A geometry-driven neural topic model for trip purpose inference
GEOINFORMATICA(2023)
摘要
Understanding urban human mobility, particularly trip purposes, is essential for optimizing traffic management, personalized recommendations, and urban planning. However, in real-world scenarios, trip purposes cannot be directly extracted from the trajectory data. To address this issue, we propose a geometry-driven neural topic model for trip purpose inference. We integrate trajectory data with nearby POI data using a geometry-driven technique to enhance the interpretability of the results. Furthermore, our model captures the semantics and relationships of the data in a high-dimensional space and identifies latent topics representing distinct trip purposes. These learned topics are analyzed using clustering algorithms to group similar trips, enabling trip purpose inference. And we evaluate our model using the trajectory data of Shenzhen and Chengdu, and compare it with baseline models. The results demonstrate that our model performs well. Furthermore, we analyze trajectory data containing trip purpose information to gain insight into human mobility patterns and the influence of trip purposes, paving the way for potential implications and future research directions.
更多查看译文
关键词
Urban human mobility,Trip purposes inference,Neural network,Trip embedding
AI 理解论文
溯源树
样例
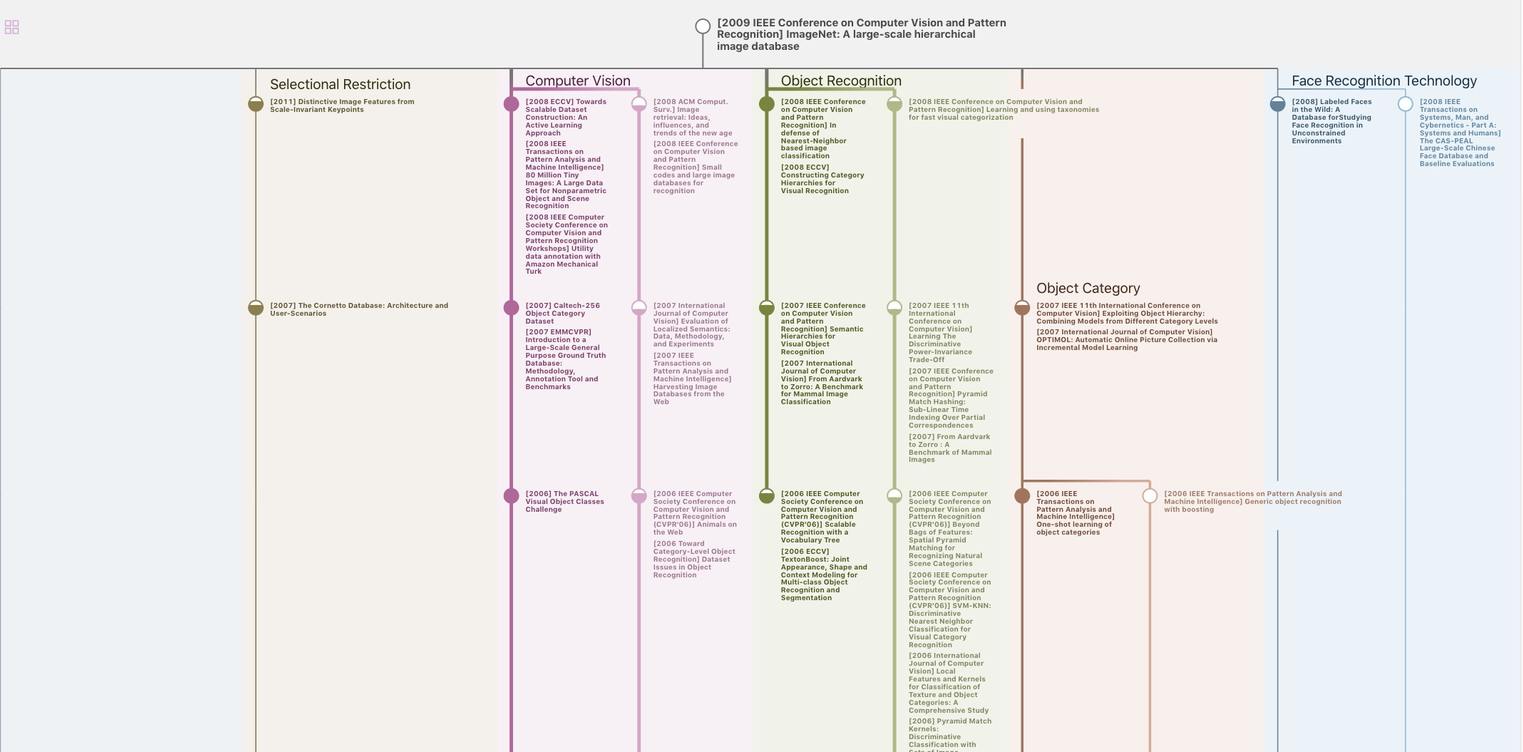
生成溯源树,研究论文发展脉络
Chat Paper
正在生成论文摘要