Improved SR-SSIM Band Selection Method Based on Band Subspace Partition
REMOTE SENSING(2023)
摘要
Scholars have performed much research on reducing the redundancy of hyperspectral data. As a measure of the similarity between hyperspectral bands, structural similarity is used in band selection methods. However, existing structural similarity methods calculate all the structural similarity between bands, which leads to excessively long runtimes for these methods. Aiming to address this problem, this paper proposes a band subspace partition method and combines it with the SR-SSIM band selection method to obtain an improved band selection method: E-SR-SSIM. E-SR-SSIM consists of two parts: band subspace partition and band subspace band selection. In the first part, the hyperspectral dataset is divided into subdatasets corresponding to a number of subspaces. In the second part, a modified SR-SSIM method is used for all subdatasets to select the most representative band in each subdataset. The Indian Pines, Salinas Kennedy Space Center and Wuhan unmanned aerial vehicle-borne hyperspectral image LongKou public datasets are used to implement the experiment. The experiment uses random forest as the supervised classifier: 10% of each category sample is randomly selected as training data, and the remaining 90% is used as test data. The evaluation indicators selected in the experiment are overall accuracy, average accuracy, kappa and recall. The experimental results show that E-SR-SSIM can effectively reduce the runtime while ensuring classification compared with SR-SSIM, and quantitative proof that the band subspace partition reduces the calculated amount of structural similarity is obtained through a mathematical analysis. The improved band subspace partition method could partition a dataset more reasonably than the original band subspace partition method.
更多查看译文
关键词
hyperspectral image,band selection method,band subspace partition,structural similarity
AI 理解论文
溯源树
样例
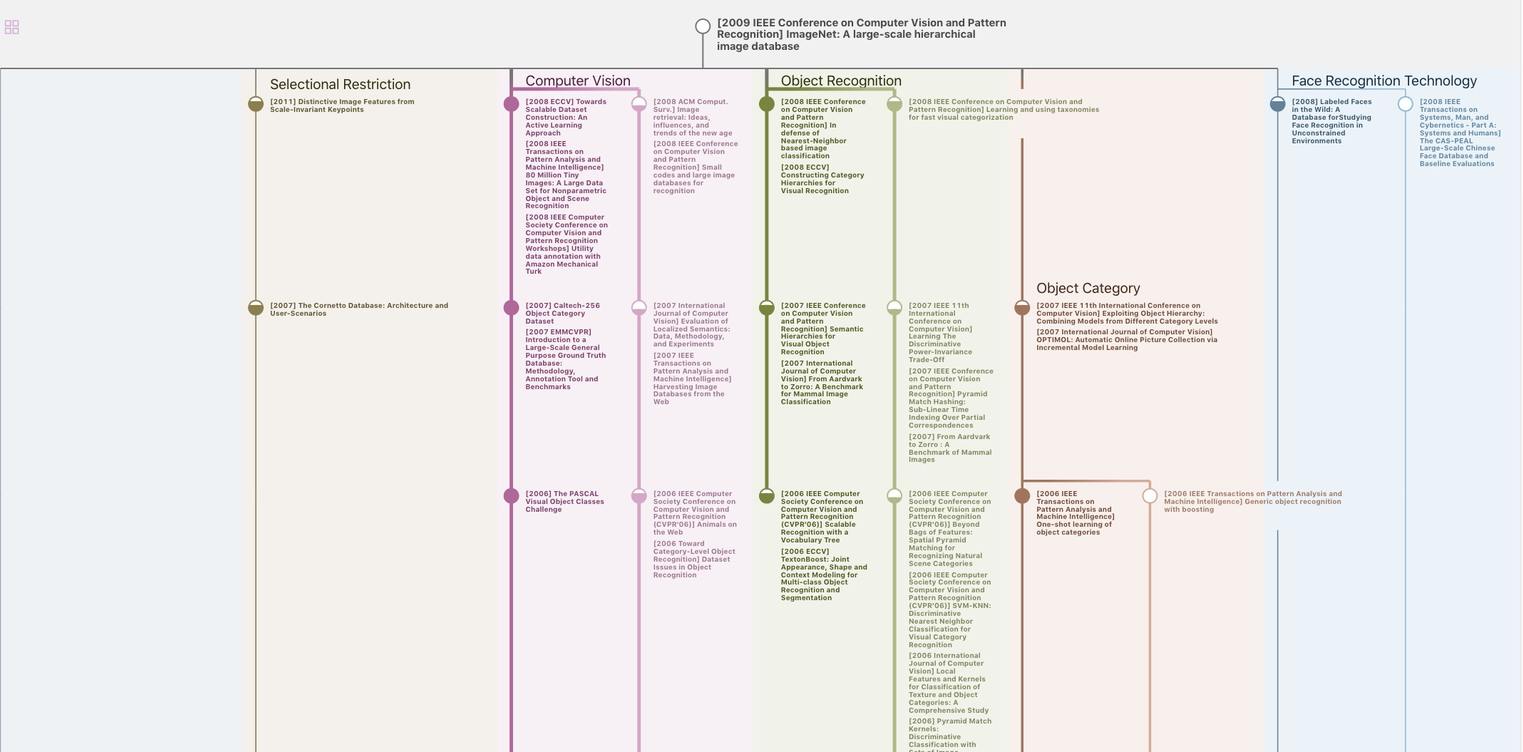
生成溯源树,研究论文发展脉络
Chat Paper
正在生成论文摘要