Short-term rockburst prediction in underground project: insights from an explainable and interpretable ensemble learning model
ACTA GEOTECHNICA(2023)
摘要
Rockburst is a frequent challenge during tunnel and other underground construction and is an extreme rock damage phenomenon. Therefore, it is very crucial to accurately estimate the damage potential of rockburst events. Microseismic (MS) monitoring can be used to obtain the relevant MS parameters for short-term rockburst prediction in real time that reflect the evolution of short-term rockburst. In this study, short-term rockburst potential data containing 7 MS parameters (cumulative number of events, cumulative released energy, cumulative apparent volume, event rate, energy rate, apparent volume rate, and incubation time) and 91 rockburst events (none rockburst, low rockburst, moderate rockburst, and high rockburst) were collected from the Jinping Hydropower Station diversion tunnel project in China. The objective of this paper is to propose an ensemble learning (EML) model based on the LévyFlight-Jaya optimization (LFJaya) and fivefold cross-validation (CV) method to achieve an accurate prediction of short-term rockburst damage potential using MS information. The EML consists of light gradient boosting machine (LGBM), extreme gradient boosting (XGBoost), random forest (RF), support vector machine (SVM), and logistic regression (LR), with seven MS parameters as the EML inputs and four rockburst levels as target variables. 70% and 30% of the cases were randomly selected for training and testing, respectively. Five metrics (accuracy, kappa, precision, recall, and F1-score) and nonparametric statistical tests were used to evaluate the performance of the model. It can be observed from the results of this study that the proposed EML has a higher test accuracy (89.29%) than the multiple base classifiers used in the study. With the use of the ensemble model, the decision boundary becomes more precise and overfitting is significantly improved. Additionally, the internal decision-making process of EML was elucidated through an analysis of the model parameters using SHapley Additive exPlanations (SHAP) and Local Interpretable Model-agnostic Explanations (LIME). It was discovered that the cumulative released energy, the number of MS events, and the cumulative apparent volume (which reflects the number and strength of rock fractures) exert a significant influence on the prediction of short-term rockburst potential. Finally, developed graphical user interface (GUI) accurately predicted six instances of rockburst in the deeply buried tunnel of Jinping. Verification results indicated that the proposed EML exhibits strong generalization and can effectively utilize MS information to achieve precise short-term rockburst potential predictions.
更多查看译文
关键词
Ensemble learning,Graphical user interface,Lévy-Jaya,LIME,Model interpretation,SHAP value,Short-term rockburst
AI 理解论文
溯源树
样例
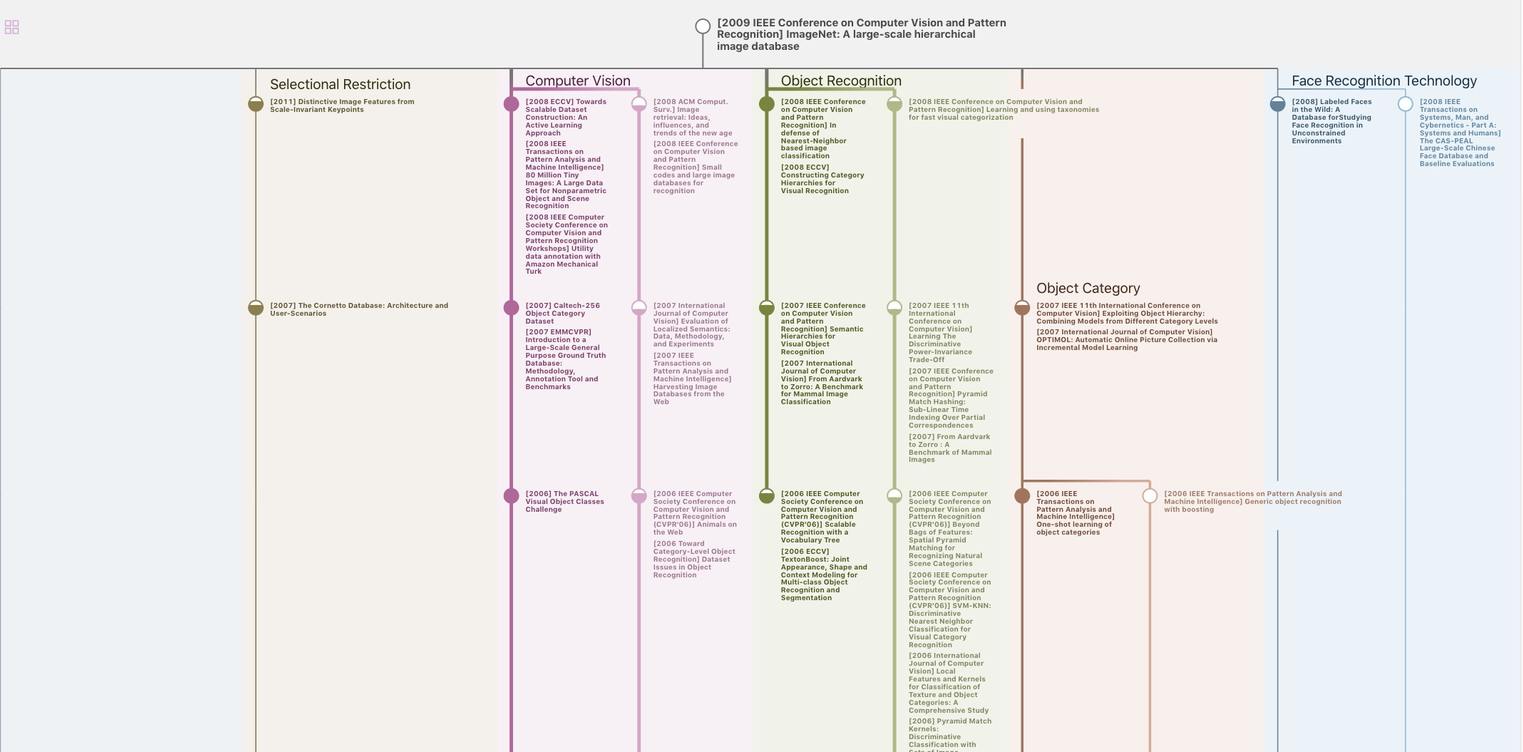
生成溯源树,研究论文发展脉络
Chat Paper
正在生成论文摘要