Cascaded neural network segmentation pipeline for automated delineation of prostate and organs at risk in male pelvic CT
MEDICAL IMAGING 2023(2023)
摘要
Delineation of the prostate and nearby organs at risk (OARs) is a fundamental step in prostate cancer radiation therapy planning. Such contouring is often done manually, which can be a time-consuming and highly variable process. To alleviate these issues, we propose a fully automated two-step deep learning approach to segment the prostate, bladder, rectum, seminal vesicles, and femoral heads from CT images. The first step localizes the organs of interest using a modified 3D UNet architecture that contains an axial cross-attention module. Final segmentations are then computed for each organ individually using organ-specifically optimized UNet-based models. A total of 275 CT images were used for model training and validation. When evaluated on a hold-out set of 15 image sets, the full pipeline achieved mean dice similarity coefficients (DSC) and 95% Hausdorff distances (95HD, in mm) of 0.866 +/- 0.034 and 4.46 +/- 1.02 (prostate), 0.957 +/- 0.014 and 2.91 +/- 0.289 (bladder), 0.853 +/- 0.044 and 5.10 +/- 1.87 (rectum), 0.740 +/- 0.117 and 6.72 +/- 9.46 (seminal vesicles), 0.942 +/- 0.016 and 2.85 +/- 1.04 (left femoral head), 0.942 +/- 0.018 and 3.04 +/- 1.37 (right femoral head).
更多查看译文
AI 理解论文
溯源树
样例
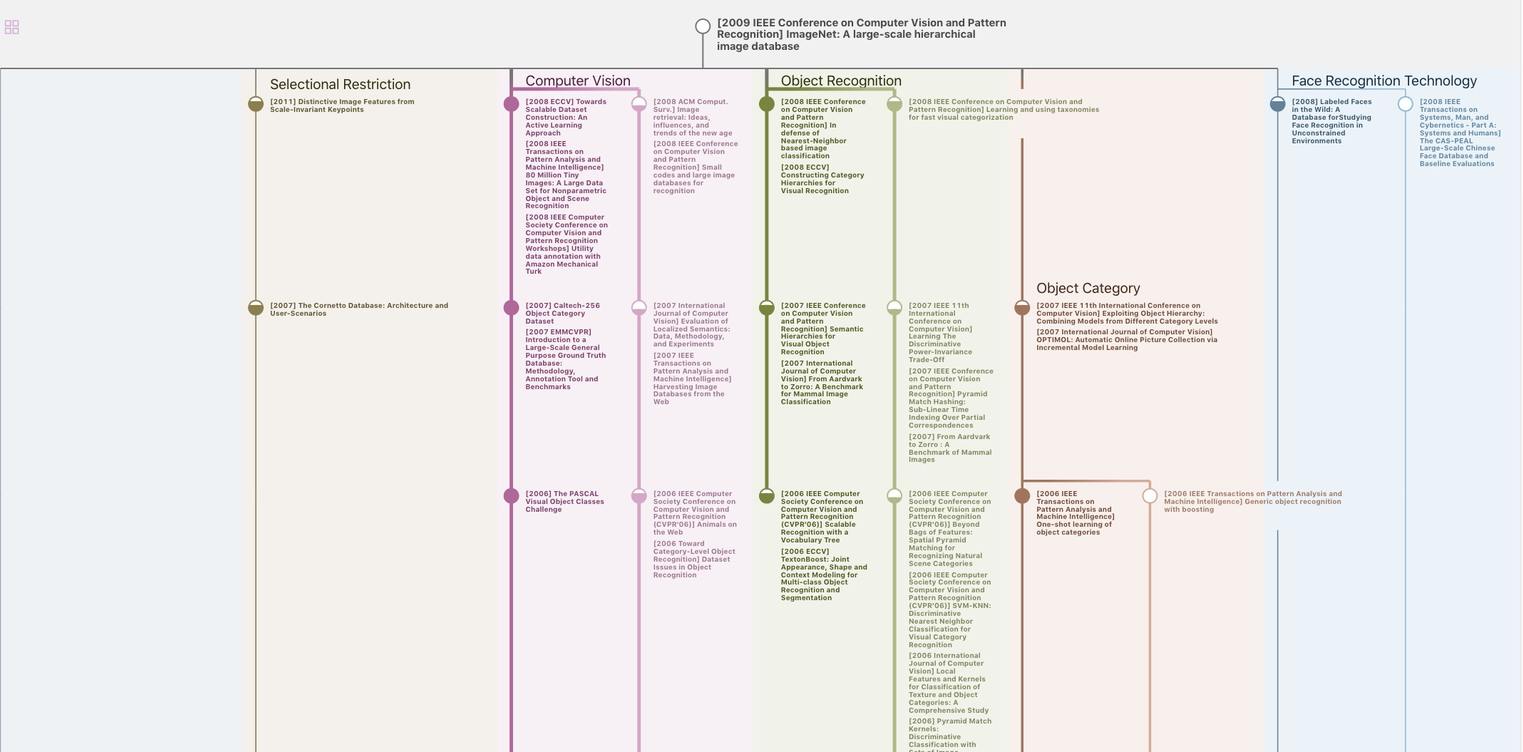
生成溯源树,研究论文发展脉络
Chat Paper
正在生成论文摘要