Reconstructing PM2.5 Data Record for the Kathmandu Valley Using a Machine Learning Model
ATMOSPHERE(2023)
摘要
This paper presents a method for reconstructing the historical hourly concentrations of Particulate Matter 2.5 (PM2.5) over the Kathmandu Valley from 1980 to the present. The method uses a machine learning model that is trained using PM2.5 readings from the US Embassy (Phora Durbar) as a ground truth, and the meteorological data from the Modern-Era Retrospective Analysis for Research and Applications v2 (MERRA2) as input. The Extreme Gradient Boosting (XGBoost) model acquires a credible 10-fold cross-validation (CV) score of similar to 83.4%, an r2-score of similar to 84%, a Root Mean Square Error (RMSE) of similar to 15.82 mu g/m(3), and a Mean Absolute Error (MAE) of similar to 10.27 mu g/m(3). Further demonstrating the model's applicability to years other than those for which truth values are unavailable, the multiple cross-tests with an unseen data set offered r2-scores for 2018, 2019, and 2020 ranging from 56% to 67%. The model-predicted data agree with true values and indicate that MERRA2 underestimates PM2.5 over the region. It strongly agrees with ground-based evidence showing substantially higher mass concentrations in the dry pre- and post-monsoon seasons than in the monsoon months. It also shows a strong anti-correlation between PM2.5 concentration and humidity. The results also demonstrate that none of the years fulfilled the annual mean air quality index (AQI) standards set by the World Health Organization (WHO).
更多查看译文
关键词
machine learning, reconstructed historical PM2.5, Kathmandu Valley
AI 理解论文
溯源树
样例
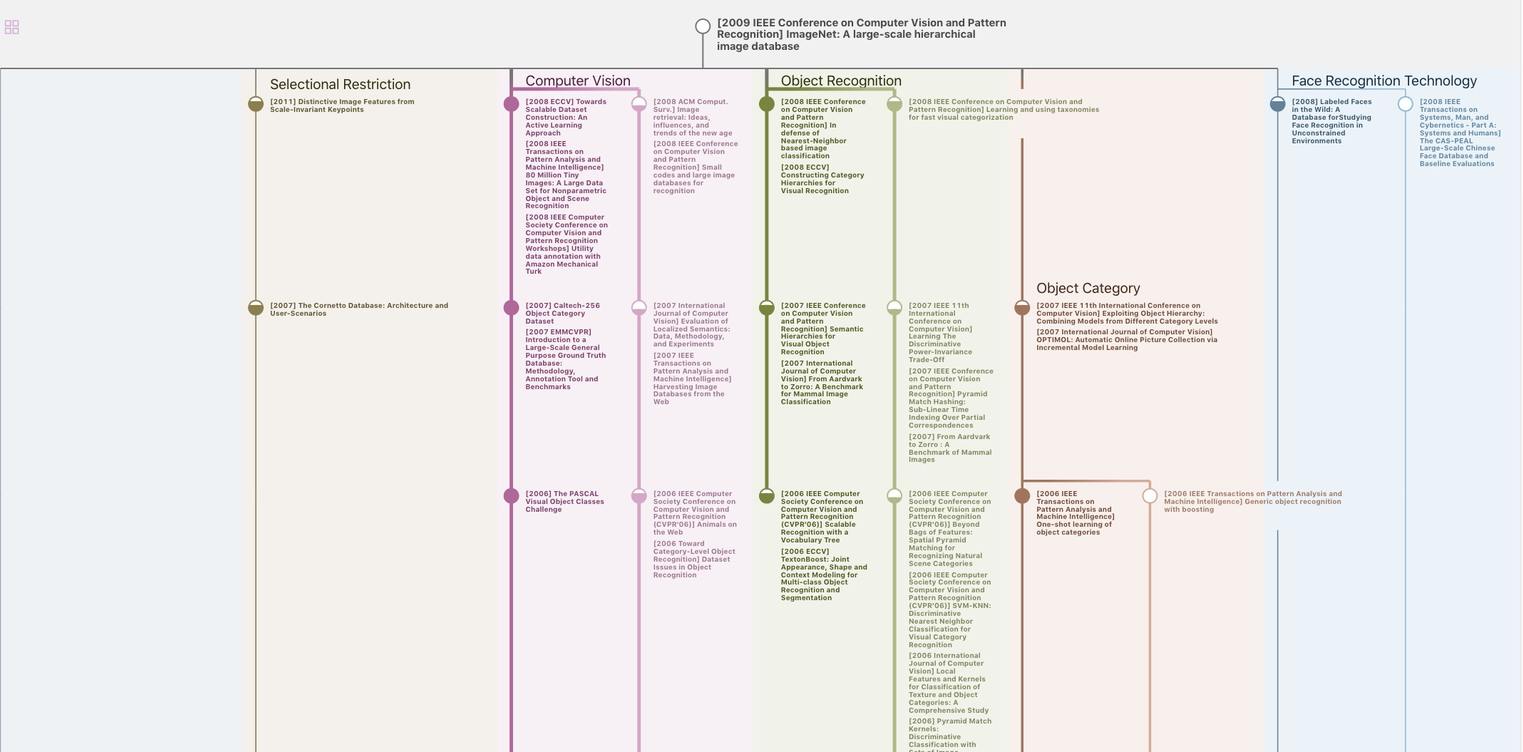
生成溯源树,研究论文发展脉络
Chat Paper
正在生成论文摘要