Deep learning approaches for prediction of adiabatic temperature rise of concrete with complex mixture constituents
JOURNAL OF BUILDING ENGINEERING(2023)
摘要
Temperature control and crack prevention of mass concrete are significant to the safety and durability of structures such as dams and long-span bridges. Adiabatic temperature rise is the key issue in thermal and crack risk analysis of mass concrete. Due to the substantial diversity of admixtures influencing the hydration process in modern concrete, it is challenging to develop a comprehensive analytical model to predict adiabatic temperature rise based on theoretical frameworks. As a data-driven approach, deep learning (DL) provides a potential solution for predicting the properties of complex materials in recent years. DL model based on artificial neuron network (ANN) and recurrent neuron network (RNN) were employed in this study to predict adiabatic temperature rise considering 15 variables as input parameters. The determination coefficient (R2) of the prediction results given from ANN and RNN was 0.7616 and 0.8542, respectively. Segmentation technique and bidirectional deep (BD) neuron network algorithm were implemented to improve the performance of RNN. It was demonstrated that BD-RNN provided better results with promising statistical parameters (R2 = 0.9245) and curve fitting of adiabatic temperature rise profiles. Through optimization and k-fold cross-validation, an evolutionary DL model was developed for reliable prediction of adiabatic temperature rise of concrete with complex admixtures.
更多查看译文
关键词
deep learning,concrete,adiabatic temperature rise,prediction,mixture
AI 理解论文
溯源树
样例
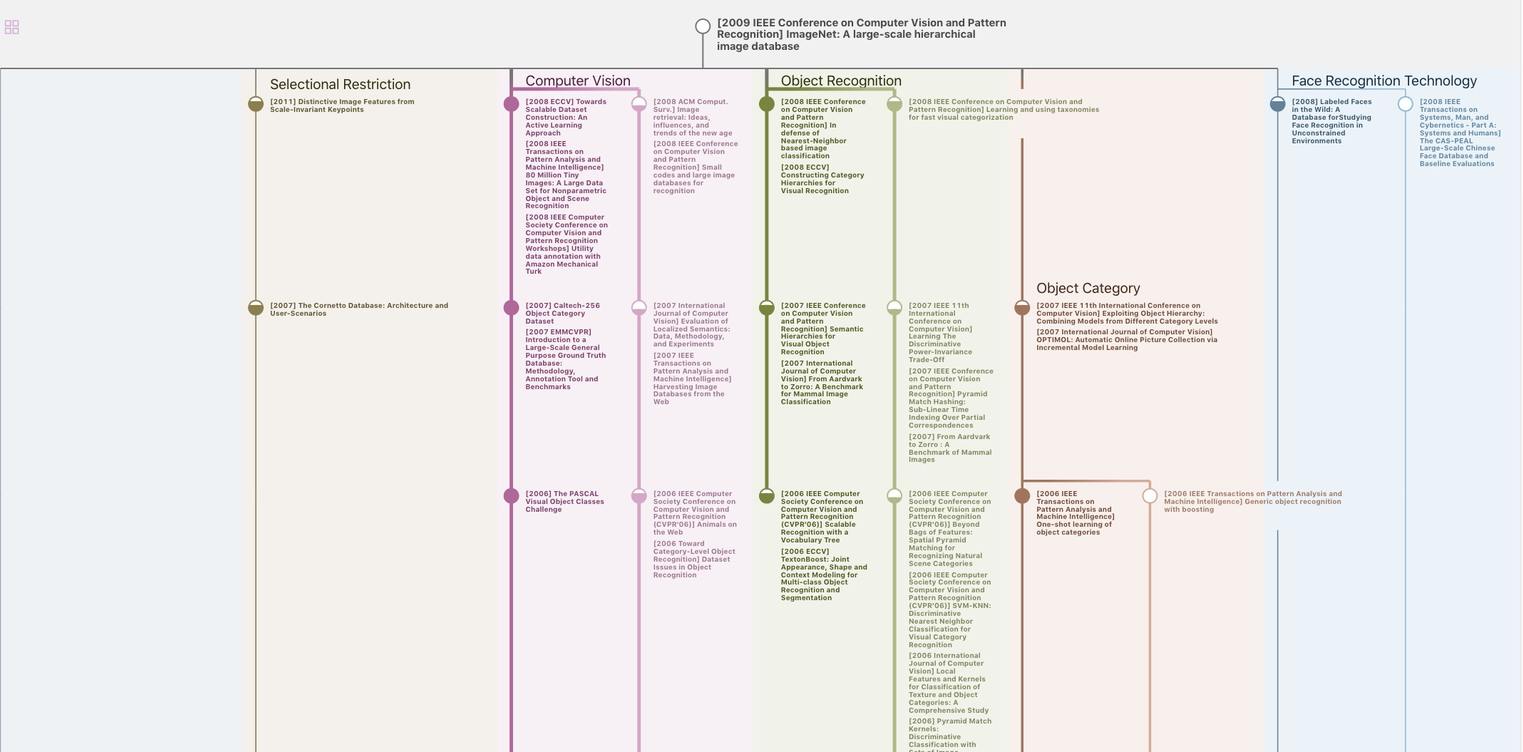
生成溯源树,研究论文发展脉络
Chat Paper
正在生成论文摘要