Generalized nonconvex regularization for tensor RPCA and its applications in visual inpainting
APPLIED INTELLIGENCE(2023)
摘要
a demonstrated and foremost approach of extracting the key features from corrupted observations, tensor robust principal component analysis has been considered in various fields of data processing related to tensors. It is usually modeled as a low-rank and sparse tensor decomposition problem and can be solved by minimizing a simple convex program. However, convex optimization methods often fail to deeply explore the rank and sparsity of tensors, which leads to suboptimality. Based on tensor singular value decomposition, in this work, we introduce generalized nonconvex regularizers accommodating most popular nonconvex (and possibly nonsmooth) surrogate functions to be used as effective approximations of the tensor rank function and ℓ _0 -norm. The established unified frame equips universality for a large group of nonconvex surrogate functions. Moreover, we consider tube-wise sparse noise in addition to entry-wise sparse noise, which provides a better way of handling structured corrupted observations arising from practical issues. We further develop an efficient algorithm with convergence guarantees to implement generalized nonconvex optimization based upon the alternating direction method of multipliers. The satisfactory performance results of the proposed method are verified by simulations and visual inpainting applications. Graphic abstract
更多查看译文
关键词
generalized nonconvex regularization,tensor rpca
AI 理解论文
溯源树
样例
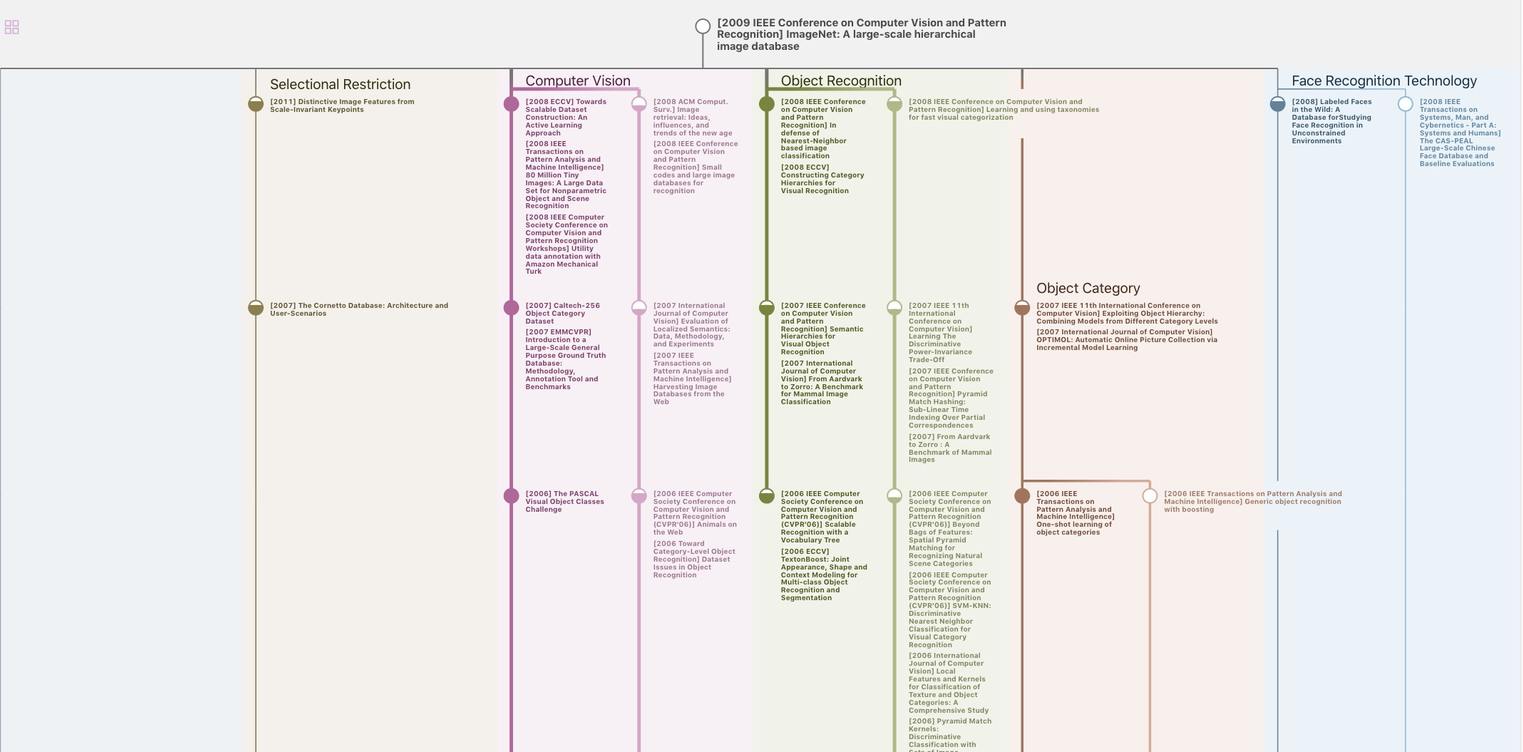
生成溯源树,研究论文发展脉络
Chat Paper
正在生成论文摘要