A crystallization case study toward optimization of expensive to evaluate mathematical models using Bayesian approach
MATERIALS AND MANUFACTURING PROCESSES(2023)
摘要
Crystallization process operated in MSMPR mode is of great relevance in manufacturing because of its low operational requirements and easy maintenance. However, to enhance product purity and downstream operations, one needs to handle trade-off among residence time, mean and spread of crystal size distribution. In this paper, we, therefore, study this multi-objective optimization problem (MOOP) to obtain the Pareto solutions using high-fidelity (HF) population balance equations. We parallely aim to develop an optimal Pareto front using Multi-objective Bayesian Optimization (MOBO) technique which exploits the qEHVI as the acquisition function to identify the optimization path. The results show that MOBO requires only 2.4% of HF functional calls compared to evolutionary approaches to generate similar quality results. This indicates the potential of MOBO in optimizing the time expensive MOOPs with minimum number of function calls, which can be easily extended for response surface-based optimization studies involving design of experiments.
更多查看译文
关键词
Bayesian optimization, crystallization, sampling, machine learning, Pareto
AI 理解论文
溯源树
样例
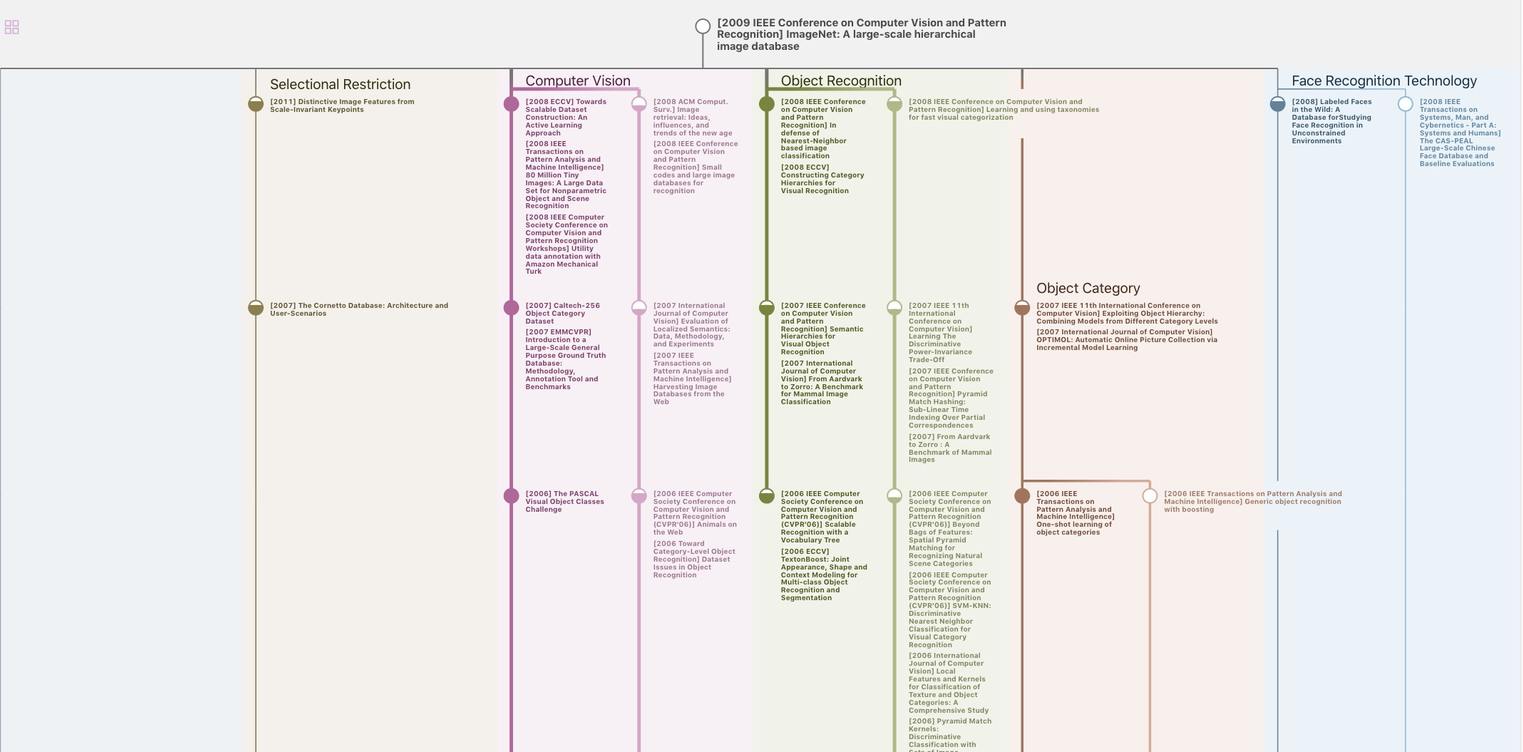
生成溯源树,研究论文发展脉络
Chat Paper
正在生成论文摘要