State-of-health estimation for lithium-ion batteries based on Bi-LSTM-AM and LLE feature extraction
FRONTIERS IN ENERGY RESEARCH(2023)
摘要
With the increasing demands for battery safety management, data-driven method becomes a promising solution for highly accurate battery state of health (SOH) estimation. However, the data-driven method faces problems of poor interpretability and high dependence on input features. This paper proposes a SOH estimation method that integrates data-driven model and signal analysis method. Specifically, the differential thermal voltammetry (DTV) analysis method is used to analyze aging characteristics to obtain features strongly related to battery aging and solve the problem of poor interpretability of data-driven methods. The use of local linear embedding method (LLE) for feature extraction has improved model efficiency. A data-driven model is constructed with the Bi-directional long short-term memory (Bi-LSTM) as the core, and the attention mechanism (AM) is added to focus on important parts of the sequence to further improve the accuracy of the model. The proposed method is validated based on the Oxford battery degradation dataset, and the results show that the proposed method achieves high accuracy and strong robustness in SOH estimation with a root mean square error (RMSE) maintained at about 0.4%. This method has the potential to be employed on cloud platforms or end-cloud collaboration systems for online implementation.
更多查看译文
关键词
state of health, deep learning, attention mechanism, differential thermal voltammetry, locally linear embedding
AI 理解论文
溯源树
样例
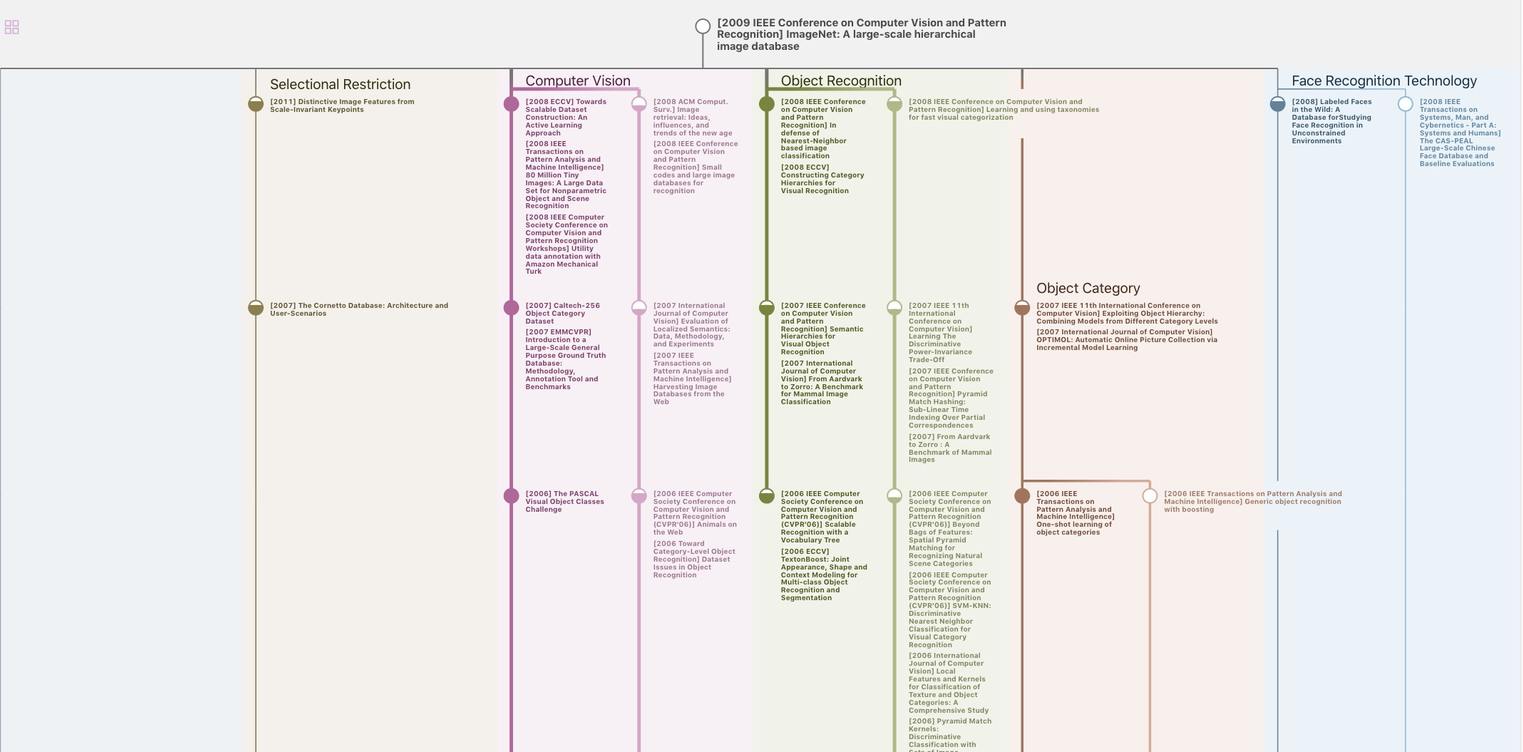
生成溯源树,研究论文发展脉络
Chat Paper
正在生成论文摘要