Physics-informed machine learning models for the prediction of transient temperature distribution of ferritic steel in directed energy deposition by cold metal transfer
SCIENCE AND TECHNOLOGY OF WELDING AND JOINING(2023)
摘要
In-situ monitoring of the additive layer characteristics in the directed energy deposition (DED) process by any contact technology is cumbersome. A well-tested finite element (FE) model is often employed to extract transient temperature distribution during deposition. However, the numerical model pertaining to each deposition attribute is computationally expensive. In the present work, we have generated a dataset through an experimentally validated thermal model, and further multiple machine learning (ML) algorithms are applied to train datasets. Models with an accuracy of more than 99% are utilised for the prediction of transient temperature distribution. The validation of deposition attributes using experiments and numerical model suggests that the physics-informed machine learning models for cold metal transfer can be applied in the DED process.
更多查看译文
关键词
Finite element model, machine learning algorithms, cold metal transfer, wire arc additive manufacturing, >
AI 理解论文
溯源树
样例
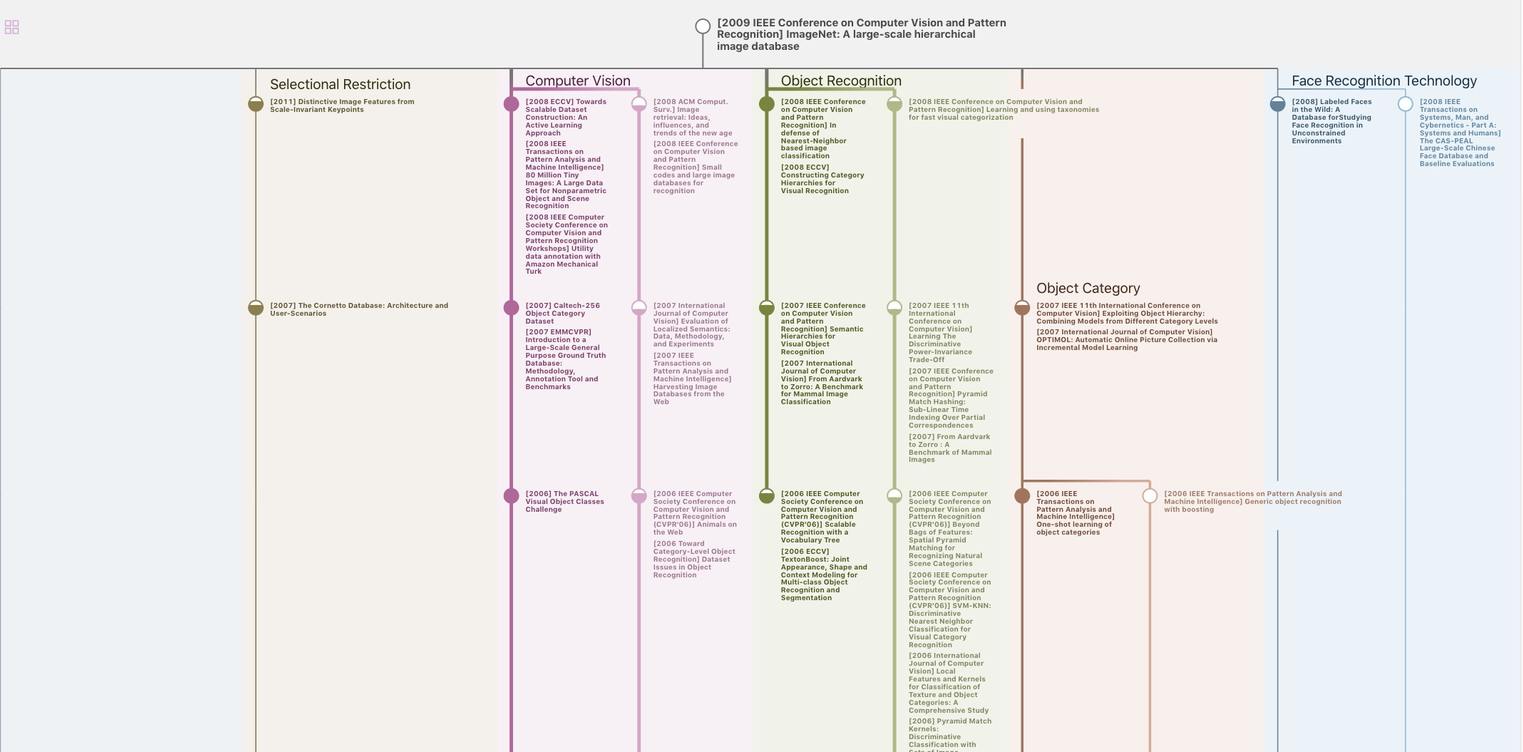
生成溯源树,研究论文发展脉络
Chat Paper
正在生成论文摘要