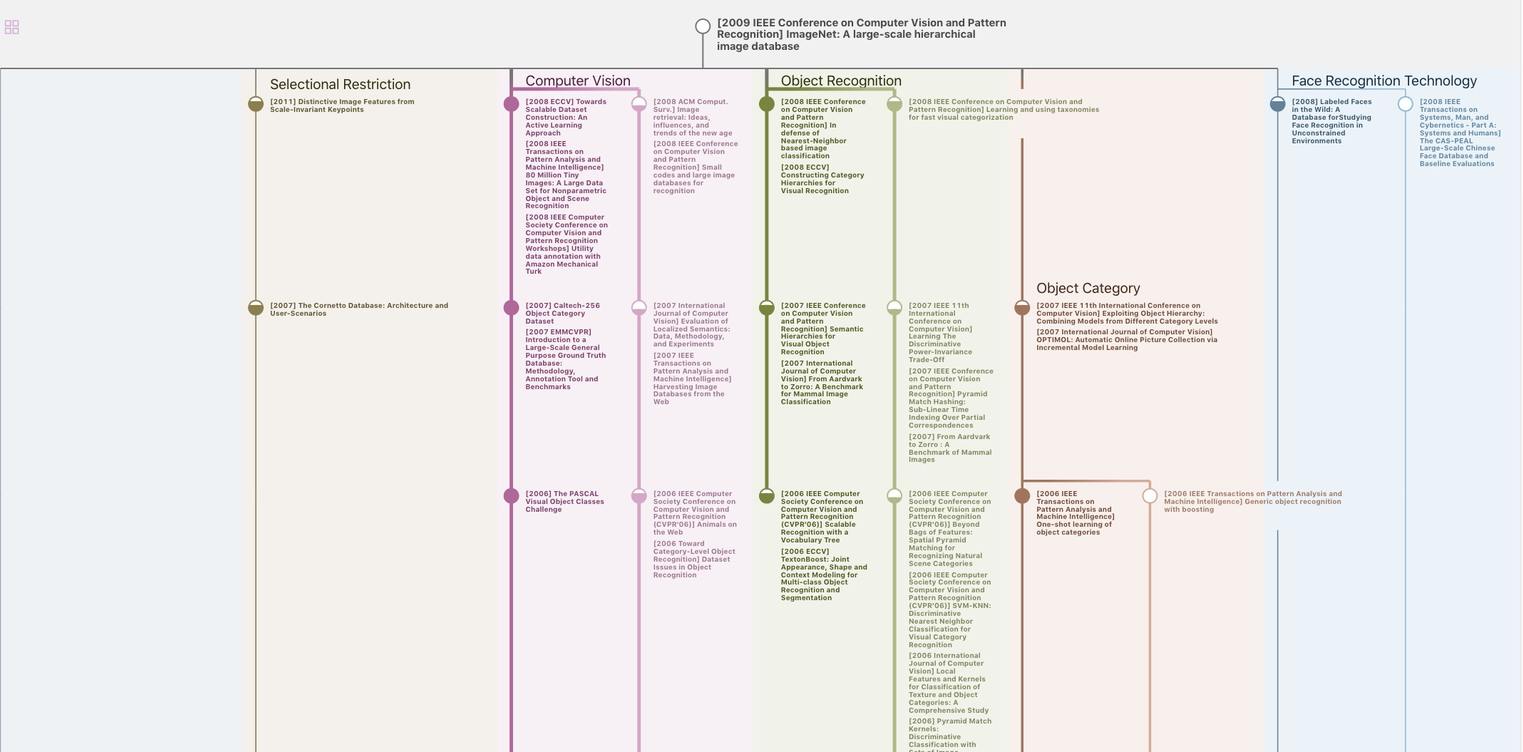
生成溯源树,研究论文发展脉络
Chat Paper
正在生成论文摘要