Comparison and Enhancement of Machine Learning Algorithms for Wind Turbine Output Prediction with Insufficient Data
ENERGIES(2023)
摘要
As the penetration of renewable energy sources into a power system increases, the significance of precise short-term forecasts for wind power generation becomes paramount. However, the erratic and non-periodic nature of wind poses challenges in accurately predicting the output. This paper presents a comprehensive investigation into forecasting wind power generation for the following day, using three machine learning models: long short-term memory (LSTM), convolutional neural network-bidirectional LSTM (CNN-biLSTM), and light gradient boosting machine (LGBM). In addition, this paper proposes a method to improve the prediction performance of LGBM by separating data according to the distribution of features, and training and testing each separated dataset with a distinct model. This study includes a comparative analysis of the performance of the proposed models in predicting wind turbine output, offering valuable insights into their respective efficiencies. The results of this investigation were analyzed for two geographically distinct wind farms (Korea and the UK). The findings of this study are expected to facilitate the selection of efficient prediction models within the forecast accuracy auxiliary service market and assist grid operators in ensuring reliable power supply for the grid.
更多查看译文
关键词
renewable energy, wind power forecasting, wind turbine generator, artificial intelligence, CNN, LSTM, LGBM
AI 理解论文
溯源树
样例
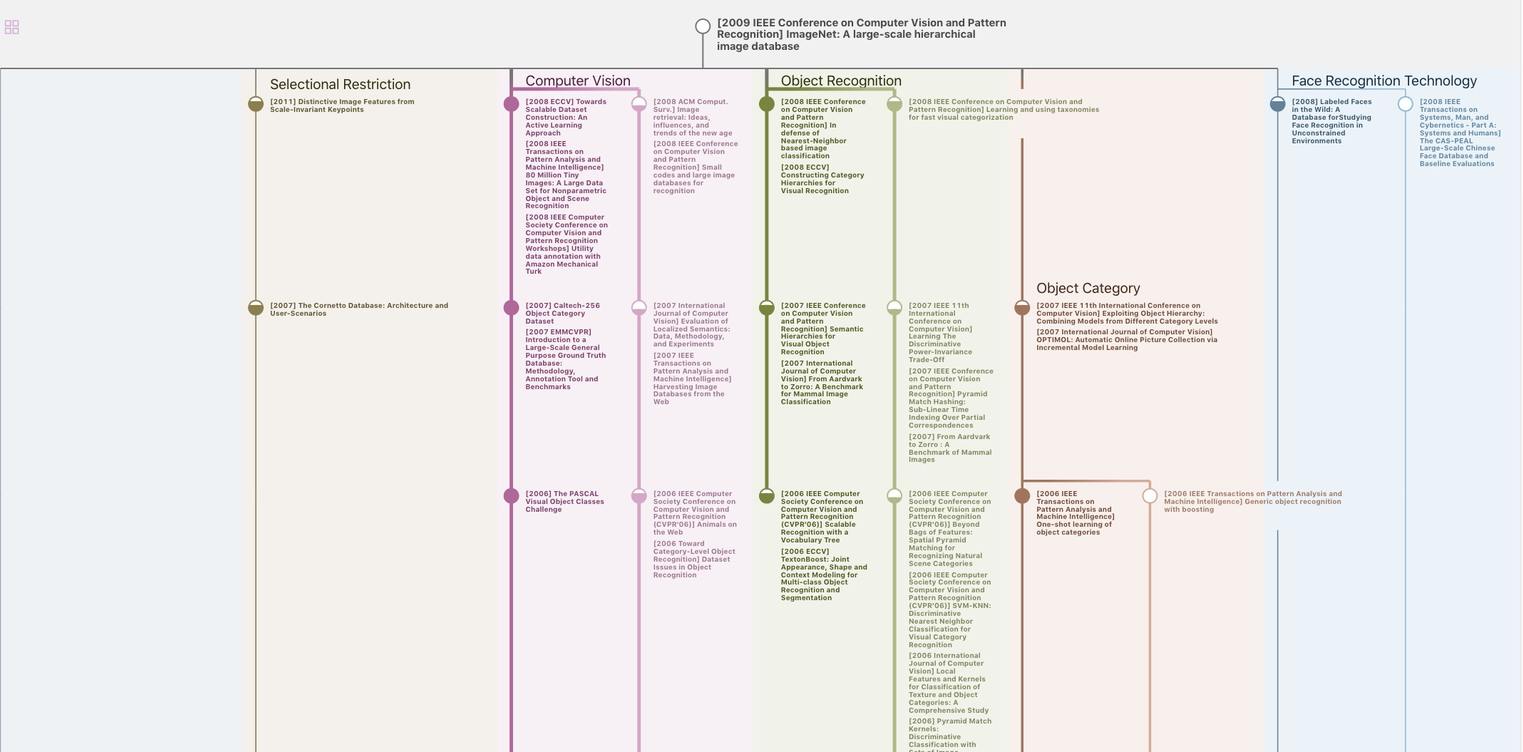
生成溯源树,研究论文发展脉络
Chat Paper
正在生成论文摘要