Machine Learning Estimation of Battery Efficiency and Related Key Performance Indicators in Smart Energy Systems
ENERGIES(2023)
摘要
Battery systems are extensively used in smart energy systems in many different applications, such as Frequency Containment Reserve or Self-Consumption Increase. The behavior of a battery in a particular operation scenario is usually summarized using different key performance indicators (KPIs). Some of these indicators such as efficiency indicate how much of the total electric power supplied to the battery is actually used. Other indicators, such as the number of charging-discharging cycles or the number of charging-discharging swaps, are of relevance for deriving the aging and degradation of a battery system. Obtaining these indicators is very time-demanding: either a set of lab experiments is run, or the battery system is simulated using a battery simulation model. This work instead proposes a machine learning (ML) estimation of battery performance indicators derived from time series input data. For this purpose, a random forest regressor has been trained using the real data of electricity grid frequency evolution, household power demand, and photovoltaic power generation. The results obtained in the research show that the required KPIs can be estimated rapidly with an average relative error of less than 10%. The article demonstrates that the machine learning approach is a suitable alternative to obtain a very fast rough approximation of the expected behavior of a battery system and can be scaled and adapted well for estimation queries of entire fleets of battery systems.
更多查看译文
关键词
battery efficiency,smart energy systems,machine learning,key performance indicators,related key performance indicators
AI 理解论文
溯源树
样例
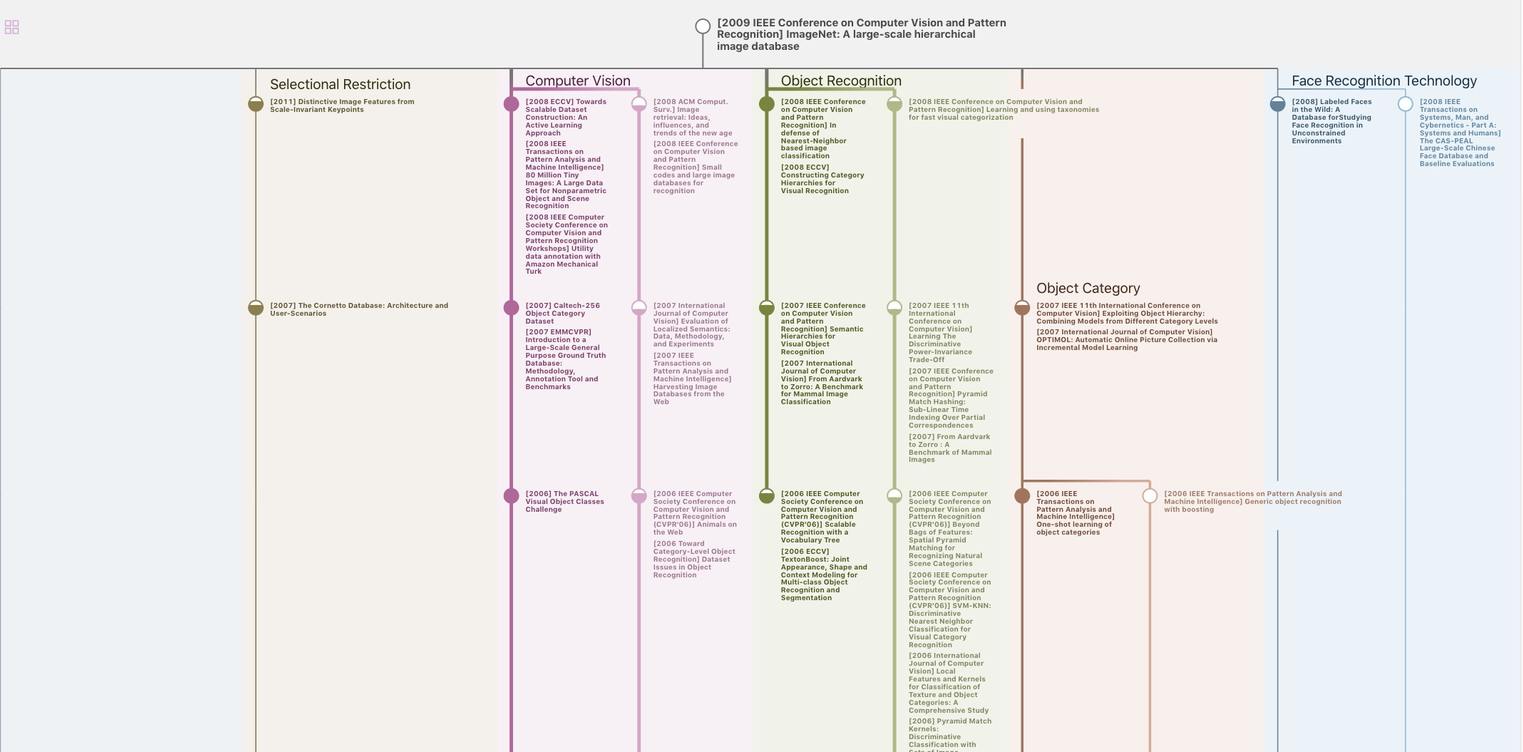
生成溯源树,研究论文发展脉络
Chat Paper
正在生成论文摘要