Sea Surface Chlorophyll-a Concentration Retrieval from HY-1C Satellite Data Based on Residual Network
REMOTE SENSING(2023)
摘要
A residual network (ResNet) model was proposed for estimating Chl-a concentrations in global oceans from the remote sensing reflectance (R-rs) observed by the Chinese ocean color and temperature scanner (COCTS) onboard the HY-1C satellite. A total of 52 images from September 2018 to September 2019 were collected, and the label data were from the multi-task Ocean Color-Climate Change Initiative (OC-CCI) daily products. The results of feature selection and sensitivity experiments show that the logarithmic values of R(rs)565 and R(rs)520/Rrs443, R(rs)565/R(rs)490, R(rs)520/R(rs)490, R(rs)490/R(rs)443, and R(rs)670/R(rs)565 are the optimal input parameters for the model. Compared with the classical empirical OC4 algorithm and other machine learning models, including the artificial neural network (ANN), deep neural network (DNN), and random forest (RF), the ResNet retrievals are in better agreement with the OC-CCI Chl-a products. The root-mean-square error (RMSE), unbiased percentage difference (UPD), and correlation coefficient (logarithmic, R(log)) are 0.13 mg/m(3), 17.31%, and 0.97, respectively. The performance of the ResNet model was also evaluated against in situ measurements from the Aerosol Robotic Network-Ocean Color (AERONET-OC) and field survey observations in the East and South China Seas. Compared with DNN, ANN, RF, and OC4 models, the UPD is reduced by 5.9%, 0.7%, 6.8%, and 6.3%, respectively.
更多查看译文
关键词
sea surface chlorophyll-a concentration (Chl-a),Chinese ocean color and temperature scanner (COCTS),HY-1C,residual neural network
AI 理解论文
溯源树
样例
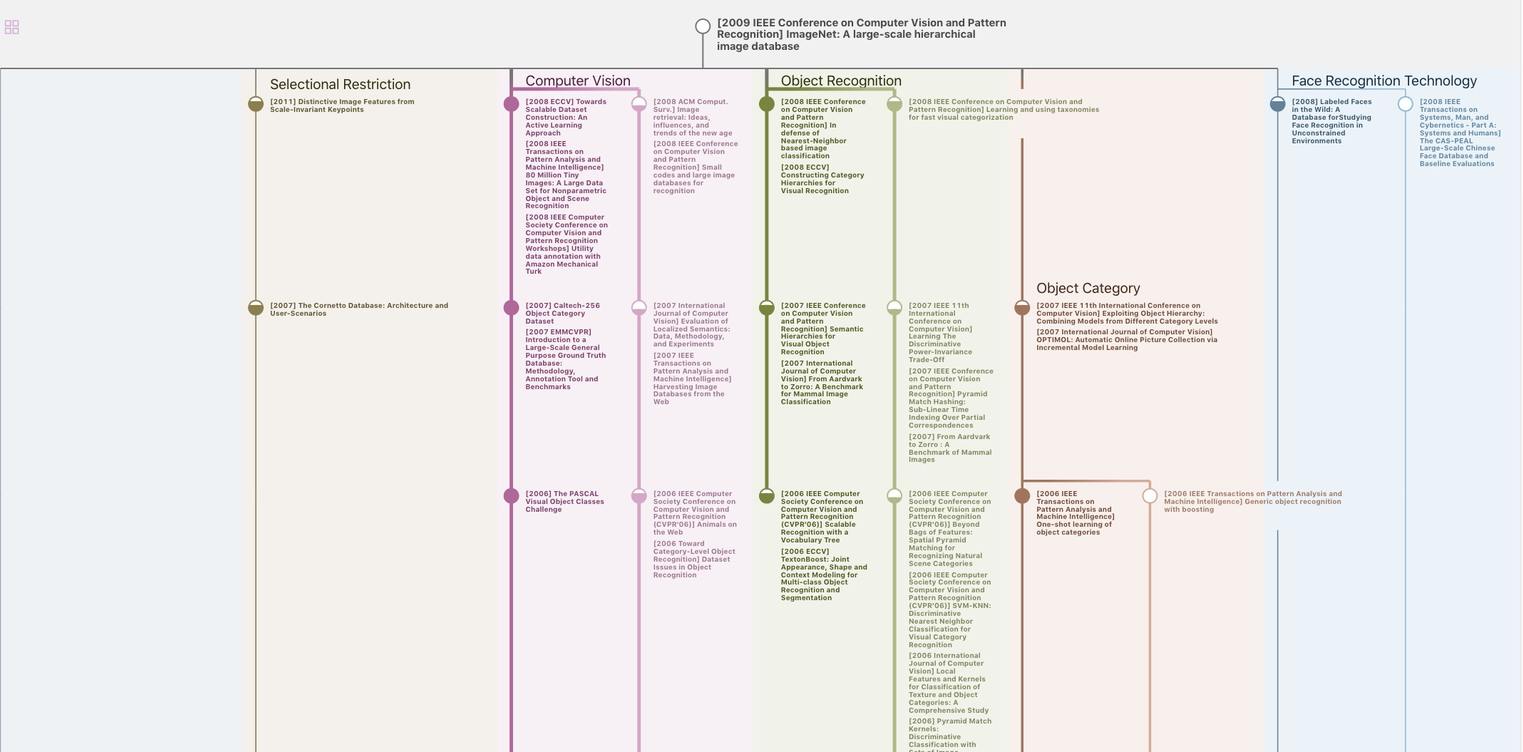
生成溯源树,研究论文发展脉络
Chat Paper
正在生成论文摘要