A Privacy Robust Aggregation Method Based on Federated Learning in the IoT
ELECTRONICS(2023)
摘要
Federated learning has been widely applied because it enables a large number of IoT devices to conduct collaborative training while maintaining private data localization. However, the security risks and threats faced by federated learning in IoT applications are becoming increasingly prominent. Except for direct data leakage, there is also a need to face threats that attackers interpret gradients and infer private information. This paper proposes a Privacy Robust Aggregation Based on Federated Learning (PBA), which can be applied to multiple server scenarios. PBA filters outliers by using the approximate Euclidean distance calculated from binary sequences and the 3s criterion. Then, this paper provides correctness analysis and computational complexity analysis on the aggregation process of PBA. Moreover, the performance of PBA is evaluated concerning ensuring privacy and robustness in this paper. The results indicate that PBA can resist Byzantine attacks and a state-of-the-art privacy inference, which means that PBA can ensure privacy and robustness.
更多查看译文
关键词
privacy robust aggregation method,federated learning,iot
AI 理解论文
溯源树
样例
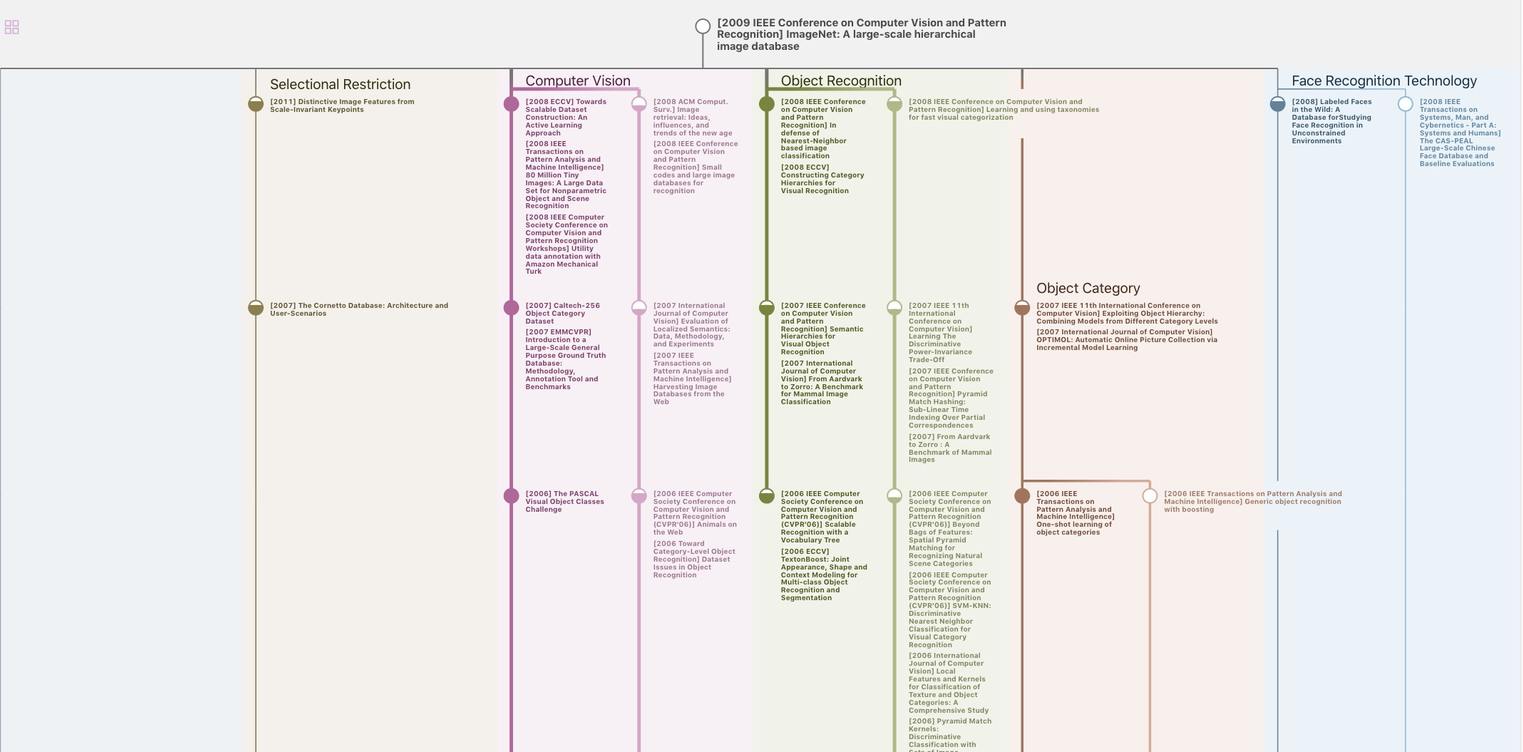
生成溯源树,研究论文发展脉络
Chat Paper
正在生成论文摘要