Seismic Data Denoising Using a Self-Supervised Deep Learning Network
MATHEMATICAL GEOSCIENCES(2024)
摘要
Deep learning (DL) techniques have recently attracted considerable attention in the field of seismic data denoising. However, most DL-based seismic denoising models require a considerable number of paired noisy-clean samples for training, which limits their application in practice. In this paper, a novel self-supervised DL scheme for noise attenuation in reflection seismic data is proposed based on the Neighbor2Neighbor strategy. The proposed method adopts a U-shaped convolutional network as the main network and incorporates regularization loss during training to improve the stability of network training, thereby constructing an end-to-end self-learning process for seismic data denoising. Specifically, a neighborhood subsampling workflow is built on single noisy seismic data to generate paired noisy images for training. Next, an unsupervised evaluation metric, grounded solely in noisy data, is adopted to quantitatively evaluate the performance of the denoising algorithm without labeled data. The application to synthetic datasets suggests that the proposed method achieves better denoising results than traditional (e.g., f-x deconvolution and wavelet analysis) and self-supervised methods, and rivals a widely used supervised DL model, the denoising convolution neural network. In real application to seismic data denoising without noise-free labels, the proposed method more effectively suppresses migration artifacts and random noise while better preserving the signal integrity compared to other methods. These improvements demonstrate that the proposed method is an independent, novel, and powerful data-driven DL scheme suitable for real seismic data denoising.
更多查看译文
关键词
Seismic data denoising,Self-supervised,Deep learning,Neighbor2Neighbor
AI 理解论文
溯源树
样例
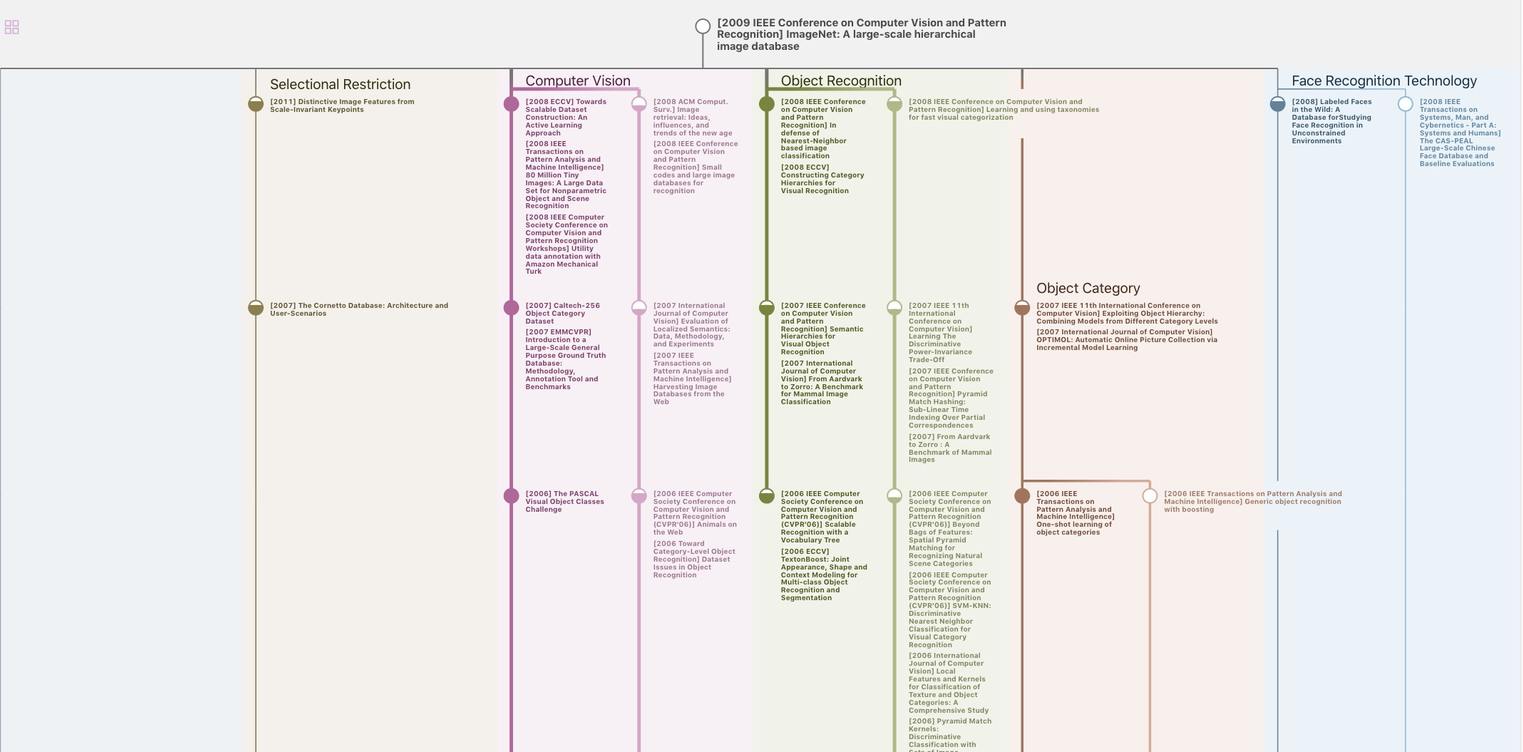
生成溯源树,研究论文发展脉络
Chat Paper
正在生成论文摘要