Attribute prediction of spatio-temporal graph nodes based on weighted graph diffusion convolution network
WORLD WIDE WEB-INTERNET AND WEB INFORMATION SYSTEMS(2023)
摘要
Spatio-temporal graph data can be analyzed by effectively mining for realizing spatio-temporal graph data prediction. It is of great significance to predict spatio-temporal graph attribute features of future moments under different scenarios. However, the current research represent the spatio-temporal graph as a fixed graph structure in the learning process and cannot describe real dependencies in spatial feature learning, which has great limitations and cannot effectively capture the dynamic dependence of spatio-temporal graph data. Therefore, this paper proposes a Deep Spatio-Temporal Graph Node Attribute Prediction Model Based on Weighted Graph Diffusion Convolution Network, named DST-WDCN. Firstly, the graph adjacency matrix is defined based on both the explicit graph structure and the hidden semantic relationships. Secondly, a dynamic weighting learning method is introduced to dynamically weight the graph structure, which is incorporated into the gated diffusion convolution for dynamic spatial correlation extraction. Thirdly, a gated dilated causal convolution is proposed to extract temporal features to fully model temporal nonlinear dependencies. Finally, the spatio-temporal convolution blocks are stacked to effectively capture the spatio-temporal dynamic correlation and realize the attribute prediction of the spatio-temporal graph in the future time. Extensive experiments over three real-world data sets have shown our proposed model is superior to the benchmark model in terms of MAE, RMSE and MAPE. The effectiveness of each component of the model has been verified through ablation experiments, and the adaptability of the dynamic weighting method has been demonstrated through learning cases.
更多查看译文
关键词
graph,prediction,diffusion,spatio-temporal
AI 理解论文
溯源树
样例
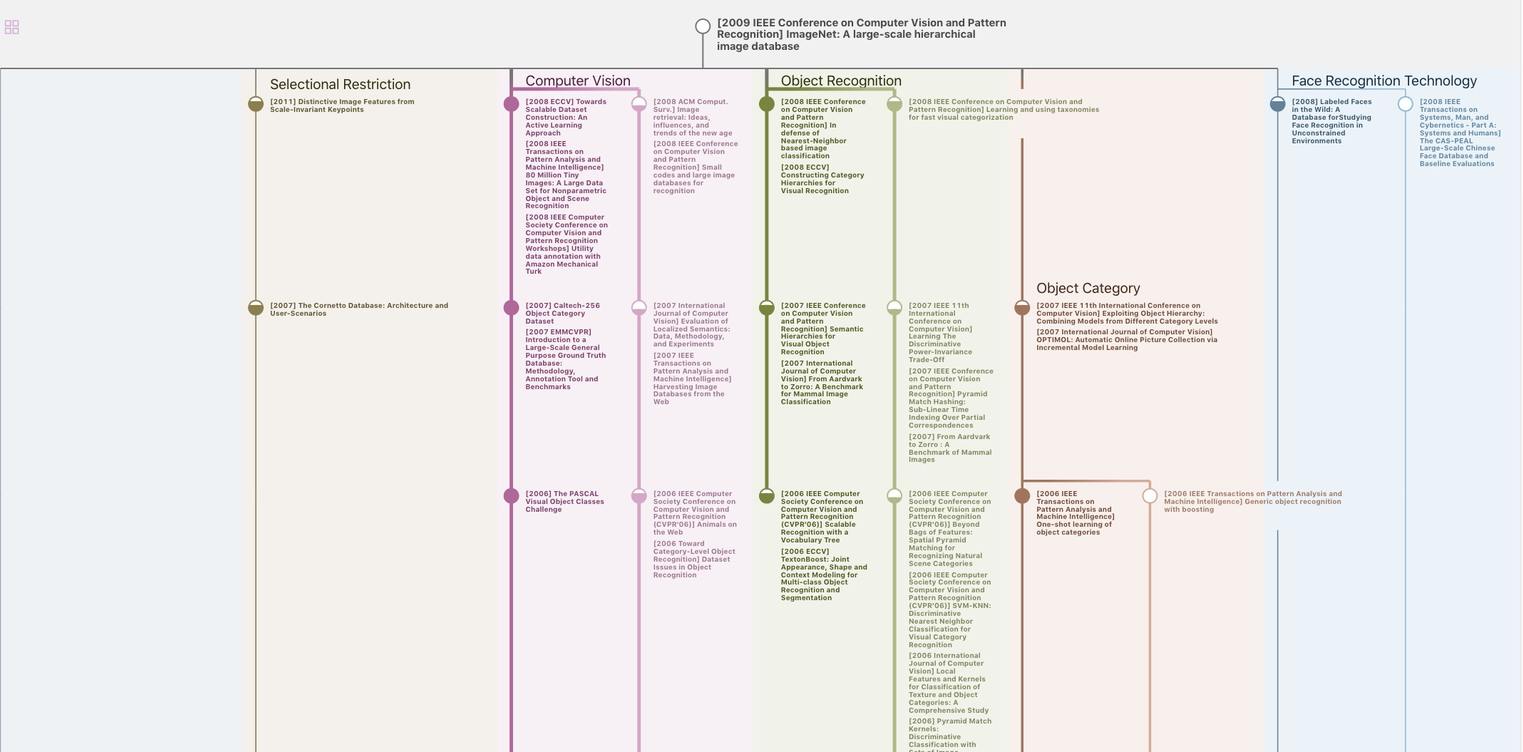
生成溯源树,研究论文发展脉络
Chat Paper
正在生成论文摘要