A Many-Objective Evolutionary Algorithm Based on Dual Selection Strategy
ENTROPY(2023)
摘要
In high-dimensional space, most multi-objective optimization algorithms encounter difficulties in solving many-objective optimization problems because they cannot balance convergence and diversity. As the number of objectives increases, the non-dominated solutions become difficult to distinguish while challenging the assessment of diversity in high-dimensional objective space. To reduce selection pressure and improve diversity, this article proposes a many-objective evolutionary algorithm based on dual selection strategy (MaOEA/DS). First, a new distance function is designed as an effective distance metric. Then, based distance function, a point crowding-degree (PC) strategy, is proposed to further enhance the algorithm's ability to distinguish superior solutions in population. Finally, a dual selection strategy is proposed. In the first selection, the individuals with the best convergence are selected from the top few individuals with good diversity in the population, focusing on population convergence. In the second selection, the PC strategy is used to further select individuals with larger crowding distance values, emphasizing population diversity. To extensively evaluate the performance of the algorithm, this paper compares the proposed algorithm with several state-of-the-art algorithms. The experimental results show that MaOEA/DS outperforms other comparison algorithms in overall performance, indicating the effectiveness of the proposed algorithm.
更多查看译文
关键词
many-objective optimization, convergence, diversity, dual selection
AI 理解论文
溯源树
样例
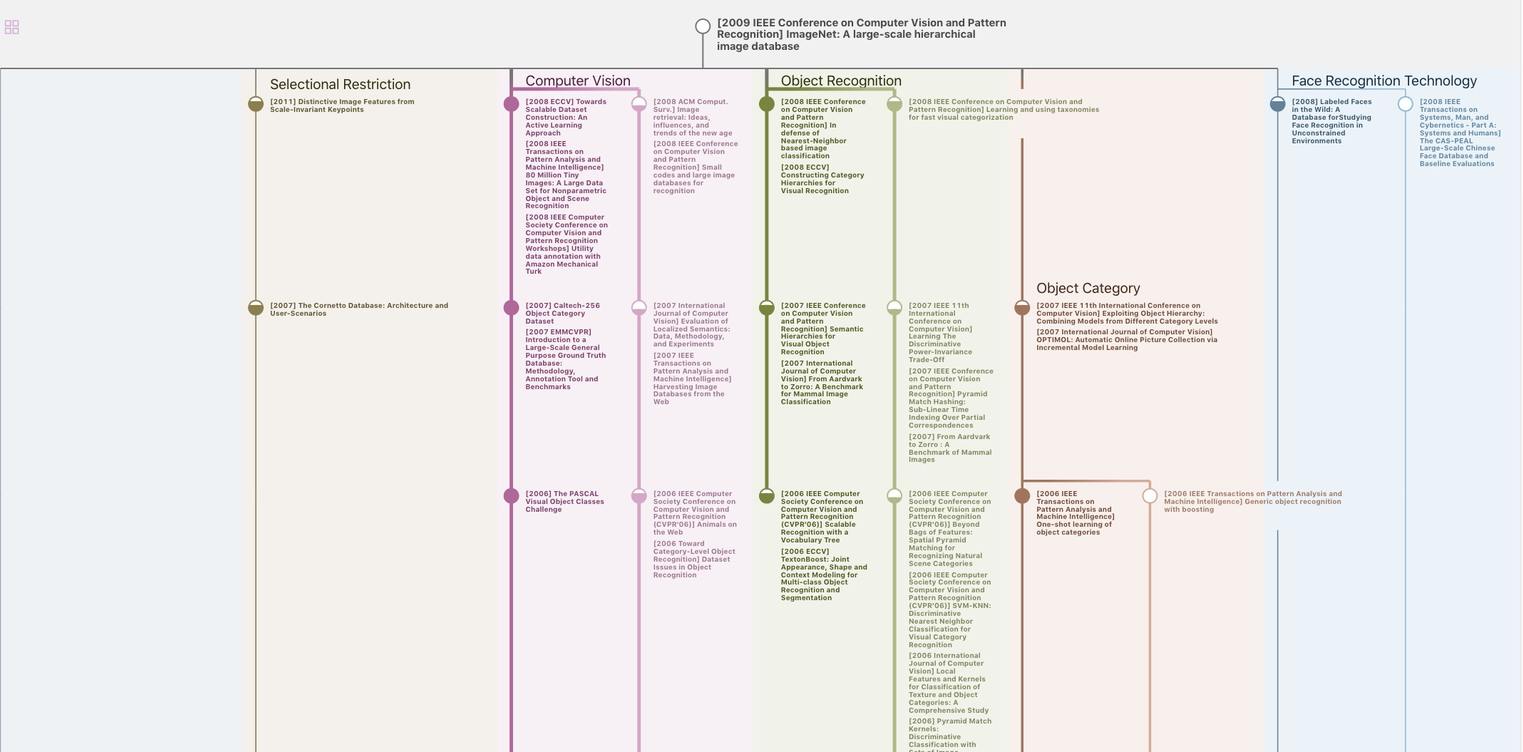
生成溯源树,研究论文发展脉络
Chat Paper
正在生成论文摘要