Multi-Agent Reinforcement Learning With Policy Clipping and Average Evaluation for UAV-Assisted Communication Markov Game
IEEE TRANSACTIONS ON INTELLIGENT TRANSPORTATION SYSTEMS(2023)
摘要
Unmanned aerial vehicle (UAV)-assisted communication is a significant technology in 6G communication. In order to cope with the dynamic trajectory optimization problem of the air-ground network, the interaction between entities is modeled as a Markov game firstly. Then, the model-free multi-agent reinforcement learning (MARL) is adopted to optimize individual decision-making. This enables agents to learn the mobile patterns of others, so as to optimize their own mobile strategy. However, there are some common issues when executing the benchmark MARL algorithms, such as biased estimation and local optimum. To solve these problems, an enhanced multi-agent proximal policy optimization algorithm is proposed with policy clipping and average evaluation to guarantee the fast convergence and accurate estimation. Simulations demonstrate that this method produces superior convergence than the benchmark algorithms. It allows the UAV base station, ground users and the aerial jammer to adopt the optimal mobile strategies to achieve their respective maximum cumulative rewards. In addition, the stable strategies of agents constitute the approximate Nash equilibrium for the UAV-assisted communication Markov Game.
更多查看译文
关键词
Multi-agent reinforcement learning,UAV-assisted communication,game theory
AI 理解论文
溯源树
样例
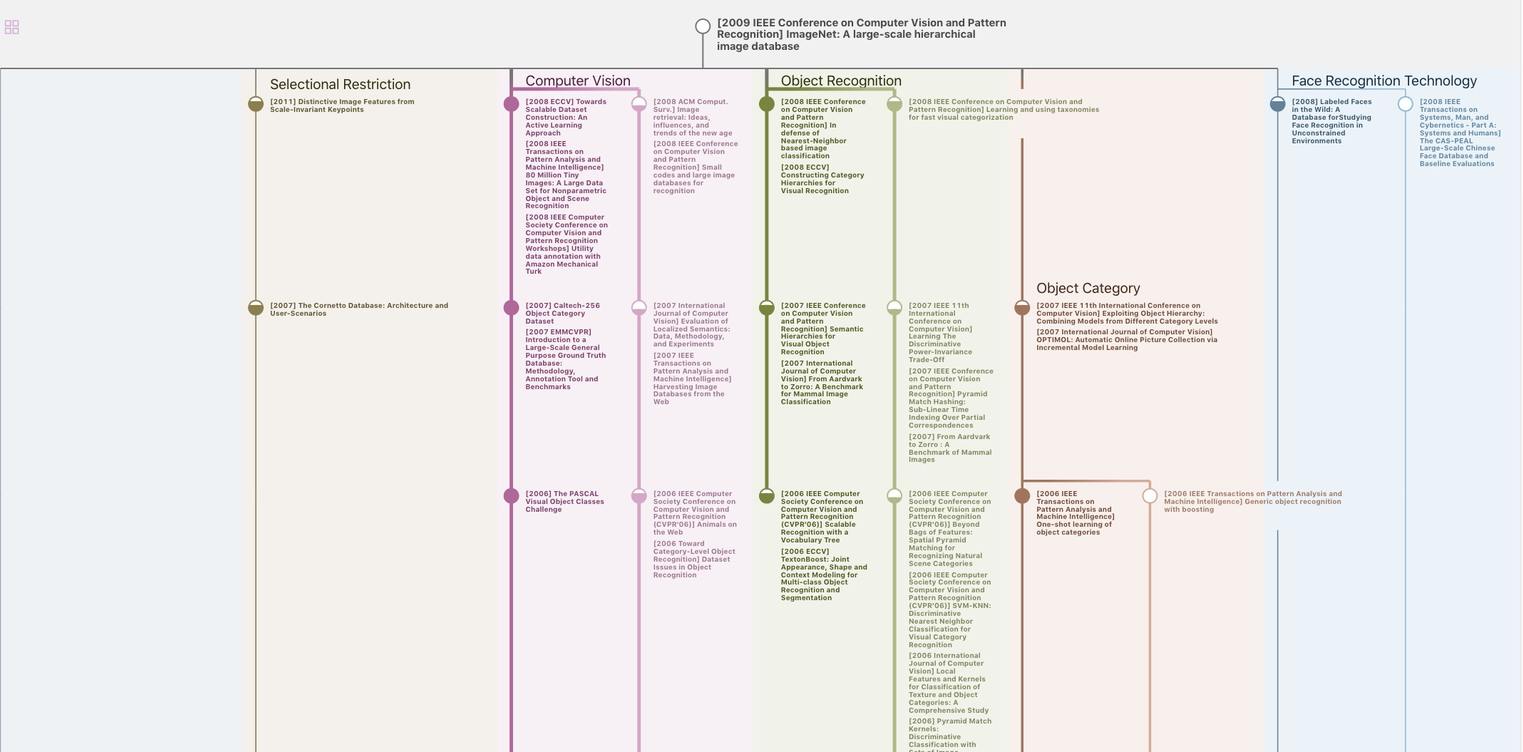
生成溯源树,研究论文发展脉络
Chat Paper
正在生成论文摘要