Maximal sparse convex surrogate-assisted evolutionary convolutional neural architecture search for image segmentation
COMPLEX & INTELLIGENT SYSTEMS(2024)
摘要
Designing reasonable architectures of convolutional neural network (CNN) for specific image segmentation remains a challenging task, as the determination of the structure and hyperparameters of CNN depends heavily on expertise and requires a great deal of time. Evolutionary algorithm (EA) has been successfully applied to the automatic design of CNNs; however, the inherent stochastic search of EA tends to cause "experience loss" and requires very large computational resources. To deal with this problem, a maximal sparse convex surrogate model with updated empirical information is proposed in this paper to guide the evolutionary process of CNN design. This sparse convex function is transformed from a non-convex function to a maximized sparse convex function, which can better utilize the prior empirical knowledge to assist the evolutionary search. In addition, a balance strategy between computational resources and accuracy is proposed in the selection of reasonable network architectures. The proposed fully automatic design method of CNN is applied to the segmentation of steel microstructure images, and experimental results demonstrate that the proposed method is competitive with the existing state-of-the-art methods.
更多查看译文
关键词
Convolutional neural networks,Neural architecture search,Evolutionary algorithm,Surrogate model,Image segmentation
AI 理解论文
溯源树
样例
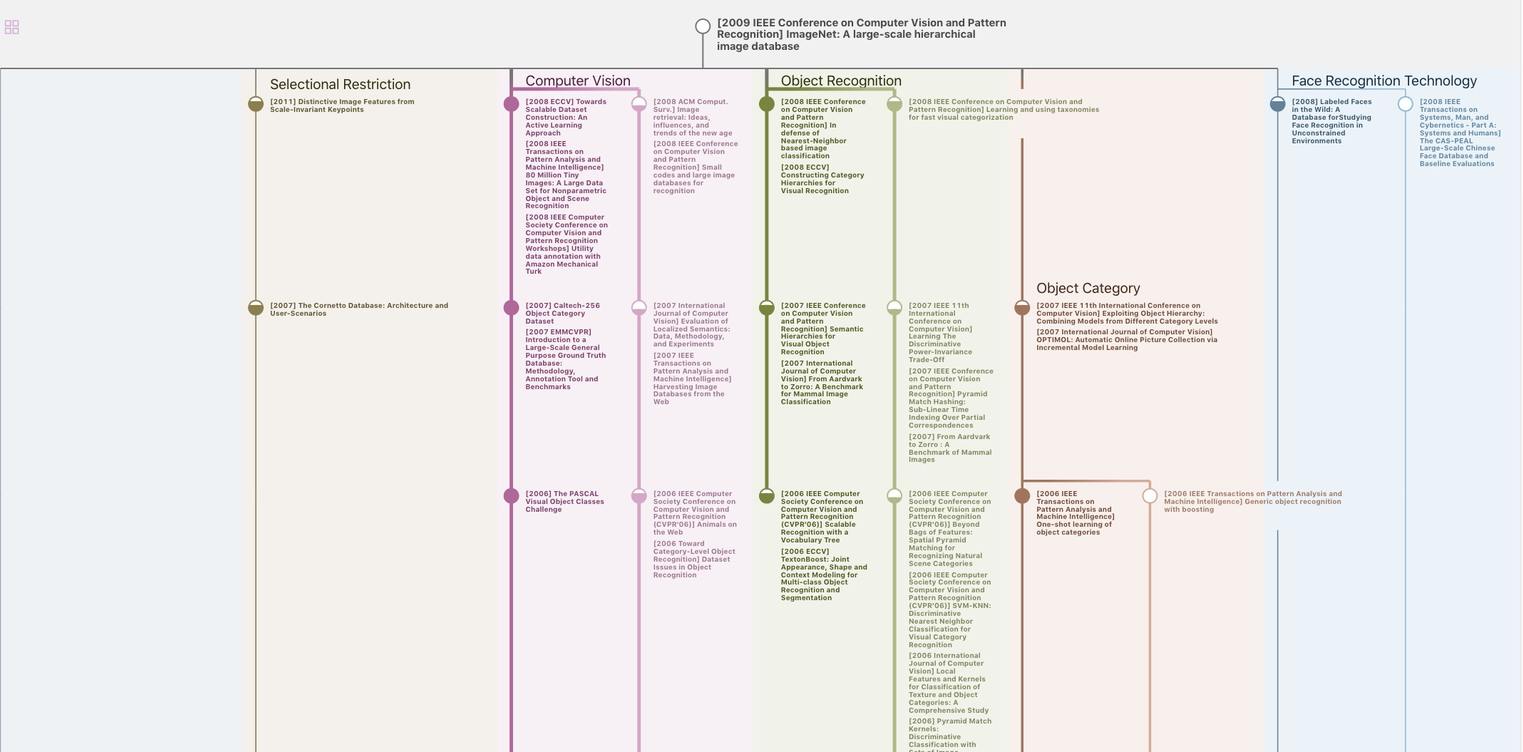
生成溯源树,研究论文发展脉络
Chat Paper
正在生成论文摘要