Modeling tropospheric zenith wet delays in the Chinese mainland based on machine learning
GPS SOLUTIONS(2023)
摘要
In this study, the tropospheric zenith wet delay (ZWD) modeling was realized based on the regression analysis of 7 years (2013–2019) of radiosonde data at 182 sites in the Chinese mainland and the surroundings through two machine learning (ML) approaches including back-propagation neural network (BPNN) and random forest (RF). Furthermore, the forecasting performance of the ML-based models and other formulae for ZWD calculation was assessed by the sounding profiles at the discrete sites for the year 2020. Our results show that RF-based and BPNN-based blind ZWD models obtain an overall accuracy of 4.7 cm in the Chinese mainland, which is slightly superior to that of the empirical Global Pressure and Temperature (GPT3) model (RMS: 4.8 cm). On the other hand, when sites access meteorological data, the ML-based models with meteorological parameterization (CZWD-C and CZWD-F) can achieve overall accuracies of 3.5 cm and 3.7 cm in the Chinese mainland, respectively. Their accuracies improved by 19% and 16% compared to the Saastamoinen model and improved by 12% and 9% in contrast to the Askne and Nordius formula. Moreover, ZWD forecasting accuracy in the Chinese mainland is significantly improved by introducing surface meteorological parameters into the functional formulation, in particular with the surface water vapor pressure. Furthermore, compared with the GPT3, the ML-based models with meteorological parameterization can improve ZWD forecasting accuracy across mainland China, especially in regions with monsoon climate patterns.
更多查看译文
关键词
Tropospheric zenith wet delay,Machine learning,Back-propagation neural network,Random forest,Chinese mainland
AI 理解论文
溯源树
样例
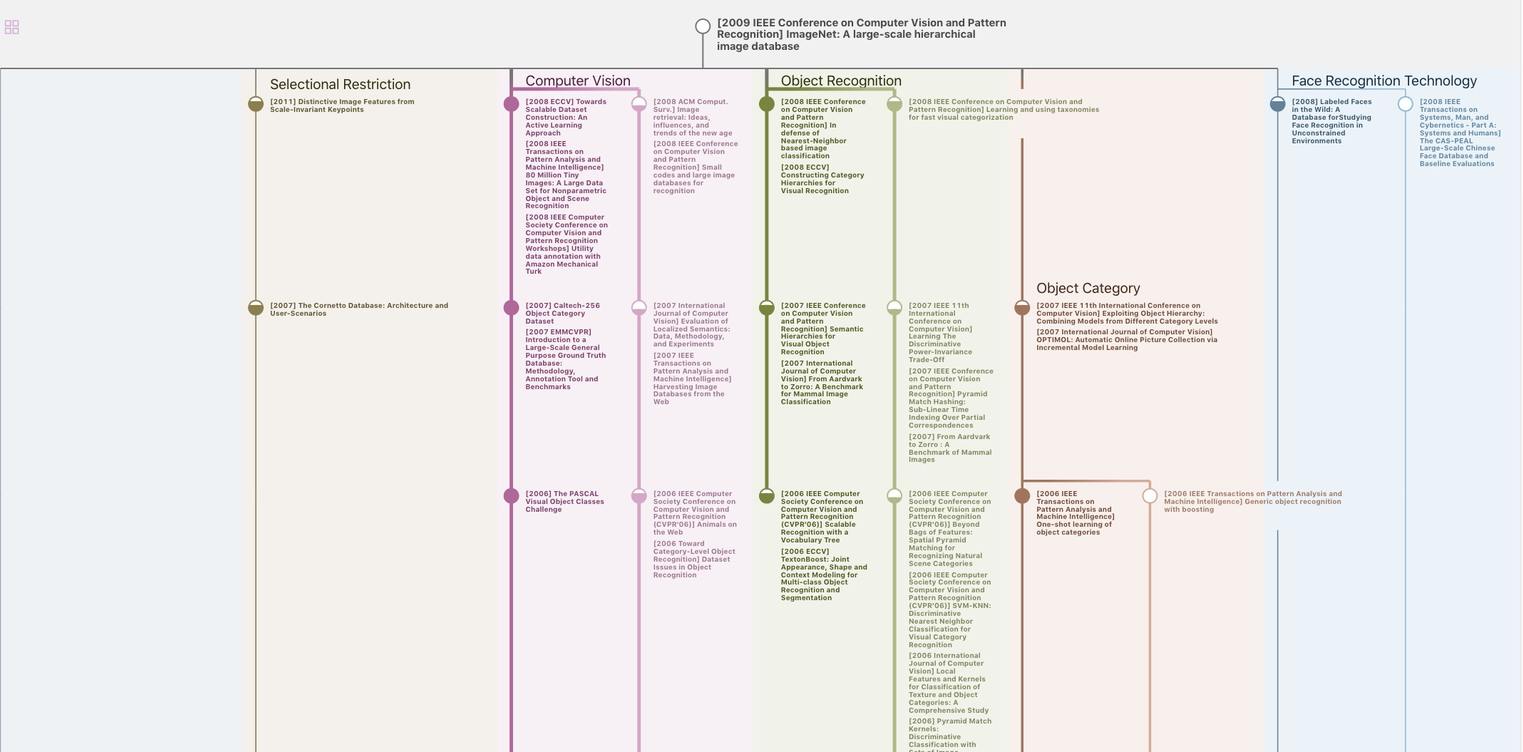
生成溯源树,研究论文发展脉络
Chat Paper
正在生成论文摘要