Machine learning-assisted investigation of the impact of lithium-ion de-embedding on the thermal conductivity of LiFePO4
APPLIED PHYSICS LETTERS(2023)
摘要
In this study, we employ a machine-learning potential approach based on first-principles calculations combined with the Boltzmann transport theory to investigate the impact of lithium-ion de-embedding on the thermal conductivity of LiFePO4, with the aim of enhancing heat dissipation in lithium-ion batteries. The findings reveal a significant decrease in thermal conductivity with increasing lithium-ion concentration due to the decrease in phonon lifetime. Moreover, removal of lithium ions from different sites at a given lithium-ion concentration leads to distinct thermal conductivities, attributed to varying anharmonicity arising from differences in bond lengths and bond strengths of the Fe-O bonds. Our work contributes to a fundamental understanding of the thermal transport properties of lithium iron phosphate batteries, emphasizing the pivotal role of lithium-ion detachment and intercalation in the thermal management of electrochemical energy storage devices.
更多查看译文
关键词
thermal conductivity,learning-assisted,lithium-ion,de-embedding
AI 理解论文
溯源树
样例
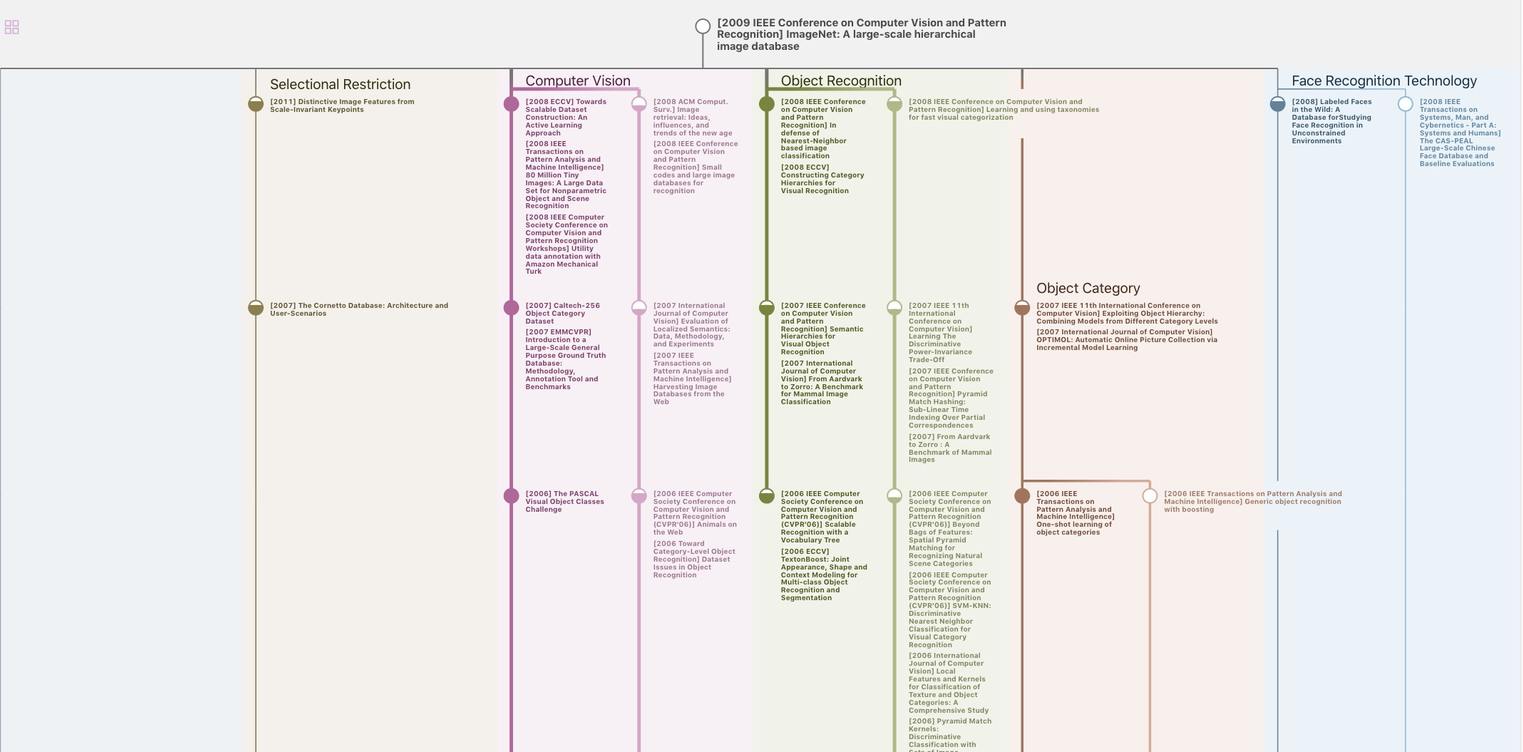
生成溯源树,研究论文发展脉络
Chat Paper
正在生成论文摘要