RDBMS to distributed in-memory data grid: A paradigm shift for improved performance
JOURNAL OF STATISTICS AND MANAGEMENT SYSTEMS(2023)
Abstract
As per available Cisco VNI report [1], global IP traffic will grow more than three times and global network device connections will be more than 28.5 billion. Availability of high-speed network and connectivity will lead to more online business opportunities to enterprises and in turn will generate large data. Sometimes these large datasets need to be processed in near real time to do some critical analysis. Traditional databases are not designed to do real time data analysis and have challenges in terms of performance and throughput. This paper describes various widely used data storage tools and frameworks currently available and how to achieve the best performance while processing large datasets using in memory data grid. It briefly covers the evolution of in-memory data grid from traditional relational database management systems. It also provides a high-level feature comparison across available in-memory database solutions and how they provide the best of solution for processing real time data beyond Hadoop referencing use cases like fraud detection and genetic data processing. It also emphasizes on leveraging power of GPU alongside CPU to achieve high performance and throughput while processing large data with multiple iterations.
MoreTranslated text
Key words
grid,improved performance,in-memory
AI Read Science
Must-Reading Tree
Example
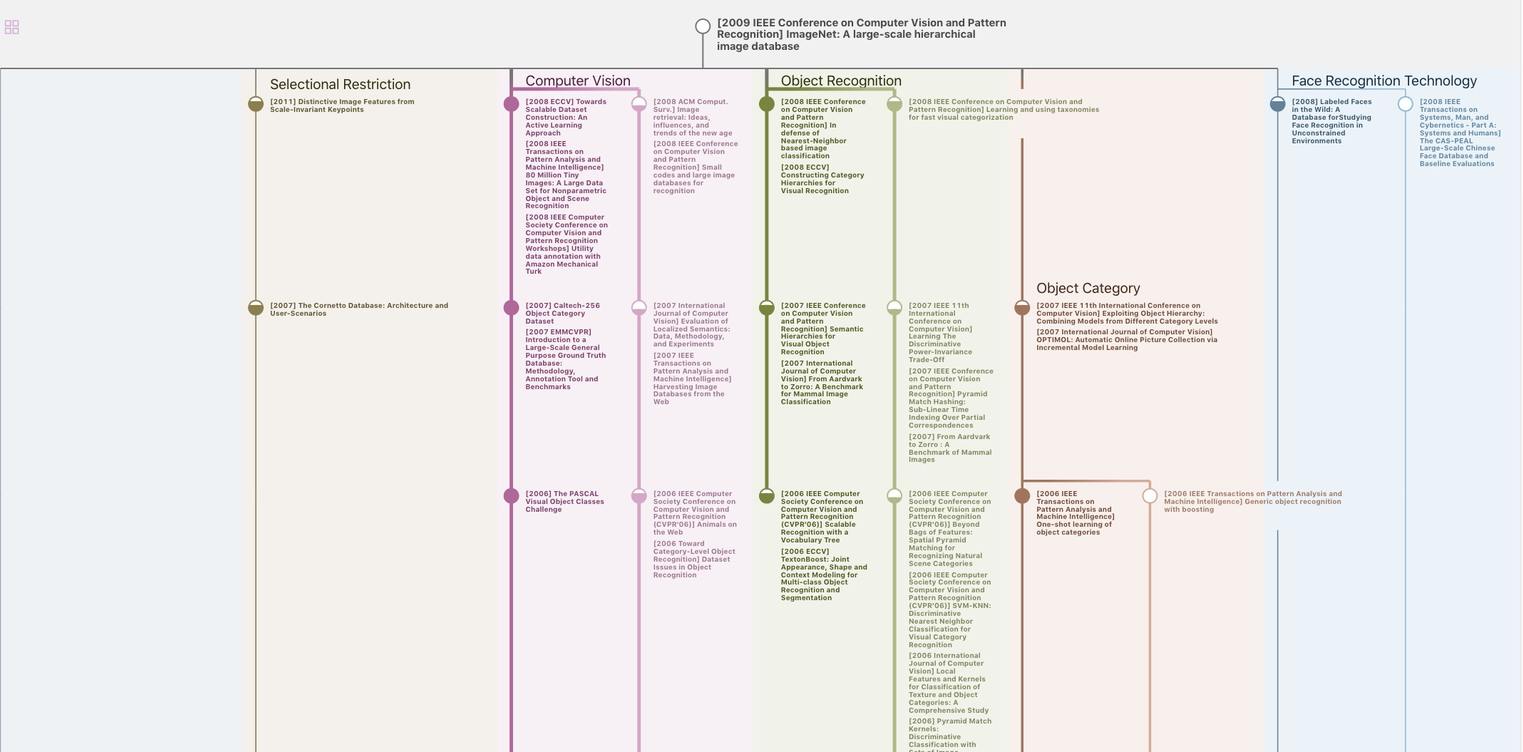
Generate MRT to find the research sequence of this paper
Chat Paper
Summary is being generated by the instructions you defined