Research on the Predictive Algorithm of Wear Degree Based on Adaptive Feature Learning
APPLIED SCIENCES-BASEL(2023)
摘要
In the prediction and modeling analysis of wear degree in the field of industrial parts processing, there are problems such as poor prediction ability for long sequence data and low sensitivity of output feedback to changes in input signals. In this paper, a combined prediction model is proposed that integrates dual attention mechanisms and self-regressive correction. Firstly, pre-processing is performed on the collected wear data to eliminate noise and aberrant mutation data. Then, the feature attention mechanism is introduced to analyze the input data sequence, and the weights of each feature under the temporal condition are set based on the contribution of the prediction results, thereby obtaining the LSTM hidden state at the current time. Subsequently, the temporal attention mechanism is introduced to perform a weighted calculation of the hidden state information, analyze the correlation of long-term sequential wear data, and decode and output the analysis results. Finally, the ARIMA model is used to perform linear correction on the predicted results to improve the accuracy of wear degree prediction. The proposed model is compared and analyzed with the models that are highly related in recent research on real-world wear degree datasets. The experimental results show that the improved model has a better ability to improve the corresponding problems and has a significant increase in prediction accuracy.
更多查看译文
关键词
attention mechanism,time series,ARIMA model,combined prediction
AI 理解论文
溯源树
样例
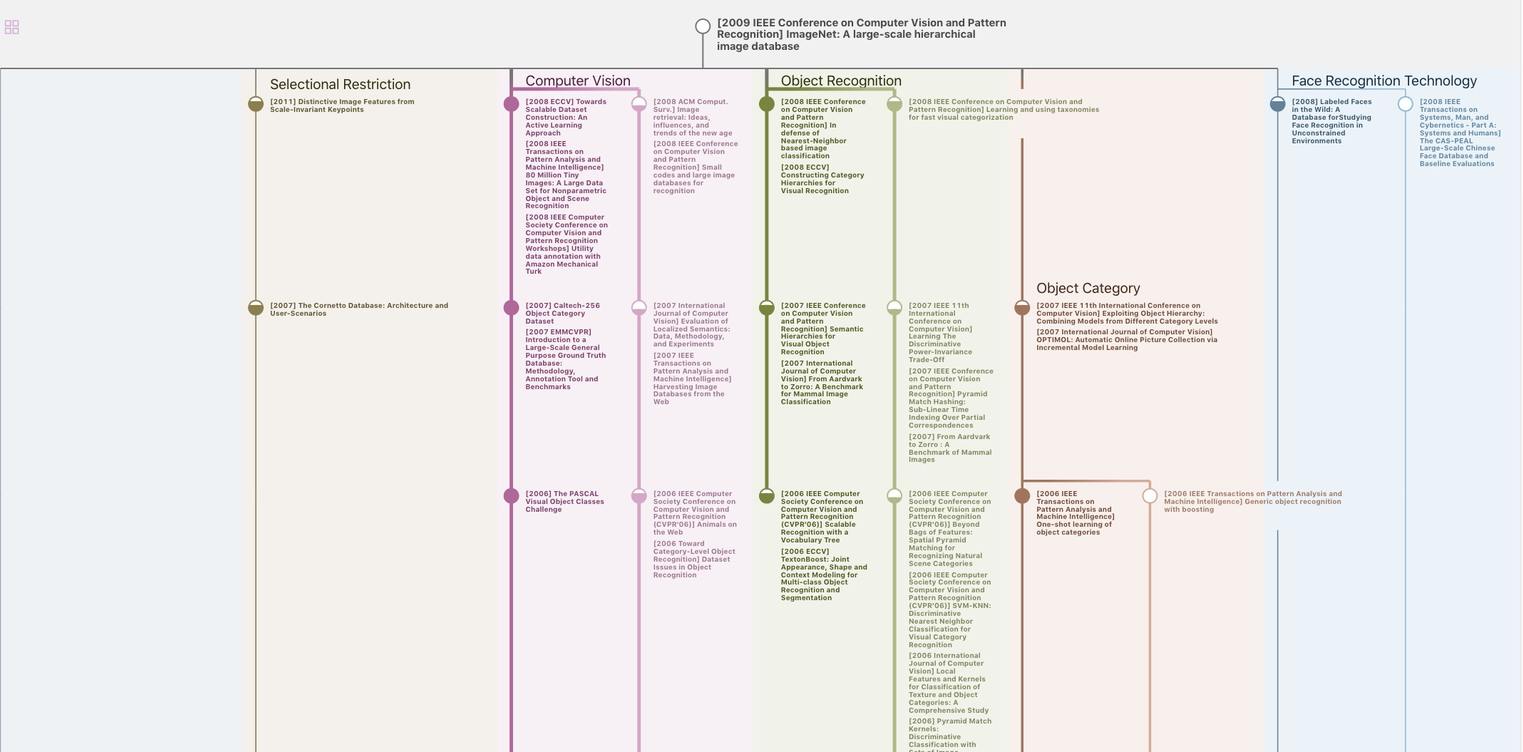
生成溯源树,研究论文发展脉络
Chat Paper
正在生成论文摘要