DRL-based admission control and resource allocation for 5G network slicing
SADHANA-ACADEMY PROCEEDINGS IN ENGINEERING SCIENCES(2023)
摘要
Network Slicing in 5G networks enables allocation and sharing of the underlying network resources of an infrastructure provider (INP) among multiple tenants of the INP. Each tenant generates slice requests specifying the resources required and the INP collects revenue from the tenants for hosting the admitted slices. In this paper, we have used the Prioritised Experience Replay-based Deep Q-Network with N step return (N-PERDQN) Reinforcement learning (RL) approach to solve the slice admission control and associated resource allocation problem, in a dynamic environment. The slices are classified as elastic and inelastic , with flexible and rigid service guarantee requirements respectively. We have also used Long Short-Term Memory (LSTM) to predict the admitted elastic slices’ future resource requirements to share resources among elastic slices more efficiently. This enables the INP to accept more slice requests to increase its revenue while maximizing system utilization. The system has been modeled using the SimPy discrete-event simulator and TensorFlow machine learning. Performance metrics studied include INP revenue generated, class-specific slice acceptance rate and resource utilization were studied on Materna data center trace. The proposed N-PERDQN approach performs better than other RL and heuristic methods by up to 10% on average, in terms of generating INP revenue. Also, N-PERDQN+LSTM accepts around 6% more slice requests than N-PERDQN without LSTM. We have also tested the performance of the proposed algorithm with an offline algorithm, that has complete set of incoming slice request information available beforehand. The proposed algorithm is able to achieve close to 82% to 86% in terms of revenue gain of the offline algorithm. The proposed mechanism was also studied using the Alibaba data center trace and similar improvements were observed.
更多查看译文
关键词
5G Network Slicing,admission control,deep reinforcement learning,prioritised experience replay,LSTM-based resource usage prediction
AI 理解论文
溯源树
样例
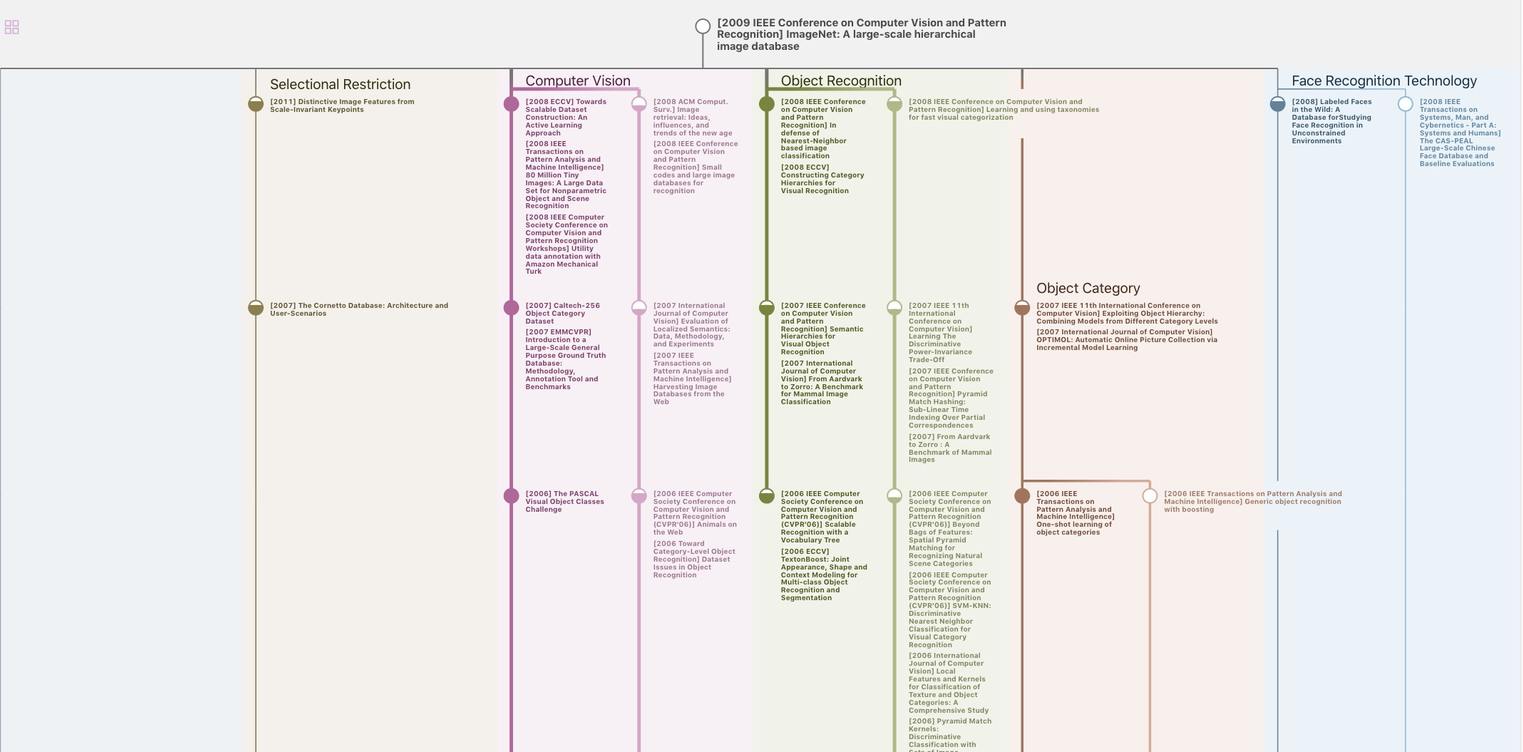
生成溯源树,研究论文发展脉络
Chat Paper
正在生成论文摘要