We Need to Communicate: Communicating Attention Network for Semantic Segmentation of High-Resolution Remote Sensing Images
REMOTE SENSING(2023)
摘要
Traditional models that employ CNNs as encoders do not sufficiently combine high-level features and low-level features. However, high-level features are rich in semantic information but lack spatial detail, while low-level features are the opposite. Therefore, the integrated utilization of multi-level features and the bridging of the gap between them is crucial to promote the accuracy of semantic segmentation. To address this issue, we presented communicating mutual attention (CMA) and communicating self-attention (CSA) modules to enhance the interaction and fusion of different levels of feature maps. On the one hand, CMA aggregates the global context information of high-level features into low-level features and embeds the spatial detail localization characteristics of low-level features in high-level features. On the other hand, the CSA module is deployed to integrate the spatially detailed representation of low-level features into the attention map of high-level features. We have experimented with the communicating attention network (CANet), a U-net-like network composed of multiple CMA and CSA modules, on the ISPRS Vaihingen and Potsdam datasets with mean F1-scores of 89.61% and 92.60%, respectively. The results demonstrate that CANet embodies superior performance in the semantic segmentation task of remote sensing of images.
更多查看译文
关键词
communicating attention network,semantic segmentation,high-resolution high-resolution
AI 理解论文
溯源树
样例
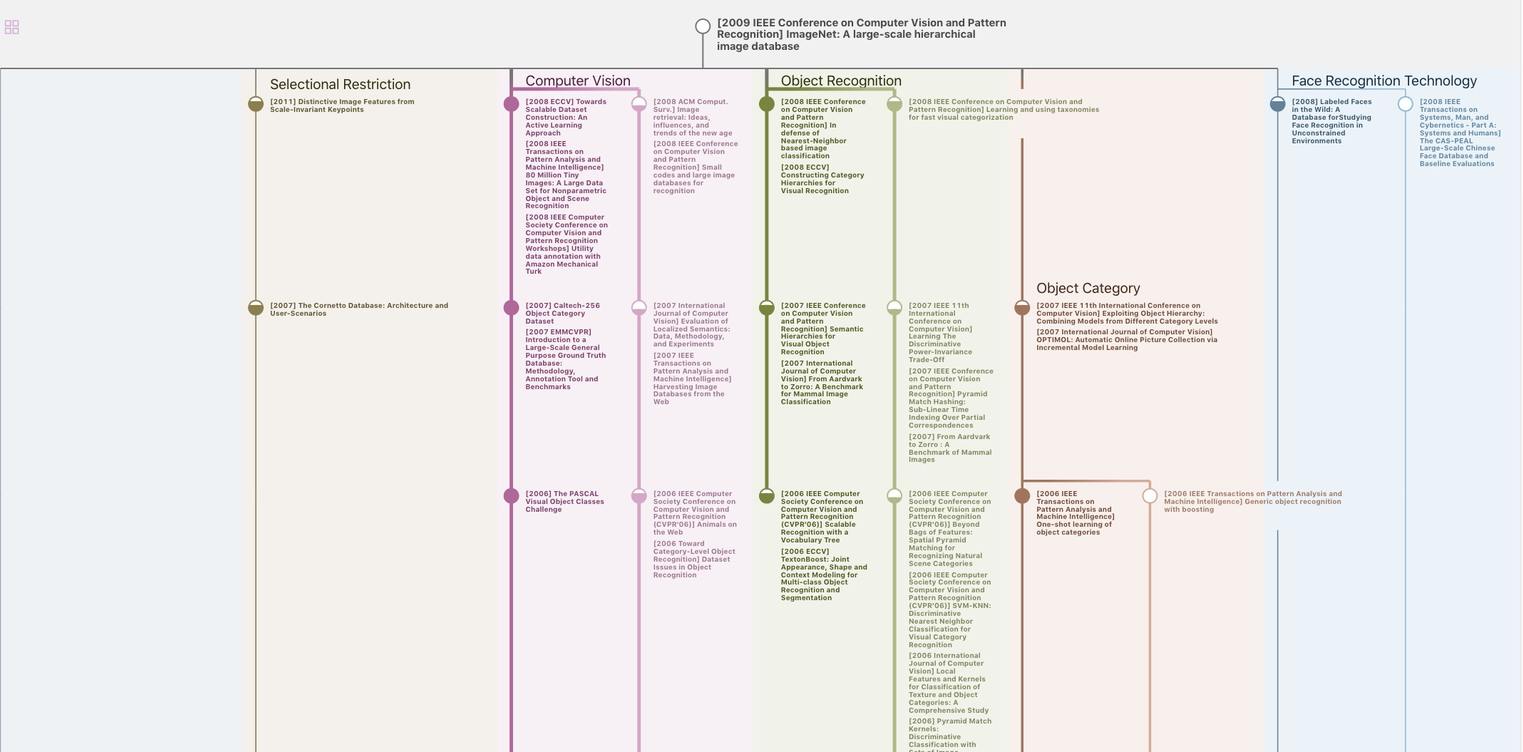
生成溯源树,研究论文发展脉络
Chat Paper
正在生成论文摘要