Smart Interference Signal Design to a Cognitive Radar
2023 IEEE RADAR CONFERENCE, RADARCONF23(2023)
Univ Dayton | Cornell Univ | Informat Syst Labs Inc | Air Force Res Lab
Abstract
This paper addresses an adversarial inference problem involving cognitive radars. The game theoretic framework described in this paper comprises “us” and an “adversary”. Our goal is to design an external interference signal that confuses the adversary radar with given information of the signals of the radar. The optimization problem is formulated such that the signal power of the designed interference is minimized while enforcing the probability that the signal-to-clutter-plus-noise ratio (SCNR) of the radar exceeds a certain SCNR level to be less than a specified threshold. The resulting problem is a challenging optimization problem since the constraint is based on a probability density function (PDF), which is non-differentiable. By taking an expected value of the SCNR, the problem is relaxed to a convex problem using the semidefinite relaxation. The simulation results verify the performance of the designed interference using the high-fidelity modeling and simulation tool, RFView.
MoreTranslated text
Key words
cognitive fully adaptive radar,Green's function,non-convex quadratic constrained quadratic programming,semidefinite relaxation,semidefinite programming
求助PDF
上传PDF
View via Publisher
AI Read Science
AI Summary
AI Summary is the key point extracted automatically understanding the full text of the paper, including the background, methods, results, conclusions, icons and other key content, so that you can get the outline of the paper at a glance.
Example
Background
Key content
Introduction
Methods
Results
Related work
Fund
Key content
- Pretraining has recently greatly promoted the development of natural language processing (NLP)
- We show that M6 outperforms the baselines in multimodal downstream tasks, and the large M6 with 10 parameters can reach a better performance
- We propose a method called M6 that is able to process information of multiple modalities and perform both single-modal and cross-modal understanding and generation
- The model is scaled to large model with 10 billion parameters with sophisticated deployment, and the 10 -parameter M6-large is the largest pretrained model in Chinese
- Experimental results show that our proposed M6 outperforms the baseline in a number of downstream tasks concerning both single modality and multiple modalities We will continue the pretraining of extremely large models by increasing data to explore the limit of its performance
Upload PDF to Generate Summary
Must-Reading Tree
Example
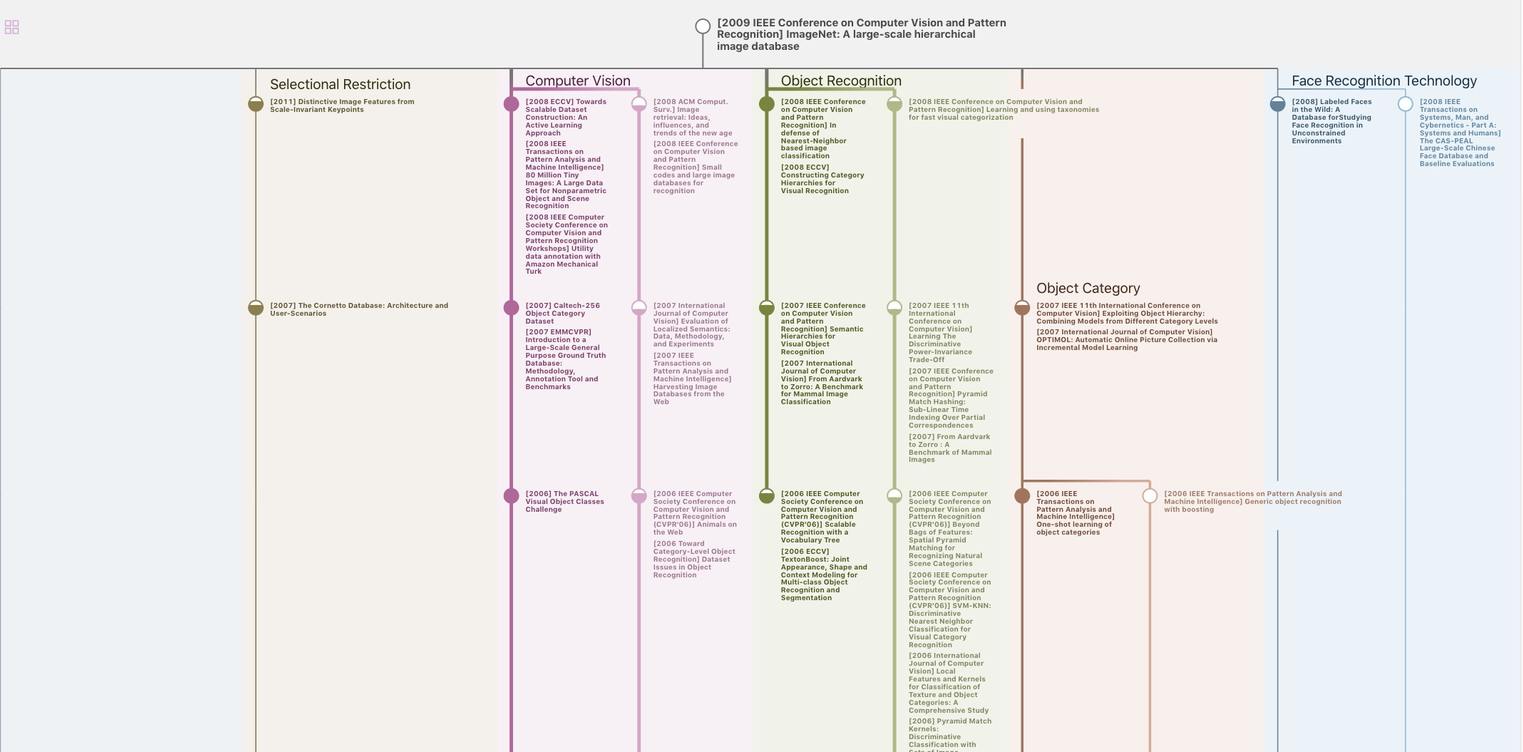
Generate MRT to find the research sequence of this paper
Related Papers
1997
被引用143 | 浏览
2021
被引用11 | 浏览
Data Disclaimer
The page data are from open Internet sources, cooperative publishers and automatic analysis results through AI technology. We do not make any commitments and guarantees for the validity, accuracy, correctness, reliability, completeness and timeliness of the page data. If you have any questions, please contact us by email: report@aminer.cn
Chat Paper
GPU is busy, summary generation fails
Rerequest