Estimating cooling capacities from aerial images using convolutional neural networks
APPLIED ENERGY(2023)
摘要
In recent decades, the global cooling demand has significantly increased and is expected to grow even further in the future. However, knowledge regarding the spatial distribution of cooling demand is sparse. Most existing studies are based on statistical modelling, which lack in small-scale details and cannot accurately identify in-dividual large cooling producers. In this study, we implement and apply a novel method to identify, map and estimate nominal cooling capacities of chillers using deep learning. Chillers typically use air-cooled condensers and cooling towers to release excess heat, and produce most of the cooling needs in the commercial and in-dustrial sectors. In this study, these units are identified from aerial images using specifically trained object detection models. The corresponding nominal cooling capacity is then estimated based on the number of fans of air-cooled condensers and the fan diameters of the cooling towers, respectively. Both detection and capacity estimations are first evaluated on test data sets and subsequently applied to an industrial area (Bruhl) and the city center in Freiburg, Germany. In Bruhl, aerial images show chillers with an estimated nominal cooling capacity of 205 MW, of which the model detected 88%, while 88% of all detections are correct. In the city center, a nominal capacity of 18.6 MW is estimated, of which the model detected 87% with 77% of all detections being correct. Hence, the developed approach facilitates a reliable analysis of the installed nominal cooling capacity of indi-vidual buildings at large scales, such as districts and cities. This information could be further used to locate areas for investments and support planning of eco-friendly, centralized supply of cooling energy, for example district heating and cooling systems or shallow geothermal energy systems such as aquifer thermal energy storage (ATES).
更多查看译文
关键词
Aerial images,Deep learning,Air-cooled chiller,Cooling tower,Cooling capacity,Urban energy planning
AI 理解论文
溯源树
样例
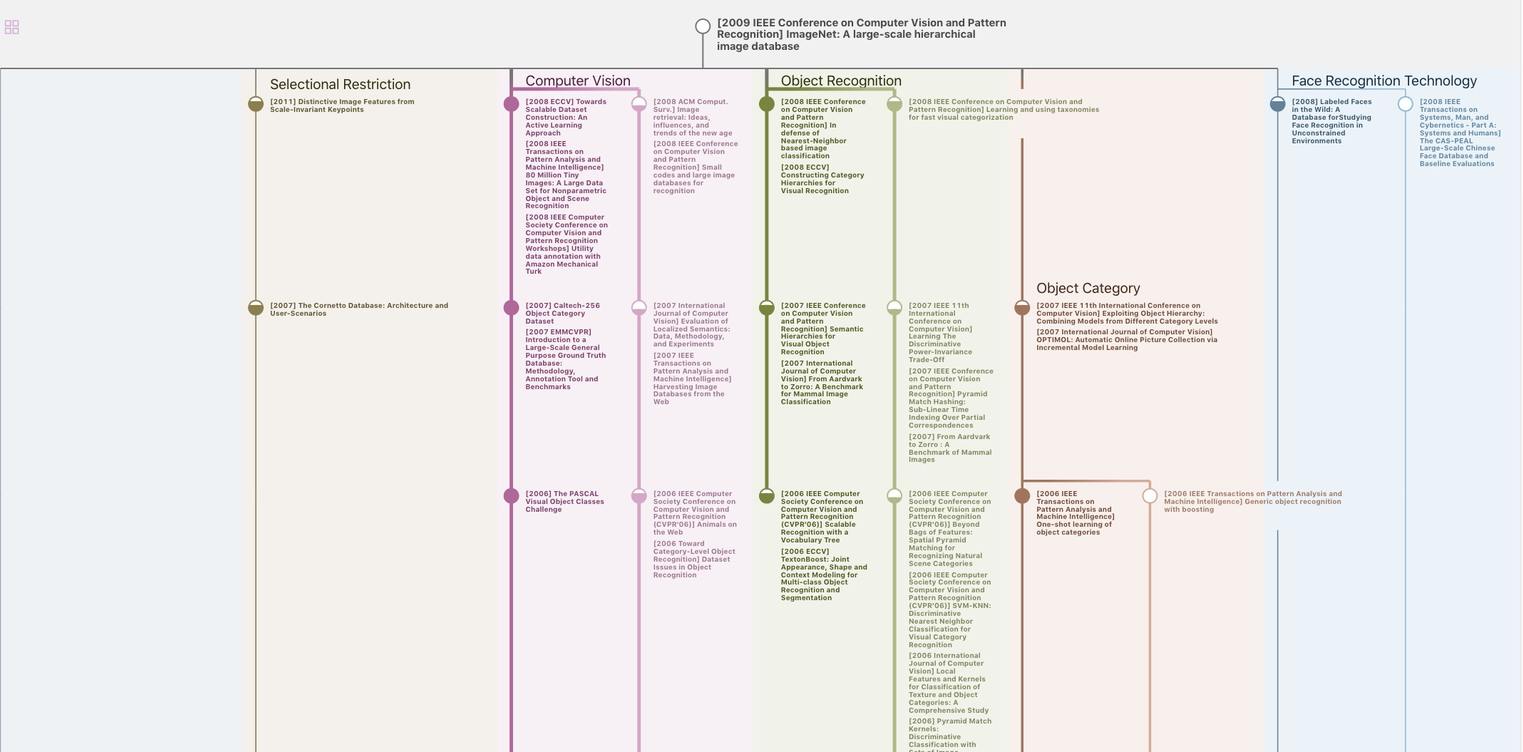
生成溯源树,研究论文发展脉络
Chat Paper
正在生成论文摘要