A Cross-Domain Generative Data Augmentation Framework for Aspect-Based Sentiment Analysis
ELECTRONICS(2023)
摘要
Aspect-based sentiment analysis (ABSA) is a crucial fine-grained sentiment analysis task that aims to determine sentiment polarity in a specific aspect term. Recent research has advanced prediction accuracy by pre-training models on ABSA tasks. However, due to the lack of fine-grained data, those models cannot be trained effectively. In this paper, we propose the cross-domain generative data augmentation framework (CDGDA) that utilizes a generation model to produce in-domain, fine-grained sentences by learning from similar, coarse-grained datasets out-of-domain. To generate fine-grained sentences, we guide the generation model using two prompt methods: the aspect replacement and the aspect-sentiment pair replacement. We also refine the quality of generated sentences by an entropy minimization filter. Experimental results on three public datasets show that our framework outperforms most baseline methods and other data augmentation methods, thereby demonstrating its efficacy.
更多查看译文
关键词
ABSA, data augmentation, cross-domain, generation model
AI 理解论文
溯源树
样例
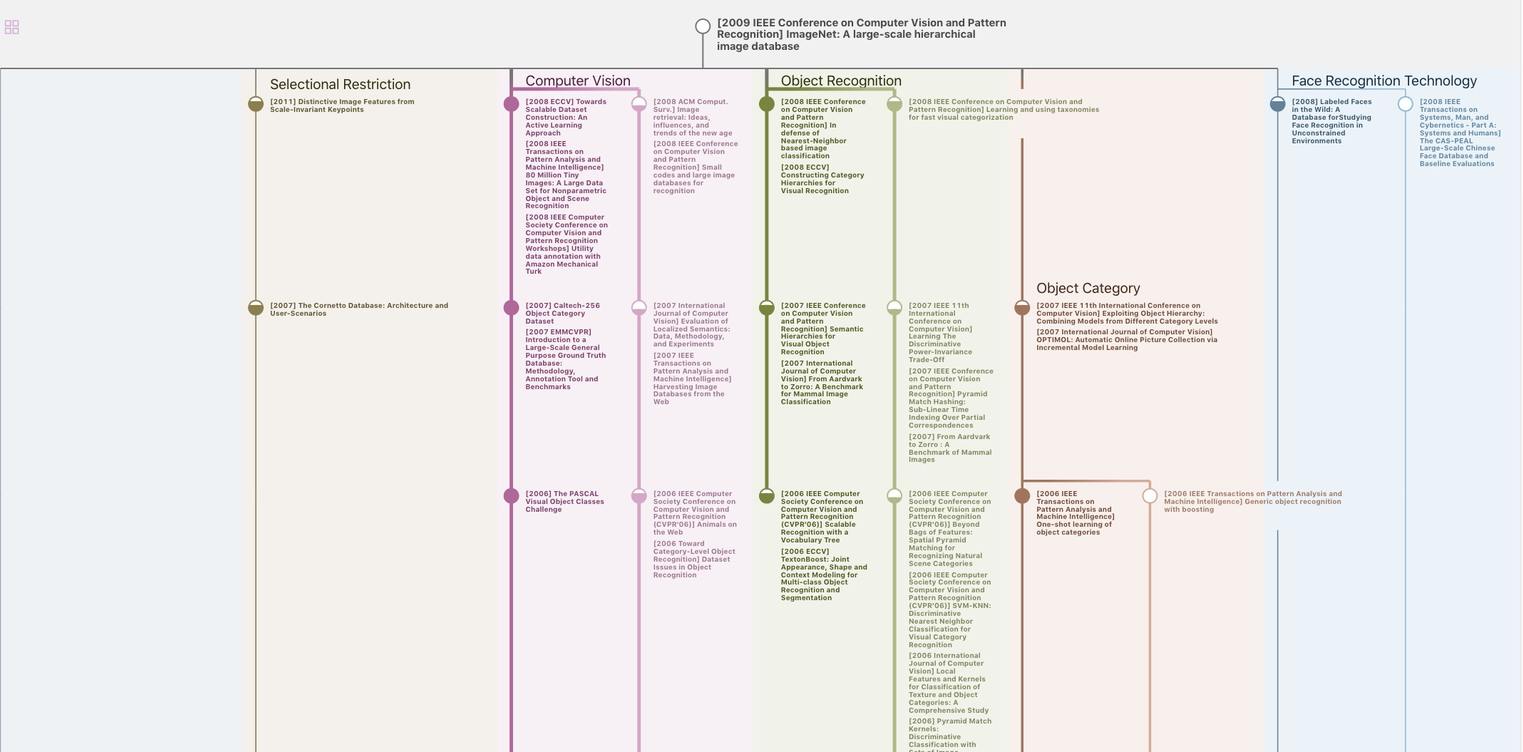
生成溯源树,研究论文发展脉络
Chat Paper
正在生成论文摘要