Dynamic interaction between dislocations and obstacles in bcc iron based on atomic potentials derived using neural networks
PHYSICAL REVIEW MATERIALS(2023)
摘要
The introduction of obstacles (e.g., precipitates) for controlling dislocation motion in molecular structures is a prevalent method for designing the mechanical strength of metals. Owing to the nanoscale size of the dislocation core (<= 1 nm), atomic modeling is required to investigate the interactions between the dislocation and obstacles. However, conventional empirical potentials are not adequately accurate in contrast to calculations based on density functional theory (DFT). Therefore, the atomic-level details of the interactions between the dislocations and obstacles remain unclarified. To this end, in this paper, we applied an artificial neural network (ANN) framework to construct an atomic potential by leveraging the high accuracy of DFT. Using the constructed ANN potential, we investigated the dynamic interaction between the (a(0)/2)< 111 >{110} edge dislocation and obstacles in body-centered cubic (bcc) iron. When the dislocation crossed the void, an ultrasmooth and symmetric half-loop was observed for the bowing-out dislocation. Except for the screw dislocation, the Peierls stress of all the dislocations predicted using the ANN was <100 MPa. More importantly, the results confirmed the formation of an Orowan loop in the interaction between a rigid sphere and dislocation. Furthermore, we discovered a phenomenon in which the Orowan loop disintegrated into two small loops during its interaction with the rigid sphere and dislocation.
更多查看译文
关键词
bcc iron,atomic potentials,dislocations,neural networks
AI 理解论文
溯源树
样例
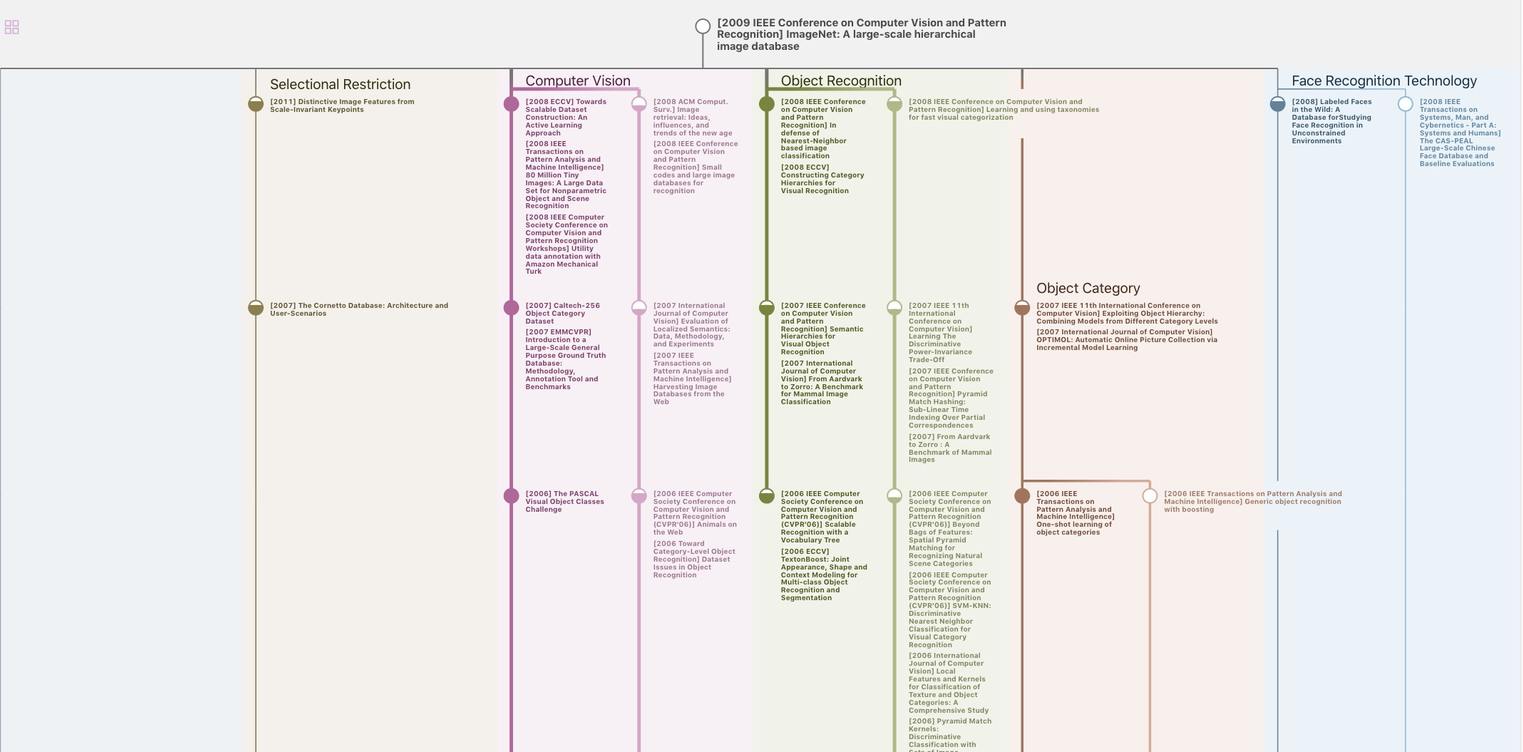
生成溯源树,研究论文发展脉络
Chat Paper
正在生成论文摘要