Accelerating Materials Discovery through Machine Learning: Predicting Crystallographic Symmetry Groups
JOURNAL OF PHYSICAL CHEMISTRY C(2023)
摘要
Predicting crystalstructure from the chemical composition is oneof the most challenging and long-standing problems in condensed matterphysics. This problem resides at the interface between materials sciencesand physics. With reliable data and proper physics-guided modeling,machine learning (ML) can provide an alternative venue to undertakeand reduce the problem's complexity. In this work, very robustML classifiers for crystallographic symmetry groups were developedand applied for ternary (A( l )B( m )C( n )) and binary (A( l )B( m )) materialsstarting only from the chemical formula. This is the first essentialstep toward predicting full geometry. Such a problem is highly multi-labeland multi-class from an ML perspective and requires careful preprocessingdue to the size imbalance of the data. The resulting predictive modelsare highly accurate for all symmetry groups, including crystal systems,point groups, Bravais lattices, and space groups, with weighted balancedaccuracies exceeding 95%. The models were developed with only a smallset of ionic and compositional features, namely, stoichiometry, ionicradii, ionization energies, and oxidation states for each elementin the ternary and binary compounds. Considering such minimal featurespace, the obtained high accuracies ascertain that the physics iswell captured. This is even further confirmed as we demonstrate thatthe accuracy of our approach is limited only by the size of data bycomparing the size of ternary and binary materials with the accuracyof developed models. The presented work could effectively contributeto accelerating new materials discovery and development.
更多查看译文
关键词
materials discovery,machine learning,symmetry
AI 理解论文
溯源树
样例
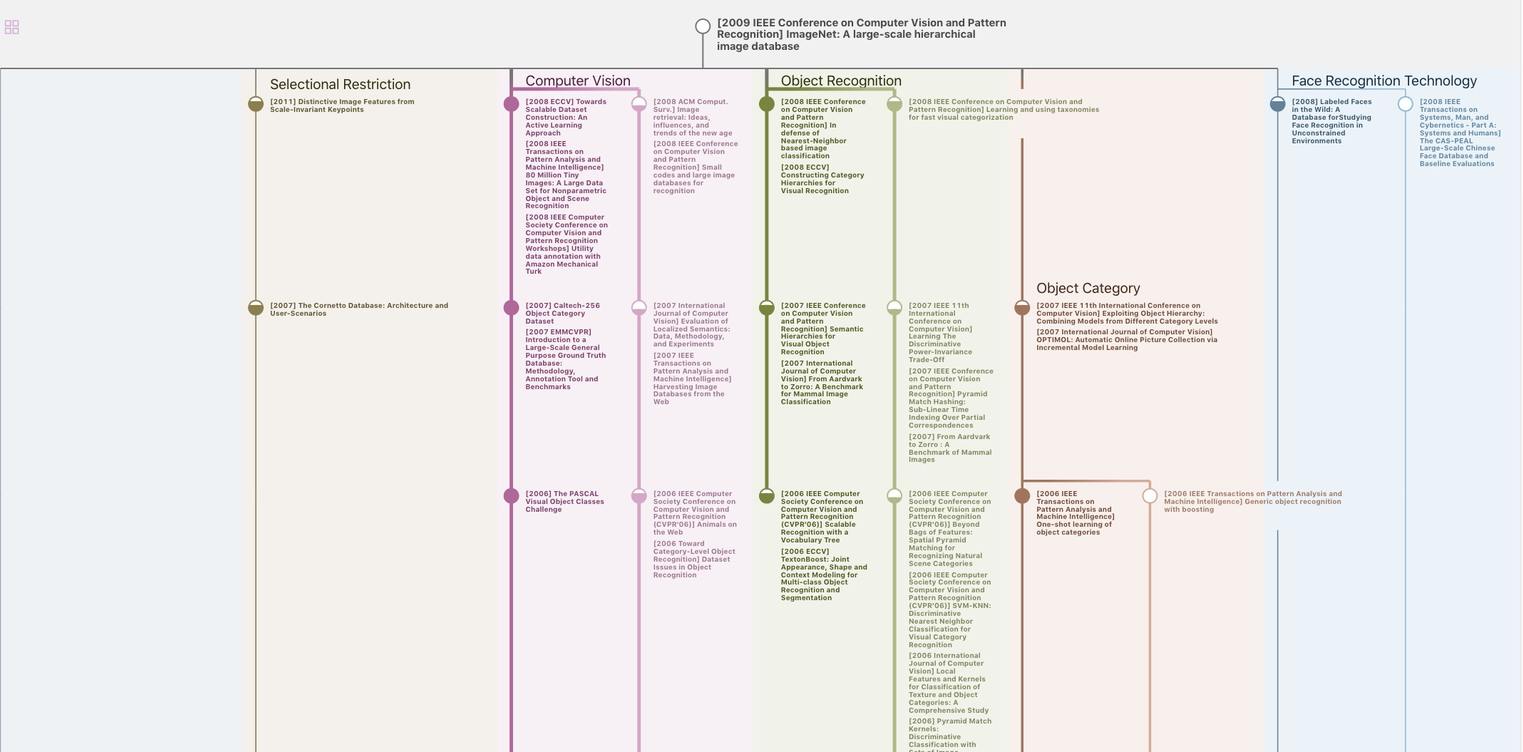
生成溯源树,研究论文发展脉络
Chat Paper
正在生成论文摘要