Deep-learning-based 3D Fault Detection for Carbon Capture and Storage
GEOPHYSICS(2023)
摘要
Fault analysis plays an important role in determining the location of potential CO 2 storage sites in which long-term storage feasibility is critical. The presence of faults and fracture zones can potentially lead to the gradual migration of CO 2 from the storage reservoir to the atmosphere through the overburden strata. Traditional methods such as manual picking of faults are time-consuming and labor-intensive tasks, which can be costly for the CO 2 storage site assessment process. To help address this concern, we develop a process for automating fault picking using deep neural networks. We implement two different deep neural networks based on the architectures of UNet and Deeplab v3+. We train the networks using synthetic data and labels and augment them using linear transformations and elastic deformations. We implement an ensemble-based approach to improve the generalization ability of our method. We also incorporate uncertainty estimation into the framework to aid in the risk management aspect of the decision-making process. We use data sets from different regions across the globe which help us to improve the methodology, with the ultimate aim to help 3D fault detection in the Smeaheia Field, a potential site for CO 2 storage scale-up on the Horda Platform, offshore Norway.
更多查看译文
关键词
Carbon Capture,CO2 Sequestration,Seismic Data Processing
AI 理解论文
溯源树
样例
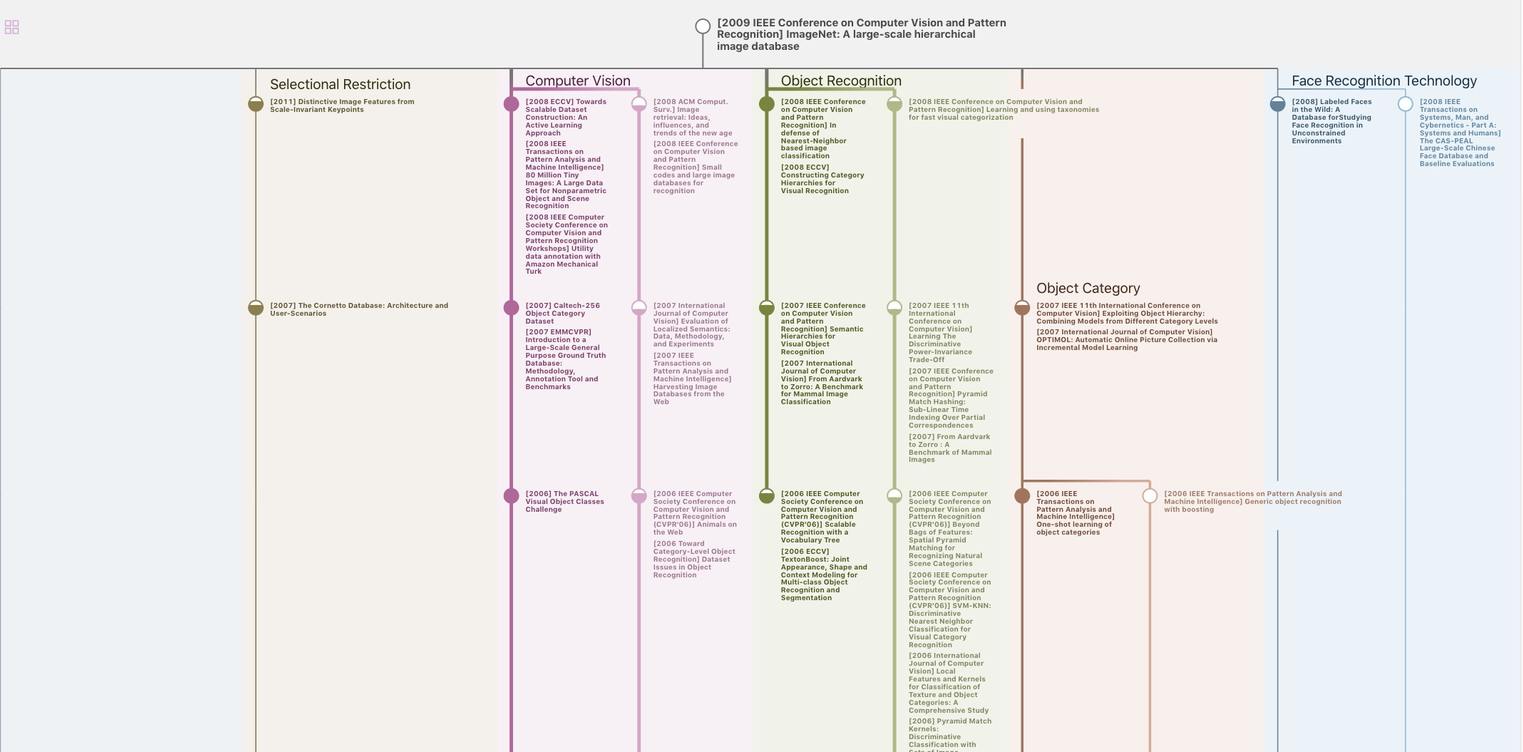
生成溯源树,研究论文发展脉络
Chat Paper
正在生成论文摘要