A machine learning algorithm for reliably predicting active galactic nucleus absorbing column densities
ASTRONOMY & ASTROPHYSICS(2023)
摘要
We present a new method for predicting the line-of-sight column density (N-H) values of active galactic nuclei (AGN) based on mid-infrared (MIR), soft X-ray, and hard X-ray data. We developed a multiple linear regression machine learning algorithm trained with WISE colors, Swift-BAT count rates, soft X-ray hardness ratios, and an MIR-soft X-ray flux ratio. Our algorithm was trained off 451 AGN from the Swift-BAT sample with known N-H and has the ability to accurately predict N-H values for AGN of all levels of obscuration, as evidenced by its Spearman correlation coefficient value of 0.86 and its 75% classification accuracy. This is significant as few other methods can be reliably applied to AGN with Log(N-H < 22.5). It was determined that the two soft X-ray hardness ratios and the MIR-soft X-ray flux ratio were the largest contributors toward accurate N-H determinations. We applied the algorithm to 487 AGN from the BAT 150 Month catalog with no previously measured N-H values. This algorithm will continue to contribute significantly to finding Compton-thick (CT) AGN (N-H = 10(24) cm(-2)), thus enabling us to determine the true intrinsic fraction of CT-AGN in the local Universe and their contribution to the cosmic X-ray background.
更多查看译文
关键词
infrared, galaxies, active, nuclei, X-rays, diffuse background, methods, data analysis
AI 理解论文
溯源树
样例
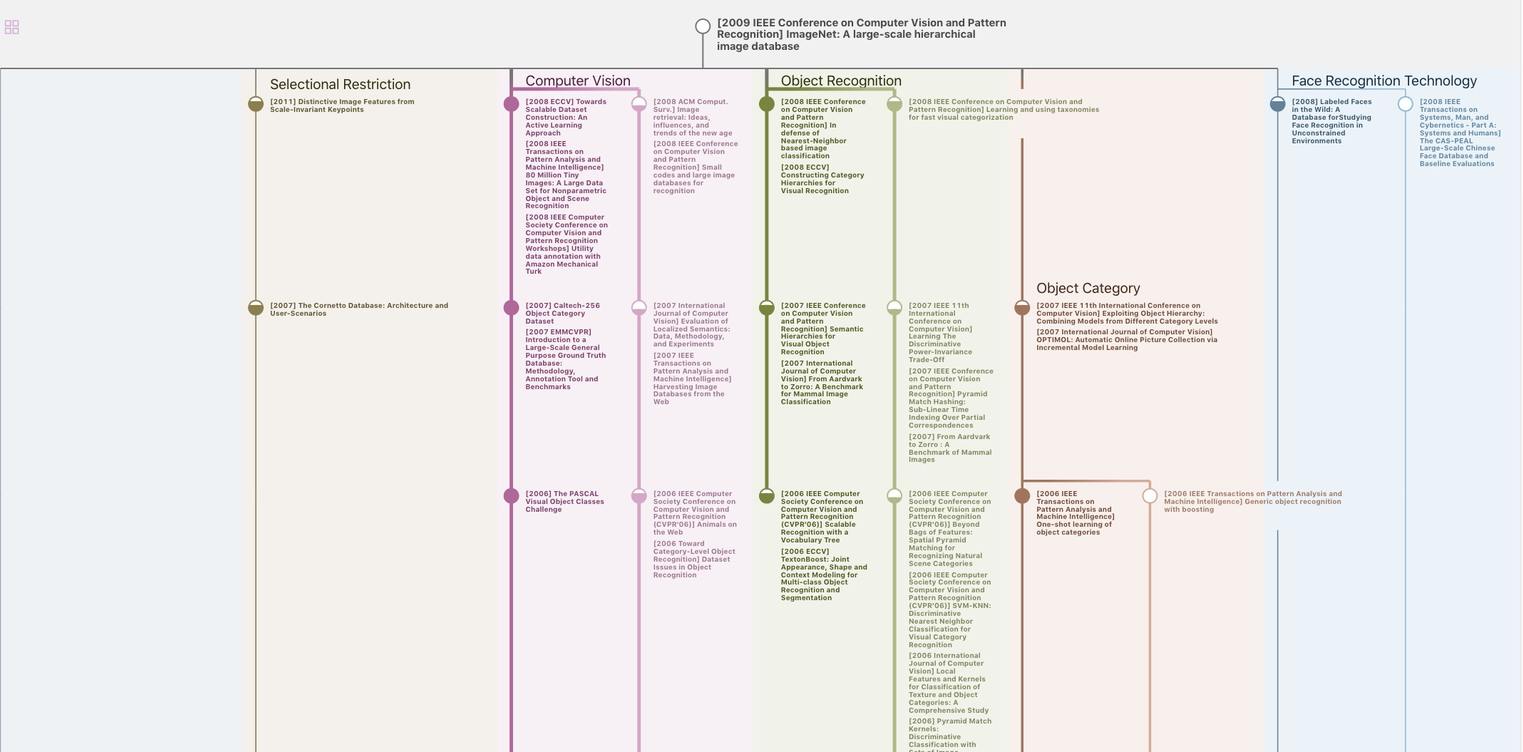
生成溯源树,研究论文发展脉络
Chat Paper
正在生成论文摘要