Accelerating the design and optimization of catalysts for the hydrogen evolution reaction in transition metal phosphides using machine learning
MOLECULAR CATALYSIS(2023)
摘要
Transition metal phosphides (TMPs) are potential substitutes for platinum group metal catalysts in hydrogen evolution reactions (HER) for sustainable energy. In this work, a novel machine learning (ML)-based technique for HER performance prediction of TMPs is presented. AGH, which assesses catalytic activity, was utilized as the expected target value and data from sources like collected papers and self-calculated data were also employed. The random forest (RFR) technique produced an R2 of 0.89 on the test set, while non-tree ML algorithms were utilized for learning and feedback. Additionally, TMPs of AGH were predicted using an original Ensemble learning technique. The ensemble learning method significantly increased the model's predictive effect and strengthened the model's stability and resilience, resulting in an R2 >0.85 on the test set for the tree algorithm model. The SHAP (SHapley Additive exPlanations) algorithm and RFR showed that the geometric means of atomic volume, first ionization energy, and p-orbital electron number significantly impact free energy performance evaluation by tree ML algorithms. These findings can effectively guide the design and development of new products. In conclusion, this approach offers a useful tool to assess TMPs' performance in HER studies.
更多查看译文
关键词
Transition metal phosphides,Machine learning,Hydrogen evolution reaction,Ensemble learning
AI 理解论文
溯源树
样例
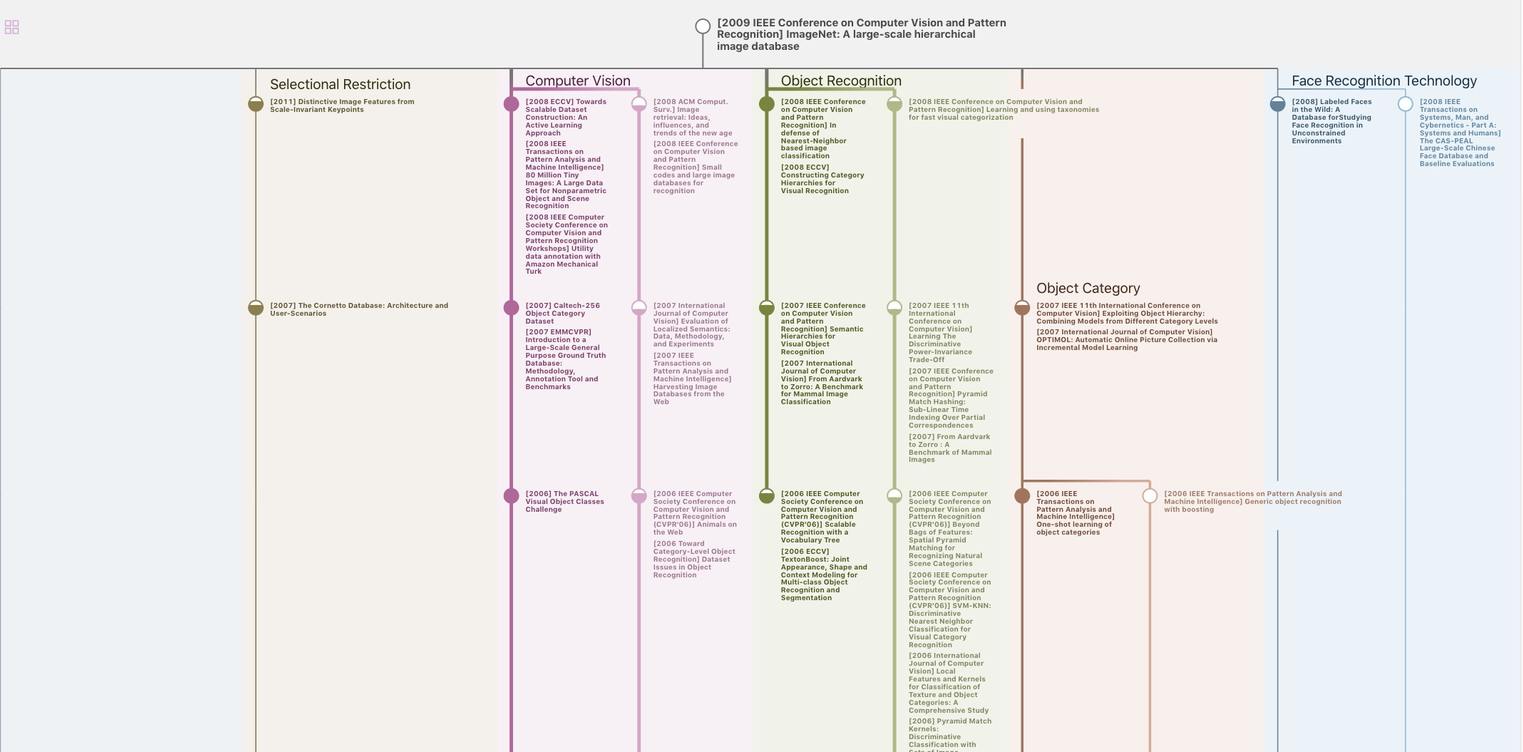
生成溯源树,研究论文发展脉络
Chat Paper
正在生成论文摘要