Machine learning techniques and multi-scale models to evaluate the impact of silicon dioxide (SiO2) and calcium oxide (CaO) in fly ash on the compressive strength of green concrete
CONSTRUCTION AND BUILDING MATERIALS(2023)
摘要
Fly ash is a by-product almost found in coal power plants; it is available worldwide. According to the hazardous impacts of cement on the environment, fly ash is known to be a suitable replacement for cement in concrete. A lot of carbon dioxide (CO2) is released during cement manufacturing. The investigations estimate that about 8 - 10% of the total CO2 emissions are maintained by cement production. Since fly ash has nearly the same chemical compounds as cement, it can be utilized as a suitable alternative to cement in concrete (green concrete). The current study analyzes the effect of the quantity of the two main components of fly ash, CaO, and SiO2, on the compressive strength of concrete modified with different fly ash content for various mix proportions. For this purpose, various concrete samples modified with fly ash were collected from the literature (236 datasets), analyzed, and modeled using four different models; Full-quadratic (FQ), Nonlinear regression (NLR), Multi-linear regression (MLR), and Artificial neural network (ANN) model to predict the compressive strength of concrete with different geometry and size of the specimens. The accuracy of the models was evaluated using correlation coefficient (R2), Mean absolute error (MAE), Root mean squared error (RMSE), Scatter Index (SI), a-20 index, and Objective function (OBJ). According to the modeling results, increasing SiO2 (%) increased the compressive strength, while increasing CaO (%) increased compressive strength only when the cement replacement with fly ash was between 52 and -100%. Based on R2, RMSE, and MAE, the ANN model was the most effective and accurate on predicting the compressive strength of concrete in different strength ranges. According to the sensitivity analysis, curing time is the most critical characteristic for predicting the compression strength of concrete using this database. The primary objective of this work is to explore and evaluate various machine learning models for predicting compressive resistance. The study emphasizes these models' development, comparison, and performance assessment, highlighting their potential to predict compressive strength accurately. The research primarily uses machine learning, leveraging algorithms and techniques to build predictive models. The focus is on harnessing the power of data-driven approaches to improve the accuracy and reliability of compressive strength predictions.
更多查看译文
关键词
concrete,fly ash,compressive strength,calcium dioxide,sio2,multi-scale
AI 理解论文
溯源树
样例
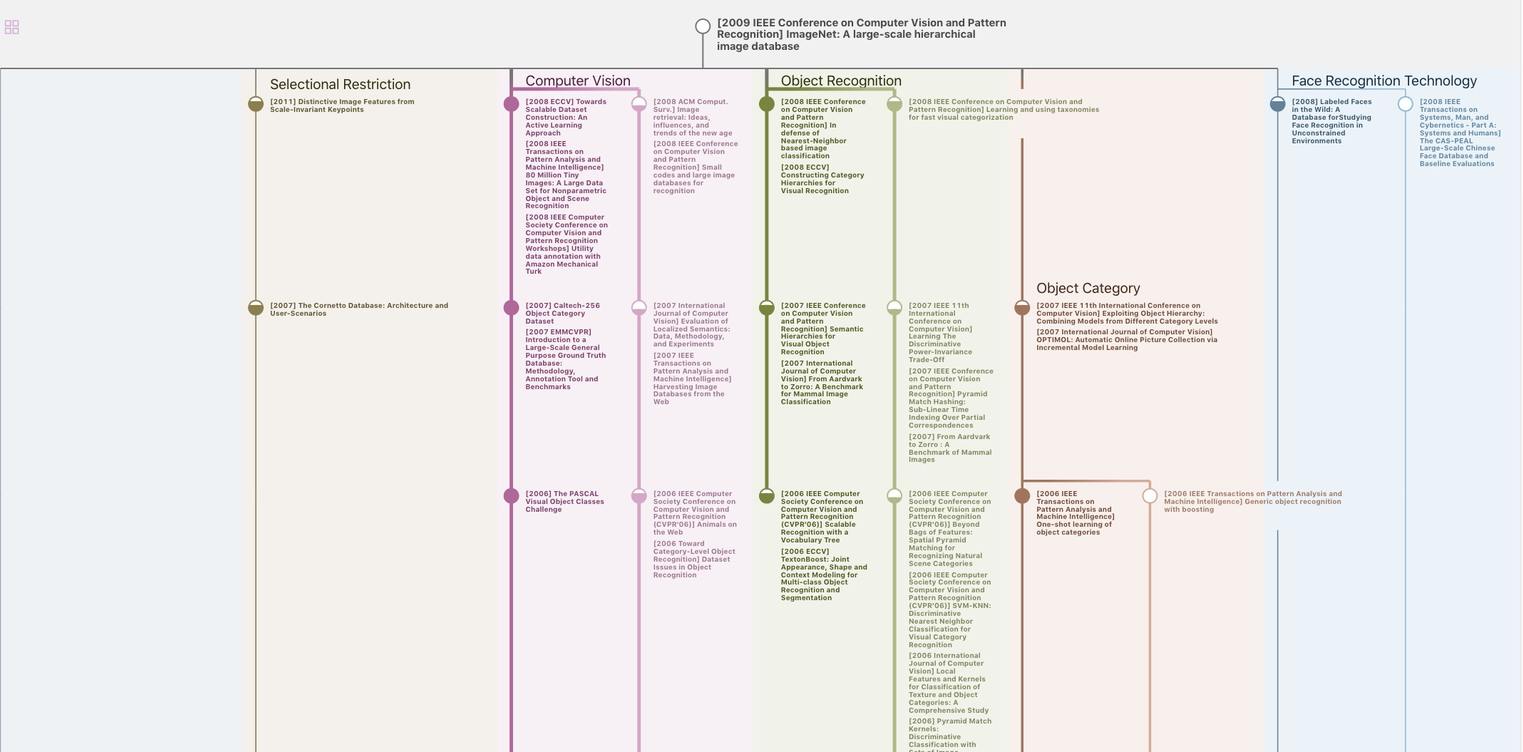
生成溯源树,研究论文发展脉络
Chat Paper
正在生成论文摘要