Monthly precipitation prediction at regional scale using deep convolutional neural networks
HYDROLOGICAL PROCESSES(2023)
摘要
Variations in monthly precipitation are associated with climate extremes having significant socio-economic and eco-environmental impacts. Knowledge of monthly precipitation information is therefore valuable for policy making. Extensive research has been conducted on dynamic prediction using state-of-the-art coupled climate models. However, the skilful prediction of monthly precipitation with dynamical models remains a challenge. With the development of machine learning tools, statistical predictions show comparable performance with dynamic models, but they are limited to at-site monthly prediction, due to the lack of ability for processing spatially connected geophysical data. To improve monthly precipitation forecasting and provide regional forecasts, we propose a model termed UNet-RegPre based on convolutional neural network and U-net architecture. The model shows comparable prediction skills with recurrent state-of-the-art dynamic forecasts (CFSv2), and has the capability to capture spatiotemporal patterns of precipitation and reproduce the main process of representative droughts. The key precursors for rainfall development identified by UNet-RegPre shows that the constructed model can detect the climatic connection between rainfall in eastern China and summer monsoon, showing the potential to advance hydrometeorological understanding with a deep learning-based model. These results suggest that the proposed model can be a potential tool for precipitation prediction.
更多查看译文
关键词
monthly precipitation prediction,deep convolutional neural networks,neural networks
AI 理解论文
溯源树
样例
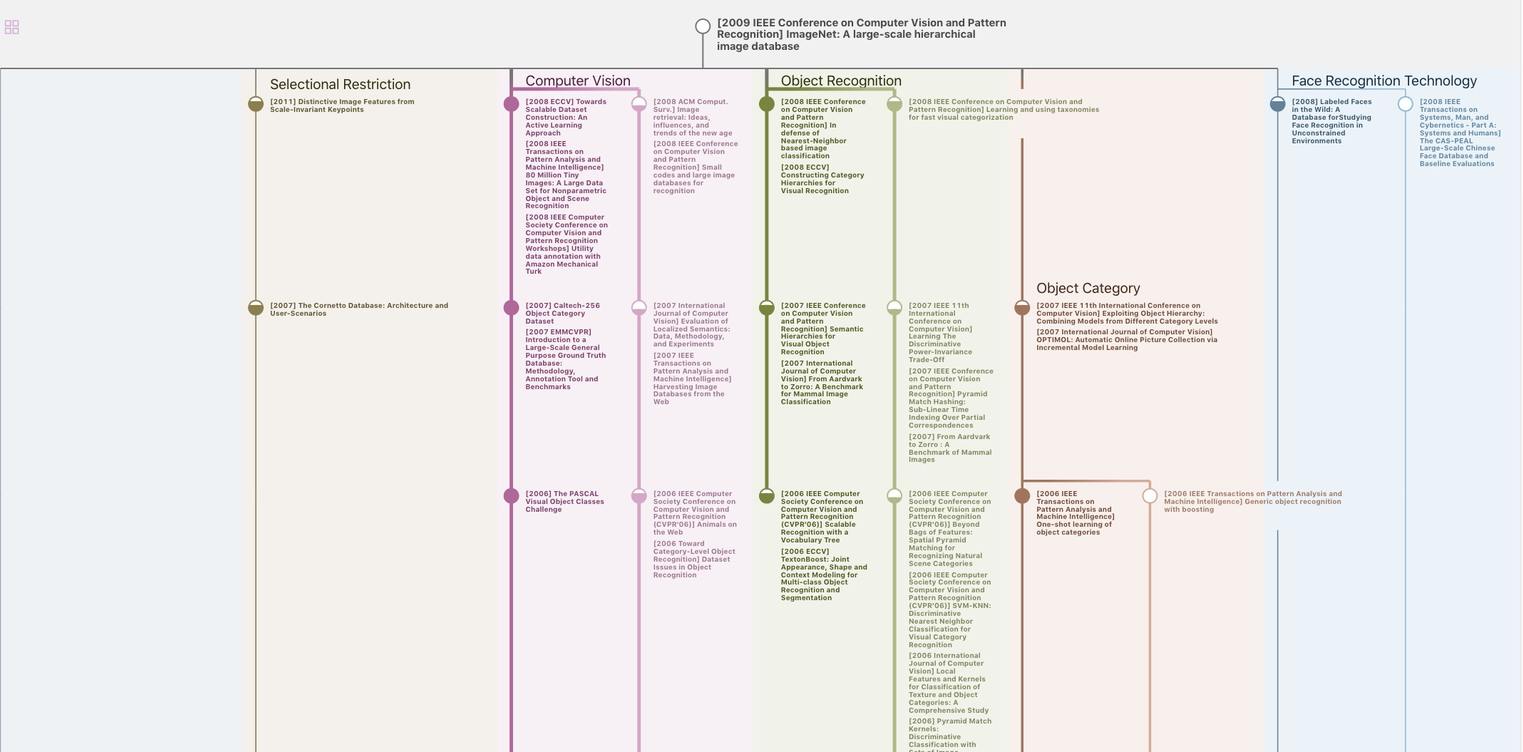
生成溯源树,研究论文发展脉络
Chat Paper
正在生成论文摘要