Sparse matrix-vector and matrix-multivector products for the truncated SVD on graphics processors
CONCURRENCY AND COMPUTATION-PRACTICE & EXPERIENCE(2023)
摘要
Many practical algorithms for numerical rank computations implement an iterative procedure that involves repeated multiplications of a vector, or a collection of vectors, with both a sparse matrix A$$ A $$ and its transpose. Unfortunately, the realization of these sparse products on current high performance libraries often deliver much lower arithmetic throughput when the matrix involved in the product is transposed. In this work, we propose a hybrid sparse matrix layout, named CSRC, that combines the flexibility of some well-known sparse formats to offer a number of appealing properties: (1) CSRC can be obtained at low cost from the popular CSR (compressed sparse row) format; (2) CSRC has similar storage requirements as CSR; and especially, (3) the implementation of the sparse product kernels delivers high performance for both the direct product and its transposed variant on modern graphics accelerators thanks to a significant reduction of atomic operations compared to a conventional implementation based on CSR. This solution thus renders considerably higher performance when integrated into an iterative algorithm for the truncated singular value decomposition (SVD), such as the randomized SVD or, as demonstrated in the experimental results, the block Golub-Kahan-Lanczos algorithm.
更多查看译文
关键词
graphics processing units,singular value decomposition,sparse matrix-multivector product,sparse matrix-vector product
AI 理解论文
溯源树
样例
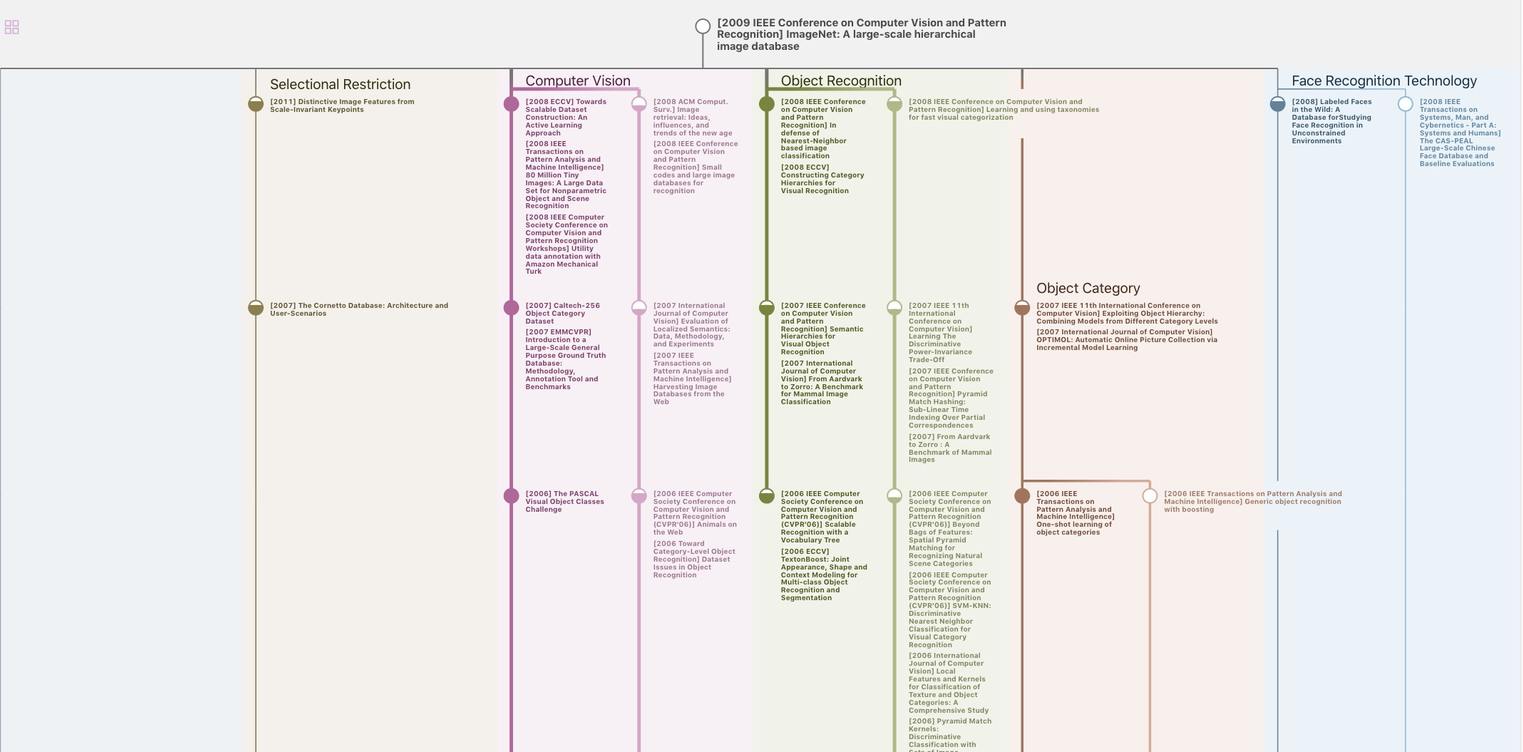
生成溯源树,研究论文发展脉络
Chat Paper
正在生成论文摘要