FGCR: Fused graph context-aware recommender system
Knowl. Based Syst.(2023)
摘要
Context-aware recommender systems (CARS), which capture user preferences by incorporating user interactions and side information, have attracted widespread attention in today’s information age. With the emergence of graph convolution networks (GCN) in recent years, graph methods have been shown effective in establishing global connections between users, items, and contexts. In this paper, we first introduce a unified graph recommendation framework to incorporate both GCN and traditional models, showing the similarities and differences between them in terms of parameter configurations and message passing styles. Then, based on the empirical analysis of existing models, we propose a novel Fused Graph Context-aware Recommender system (FGCR) model to address the identified limitations. FGCR fuses the parameter configuration of both item-based models and GCN, modeling robust node relationships in user-item-context interaction graph through a sparse item correlation matrix and dense node embeddings. In addition, FGCR applies a novel masked graph convolution strategy to refine the information aggregation process with various types of nodes. Experiments conducted on five real-world datasets show that FGCR significantly improves performance compared to the seven baseline models. Ablation study and user group analysis validate the effectiveness of each component in FGCR, especially in modeling active users and sparse contextual information.
更多查看译文
关键词
Context-aware recommendation,Recommender system,Graph neural network
AI 理解论文
溯源树
样例
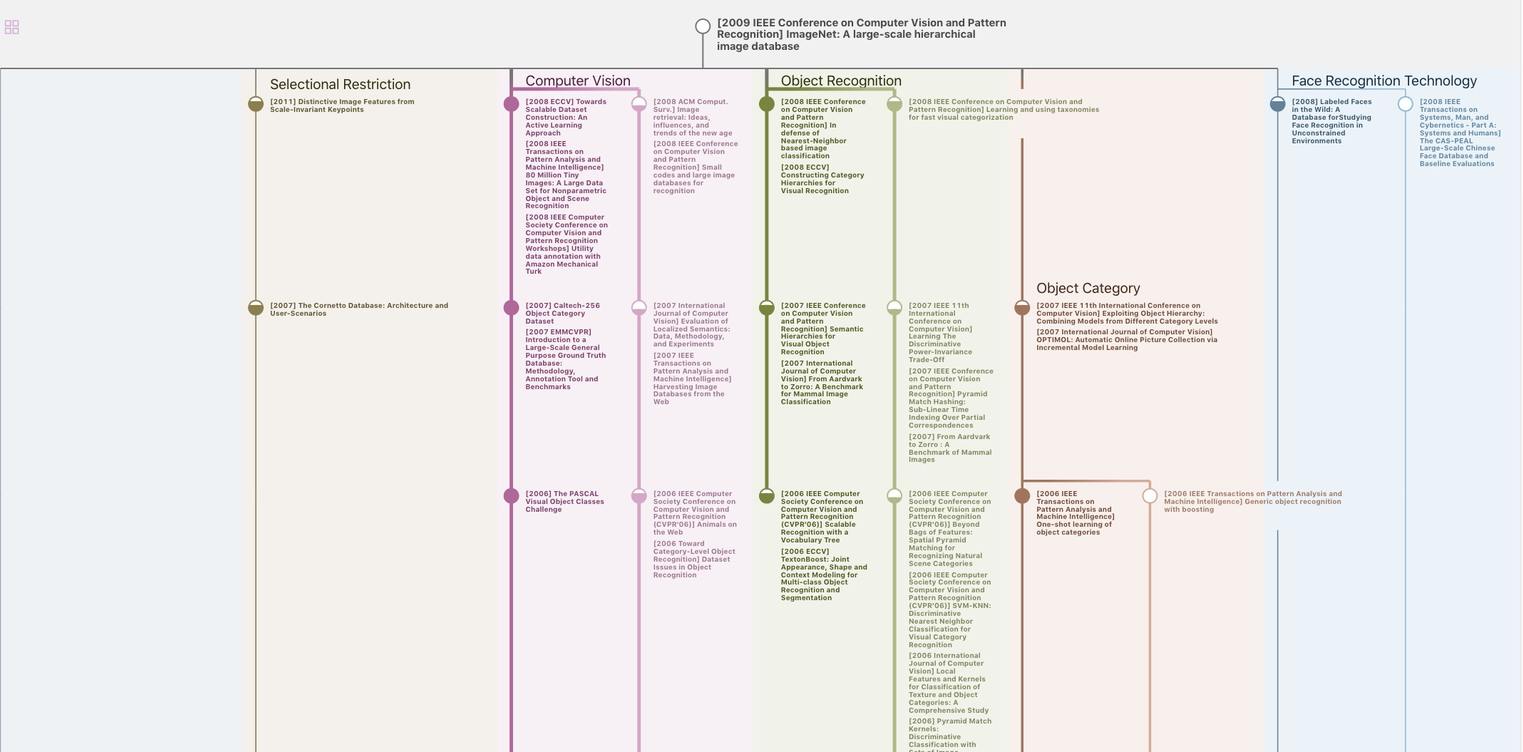
生成溯源树,研究论文发展脉络
Chat Paper
正在生成论文摘要